

Waiting for PostGIS 3.2: ST_InterpolateRaster
source link: https://blog.crunchydata.com/blog/waiting-for-postgis-3.2-st_interpolateraster
Go to the source link to view the article. You can view the picture content, updated content and better typesetting reading experience. If the link is broken, please click the button below to view the snapshot at that time.
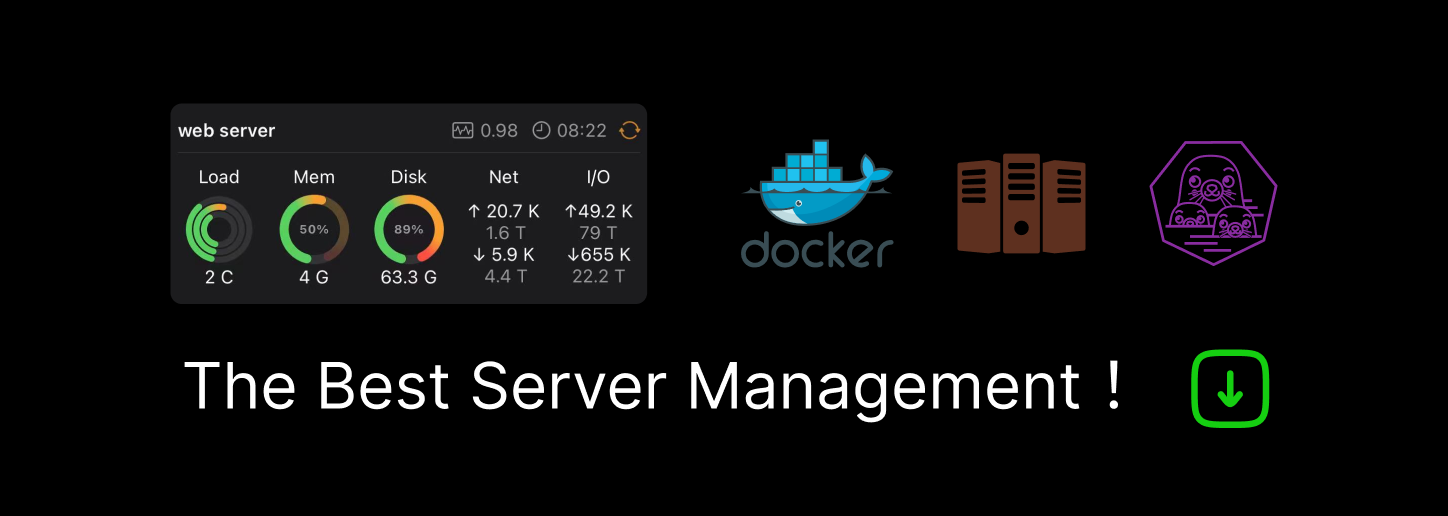
A common situation in the spatial data world is having discrete measurements of a continuous variable. Every place in the world has a temperature, but there are only a finite number of thermometers: how should we reason about places without thermometers and how should we model temperature?
For many use cases, the right way to model a continuous spatial variable is a raster: a regularly spaced grid where each square in the grid contains a value of the variable. This works for temperature and precipitation; it works for elevation and slope; it even works for travel times and wayfinding.
For this blog post, we will build up a temperature surface for Washington State, using the discrete temperature measurements of a set of Department of Transportation (WSDoT) weather stations.
Getting the Data
In conceiving of this post, I envisioned finding a single page with a download of weather stations and associated weather data. Little did I know, few governments assemble that data in a convenient, easy-to-access form.
However, I did find this old page from the WSDoT that has the virtue of being really fast to access and actually containing real-time temperature data.
Behind the map it turns out the temperature data live as HTML overlays.
To turn the web data into a temperature table, I created a Rube Goldberg SQL query, that combines some of my favourite bits and pieces:
- The pgsql-http extension for direct access to HTTP pages inside the database.
