

Learn to Rank 入门
source link: https://arminli.com/learn-to-rank/
Go to the source link to view the article. You can view the picture content, updated content and better typesetting reading experience. If the link is broken, please click the button below to view the snapshot at that time.
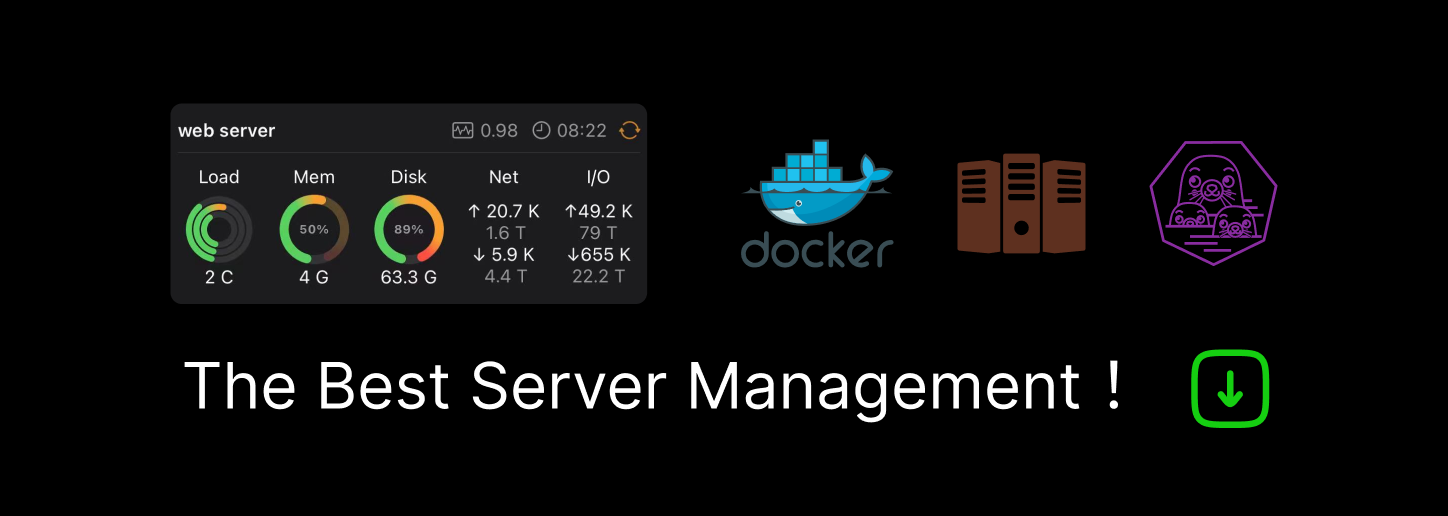
Learn to Rank 入门
March 29, 2017
Learn to Rank(LTR)是使用机器学习技术解决排序问题的方法。
排序是信息检索(IR)的核心问题,比如文件检索,协同过滤,关键词提取,情感分析等,本文以文件检索为例进行讲解。
文件检索并不是一个狭小的领域,其实,网页、邮件、论文、书和新闻都属于文件检索中的例子。
机器学习框架组件
在许多机器学习的研究中,需要注意以下几个关键组件(key components):
输入空间(input space)
包含了我们要研究的对象:通常,我们通过提取出的特征向量表达某个对象。
输出空间(output space)
包含了与输入对象相关的学习目标:在机器学习中,对于输出空间有两种相关但不同的定义。
- 极度依赖应用的任务输出空间:例如,在回归问题中,输出空间是实数 R\mathbb{R}R;在分类问题中,输出空间是离散类别 {0,1,…,k−1}\left\{ 0,1,\ldots ,k-1\right\}{0,1,…,k−1} 。
- 帮助学习过程的输出空间:这与任务的输出空间不同,例如,使用回归算法解决分类问题时,这种情况下有助于学习过程的输出空间是实数,而不是离散的类别。
假设空间(hypothesis space)
定义了从输入空间到输出空间的映射类别:这些函数处理输入对象的特征向量,然后根据输出空间的格式预测。
损失函数(loss function)
LTR 方法
根据上述四个组件,可以把 LTR 的方法分为三类:
- The pointwise approach
- The pairwise approach
- The listwise approach
不同的方法定义了不同的输入和输出空间,使用了不同的假设和不同的损失函数。
The pointwise approach
每个文档的特征向量
query 与每个文档的相关度
包括了使用文档的特征向量作为输入,预测相关度的函数,基于这个函数,我们可以排序所有文档。
不同的 pointwise 算法中,排序被建模为回归、分类、有序回归问题,因此对应的损失函数也有所不同。
需要注意的是:
- pointwise 不考虑文档和文档之间的内在依赖关系,因此在损失函数中,最终的排序列表中每个文件的位置是不可视的。
- pointwise 没有使用不同文档都对同一 query 有关联这一特性。
因此一般来说,pointwise 有一定的局限性。
The pairwise approach
被表示为特征向量的文件对(pair of documents)。
每对文档的 pairwise 偏好值(1 或 -1)。比如一个 pair 是(文件 A,文件 B),那么 A 的 label(rank) 大于 B 的 label,可以视为 +1,反之 -1。
以每对文件(两个变量)作为输入,输出他们的相对顺序的函数 h(可能是排序算法或评分函数)。
损失函数度量了 h 和 label 的差异。
The listwise approach
与 query 整个文件组(group of documents)
- 对于一个 query,所有文件与其的相关度。
- 所有文件排好序的列表(或排列)。
操作所有文件的包含多个变量的函数 h,预测他们的相关度或排列。
与输出空间对应,有两种损失函数。
- label 是 y 时,损失函数通常基于广泛使用的信息检索评估方法或近似值的偏差。
- label 是排好序的序列时,损失函数度量输出的排序后的列表和样本 label。
listwise 的优点是,对于同一 query,损失函数能天然考虑到文件的在列表中的位置。
经过实验,以 LETOR 作为 benchmark 数据集,listwise 表现的效果最好。
Reference
Liu, Tie-Yan. “Learning to rank for information retrieval.” Foundations and Trends® in Information Retrieval 3.3 (2009): 225-331.
Recommend
-
103
Last week we looked at how traditional whole-document parsers struggle to parse big files. Such parsers both too use much memory and...
-
78
-
34
Machine Learning Learning to Rank Explained Learn how the machine learning techniqu...
-
33
Machine Learning ,
-
23
Rank your things… An AES story Automated essay scoring (AES) is the use of specialized computer programs to assign grades to essays written in an educational setting. Its objective is to classify a large set of t...
-
16
Rank N Types in Scala 3 Rank N Types in Scala 3 Table of Contents I'm recently migrating some libs and projects to Scala 3, I guess it would be very helpful to me or anyone interested to learn some new functiona...
-
11
Although it’s been used by SEOs in their keyword research processes for more than a decade now, Keyword Difficulty remains novelty as its formula became ever more sophisticated, just as the search results have.In this article, I’ll go...
-
6
SIAM J. Matrix Anal. Appl., 15(2), 592–622. (31 pages) On Rank-Revealing Factorisations
-
13
When your "rank" is NaN There is programming, and there are programmers. Imagine this in another field. Right now, I can go grab my art supplies, pour out some acrylic paint, dip a brush in it, and start applying it to a...
-
5
Now that RSAs (responsive search ads) have replaced ETAs (expanded text ads) in Google Ads, it may be time to rethink your strategies for optimizing ads. Optimizing RSAs takes a whole different approach than what most advertisers have been...
About Joyk
Aggregate valuable and interesting links.
Joyk means Joy of geeK