

Forest-based Classification and Regression (Spatial Statistics)
source link: https://pro.arcgis.com/en/pro-app/latest/tool-reference/spatial-statistics/forestbasedclassificationregression.htm
Go to the source link to view the article. You can view the picture content, updated content and better typesetting reading experience. If the link is broken, please click the button below to view the snapshot at that time.
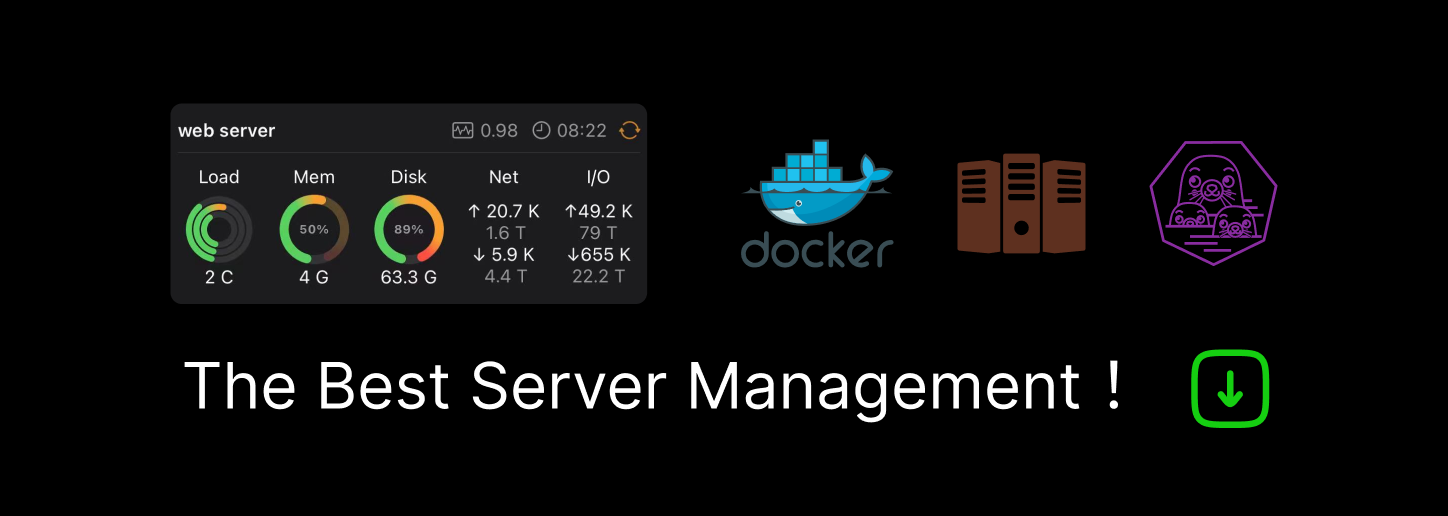
Illustration
Usage
This tool creates hundreds of trees, called an ensemble of decision trees, to create a model that can then be used for prediction. Each decision tree is created using randomly generated portions of the original (training) data. Each tree generates its own prediction and votes on an outcome. The forest model considers votes from all decision trees to predict or classify the outcome of an unknown sample. This is important as individual trees may have issues with overfitting a model; however, combining multiple trees in a forest for prediction addresses the overfitting problem associated with a single tree.
This tool can be used in three different operation modes. The Train option can be used to evaluate the performance of different models as you explore different explanatory variables and tool settings. Once a good model has been found, you can use the Predict to features or Predict to raster option. This is a data-driven tool and performs best on large datasets. The tool should be trained on at least several hundred features for best results. It is not an appropriate tool for very small datasets.
The Input Training Features can be points or polygons. This tool does not work with multipart data.
A Spatial Analyst license is required to use rasters as explanatory variables or to predict to an Output Prediction Surface.
This tool produces a variety of different outputs. Output Trained Features will contain all of the Input Training Features used in the model created as well as all of the explanatory variables used in the model (including the input fields used, any distances calculated, and any raster values extracted or calculated). It will also contain predictions for all of the features used for training the model, which can be helpful in assessing the performance of the model created. When using this tool for prediction, it will produce either a new feature class containing the Output Predicted Features or a new Output Prediction Surface if explanatory rasters are provided.
When using the Predict to features option, a new feature class containing the Output Predicted Features will be created. When the Predict to Raster option, a new Output Prediction Surface will be created.
This tool also creates messages and charts to help you understand the performance of the model created. You can access the messages by hovering over the progress bar, clicking the pop-out button, or expanding the messages section in the Geoprocessing pane. You can also access the messages for a previous run of the Forest-based Classification and Prediction tool via the Geoprocessing history. The messages include information on the model characteristics, out of bag errors, variable importance, and validation diagnostics.
You can use the Output Variable Importance Table parameter to create a table to display a chart of variable importance for evaluation. The top 20 variable importance values are also reported in the messages window. The chart can be accessed directly below the layer in the Contents pane.
Explanatory variables can come from fields or be calculated from distance features or extracted from rasters. You can use any combination of these explanatory variable types, but at least one type is required. The explanatory variables (from fields, distance features, or rasters) used should contain a variety of values. If the explanatory variable is categorical, the Categorical check box should be checked (variables of type string will automatically be checked). Categorical explanatory variables are limited to 60 unique values, though a smaller number of categories will improve model performance. For a given data size, the more categories a variable contains, the more likely it is that it will dominate the model and lead to less effective prediction results.
Distance features are used to automatically create explanatory variables representing a distance from the provided features to the Input Training Features. Distances will be calculated from each of the input Explanatory Training Distance Features to the nearest Input Training Feature. If the input Explanatory Training Distance Features are polygons or lines, the distance attributes are calculated as the distance between the closest segments of the pair of features. However, distances are calculated differently for polygons and lines. See How proximity tools calculate distance for details.