- The regexp_matches() function to parse out the pixel coordinates and temperature values from the page HTML.
- Some dirty math to convert the pixel coordinates into spatial coordinates.
- Lots of WITH queries to chain it all together.
The final result looks like this:
CREATE MATERIALIZED VIEW mv_wadot_temp as
-- Read the HTML page from the web server
WITH html AS (
SELECT content FROM http_get('https://wsdot.com/traffic/weather/default.aspx')
),
-- Parse the HTML to extract the data
data AS (
SELECT regexp_matches(
content,
'left: (\d+)px;top: (\d+)px;.*station=(\d+).* title=''(.*?)''.*>(\d+)º', 'gn') as data
FROM html
),
-- Cast the data into appropriate types
rows AS (
select
SELECT[1]::integer AS i,
data[2]::integer AS j,
data[3]::text AS station,
data[4]::text AS title,
data[5]::integer AS degf
FROM data
)
-- Re-scale/translate the pixel coordinates to map coordinates
SELECT ST_SetSRID(ST_MakePoint(
station,
i * 1437 + 184630,
(349 - j) * 1437 - 40500,
degf),3691)::geometry(pointz, 3691) AS geom,
title,
degf FROM rows;
Because it's a materialized view, you can refresh the temperature data at any time by running REFRESH MATERIALIZED VIEW mv_wadot_temp
.
Interpolate a Grid
We can confirm the data are properly located in space by overlaying the points on a standard base map. Not bad!
To turn our points into an full raster surface, we will use the ST_InterpolateRaster() function.
ST_InterpolateRaster() is a binding to the GDAL library, and the functions behind the gdal_grid utility command. To keep things simple, ST_InterpolateRaster() uses the same format for controlling the interpolation algorithm and parameters: "algorithm:param1:value1:param2:value2"
The ST_InterpolateRaster() function needs the following inputs:
- A collection of points, with the value of interest ('degf' in our case) on the Z ordinate.
- A string with the interpolation algorithm and parameters.
- An empty raster (defining the raster location, cell size, width and height) into which to place the interpolation result.
The SQL to generate the raster looks like this:
-- Constants
DROP TABLE IF EXISTS wa_rast;
CREATE TABLE wa_rast AS
WITH inputs AS (
SELECT
500::float8 AS pixelsize,
'invdist:power:5.5:smoothing:2.0' AS algorithm,
ST_Collect(geom) AS geom,
ST_Expand(ST_Collect(geom), 100000) AS ext
FROM mv_wadot_temp
),
-- Calculate output raster geometry
-- Use the expanded extent to take in areas beyond the limit of the
-- temperature stations
sizes AS (
SELECT
ceil((ST_XMax(ext) - ST_XMin(ext))/pixelsize)::integer AS width,
ceil((ST_YMax(ext) - ST_YMin(ext))/pixelsize)::integer AS height,
ST_XMin(ext) AS upperleftx,
ST_YMax(ext) AS upperlefty
FROM inputs
)
-- Feed it all into interpolation
SELECT 1 AS rid,
ST_InterpolateRaster(
geom,
algorithm,
ST_SetSRID(ST_AddBand(ST_MakeEmptyRaster(width, height, upperleftx, upperlefty, pixelsize), '16BSI'), ST_SRID(geom))
) AS rast
FROM sizes, inputs;
The final surface fit provides an interpolated temperature for every point in the raster space!
Temperature models are obviously not as simple as inverse-weighted distance, but this example should show how we can take point measurements and turn them into a continuous surface in SQL.
Conclusions
- You can use HTTP and regexp and glue to bodge together a refreshable data table from a web page.
- Surface interpolation provides a new way to leverage sparse spatial observations into domain-wide estimates.
- With more raster tools (to be discussed in upcoming posts) doing raster/vector calculations will allow for even more interesting data processing.
Recommend
About Joyk
Aggregate valuable and interesting links.
Joyk means Joy of geeK