If your Input Training Features are points and you are using Explanatory Training Rasters, the tool drills down to extract explanatory variables at each point location. For multiband rasters, only the first band is used.
Although you can have multiple layers with the same name in the Contents pane, the tool is unable to accept explanatory layers with the same name or to remove duplicate layer names in the drop-down lists. To avoid this issue, ensure that each layer has a unique name.
If your Input Training Features are polygons, the Variable to Predict is categorical, and you are using exclusively Explanatory Training Rasters, there is an option to Convert Polygons to Raster Resolution for Training. If this option is checked, the polygon is divided into points at the centroid of each raster cell whose centroid falls within the polygon. The raster values at each point location are then extracted and used to train the model. A bilinear sampling method is used for numeric variables, and the nearest method is used for categorical variables. The default cell size of the converted polygons will be the maximum cell size of input rasters. However, this can be changed using the Cell Size environment setting. If not checked, one raster value for each polygon will be used in the model. Each polygon is assigned the average value for continuous rasters and the majority for categorical rasters.
Polygons are converted to raster resolution (left) or assigned an average value (right)
There must be variation in the data used for each explanatory variable specified. If you receive an error that there is no variation in one of the fields or rasters specified, you can try running the tool again marking that variable as categorical. If 95 percent of the features have the same value for a particular variable, that variable is flagged as having no variation.
The Compensate for Sparse Categories parameter can be used if the variation in your categories are unbalanced. For instance, if you have some categories that occur hundreds of times in your dataset and a few that occur significantly less often, checking this parameter will ensure that each category is represented in each tree to create balanced models.
When matching explanatory variables, the Prediction and Training fields must be of the same type (a double field in Training must be matched to a double field in Prediction).
Forest-based models do not extrapolate, they can only classify or predict to a value that the model was trained on. When predicting a value based on explanatory variables much higher or lower than the range of the original training dataset, the model will estimate the value to be around the highest or lowest value in the original dataset. This tool may perform poorly when trying to predict with explanatory variables that are out of range of the explanatory variables used to train the model.
The tool will fail if categories exist in the prediction explanatory variables that are not present in the training features.
To use mosaic datasets as explanatory variables, use the Make Mosaic Layer tool first and copy the full path to the layer into the tool or use the Make Mosaic Layer tool and the Make Raster Layer tool to adjust the processing template for the mosaic dataset.
The default value for the Number of Trees parameter is 100. Increasing the number of trees in the forest model will result in more accurate model prediction, but the model will take longer to calculate.
When the Calculate Uncertainty parameter is checked, the tool will calculate a 90 percent prediction interval around each predicted value of the Variable to Predict. When Prediction Type is Train only or Predict to features, two fields are added to either Output Trained Features or Output Predicted Features. These fields, ending with _P05 and _P95, represent the upper and lower bounds of the prediction interval. For any new observation, you can predict with 90 percent confidence that the value of a new observation will fall within the interval, given the same explanatory variables. When the Predict to raster option is used, two additional rasters representing the upper and lower bounds of the prediction interval are added to the Contents pane.
This tool supports parallel processing for prediction and uses 50 percent of available processors by default. The number of processors can be increased or decreased using the Parallel Processing Factor environment.
To learn more about how this tool works and understand the output messages and charts, see How Forest-based Classification and Regression works.
References:
Breiman, Leo. Out-Of-Bag Estimation. 1996.
Breiman, L. (1996). Bagging predictors. Machine learning, 24(2), 123-140.
Breiman, Leo. "Random Forests". Machine Learning. 45 (1): 5-32. doi:10.1023/A:1010933404324. 2001.
Breiman, L., J.H. Friedman, R.A. Olshen, C.J. Stone. Classification and regression trees. New York: Routledge. Chapter 4. 2017.
Dietterich, T. G. (2000, June). Ensemble methods in machine learning. In International workshop on multiple classifier systems (pp. 1-15). Springer, Berlin, Heidelberg.
Gini, C. (1912). Variabilità e mutabilità. Reprinted in Memorie di metodologica statistica (Ed. Pizetti E, Salvemini, T). Rome: Libreria Eredi Virgilio Veschi.
Grömping, U. (2009). Variable importance assessment in regression: linear regression versus random forest. The American Statistician, 63(4), 308-319.
Ho, T. K. (1995, August). Random decision forests. In Document analysis and recognition, 1995., proceedings of the third international conference on Document Analysis and Recognition. (Vol. 1, pp. 278-282). IEEE.
James, G., Witten, D., Hastie, T., & Tibshirani, R. (2013). An introduction to statistical learning (Vol. 112). New York: springer.
LeBlanc, M., & Tibshirani, R. (1996). Combining estimates in regression and classification. Journal of the American Statistical Association, 91(436), 1641-1650.
Loh, W. Y., & Shih, Y. S. (1997). Split selection methods for classification trees. Statistica sinica, 815-840.
Meinshausen, Nicolai. "Quantile regression forests." Journal of Machine Learning Research 7. Jun (2006): 983-999.
Nadeau, C., & Bengio, Y. (2000). Inference for the generalization error. In Advances in neural information processing systems (pp. 307-313).
Strobl, C., Boulesteix, A. L., Kneib, T., Augustin, T., & Zeileis, A. (2008). Conditional variable importance for random forests. BMC bioinformatics, 9(1), 307.
Zhou, Z. H. (2012). Ensemble methods: foundations and algorithms. CRC press.
Recommend
About Joyk
Aggregate valuable and interesting links.
Joyk means Joy of geeK