

[2007.14321] Label-Only Membership Inference Attacks
source link: https://arxiv.org/abs/2007.14321
Go to the source link to view the article. You can view the picture content, updated content and better typesetting reading experience. If the link is broken, please click the button below to view the snapshot at that time.
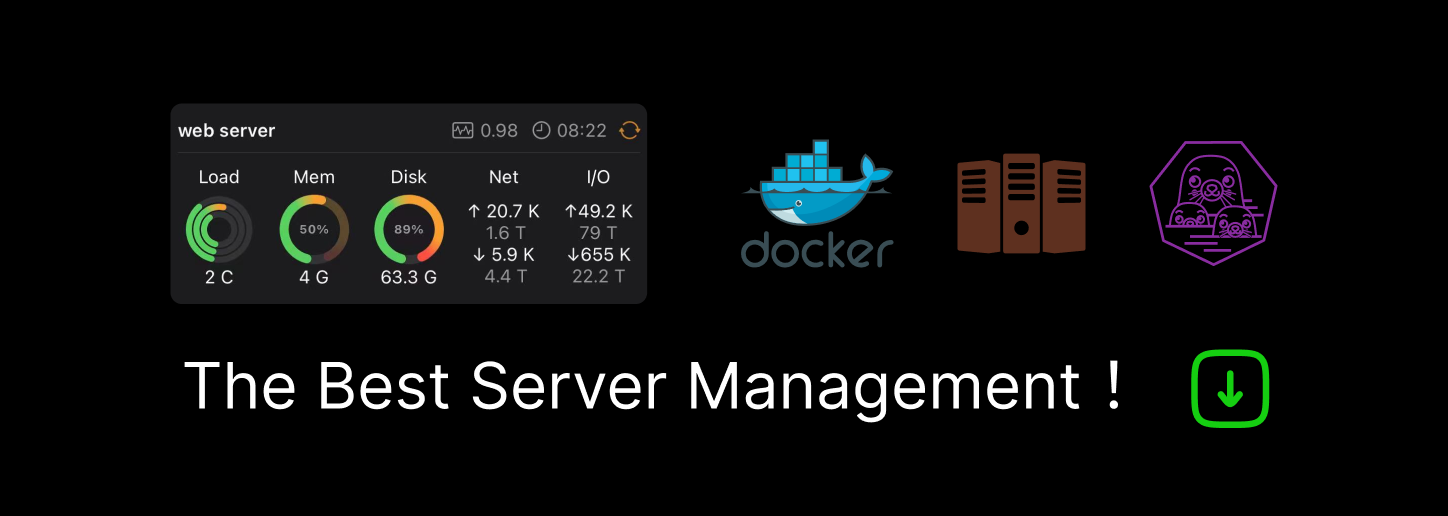
Computer Science > Cryptography and Security
[Submitted on 28 Jul 2020 (v1), last revised 22 Jan 2021 (this version, v2)]
Label-Only Membership Inference Attacks
Membership inference attacks are one of the simplest forms of privacy leakage for machine learning models: given a data point and model, determine whether the point was used to train the model. Existing membership inference attacks exploit models' abnormal confidence when queried on their training data. These attacks do not apply if the adversary only gets access to models' predicted labels, without a confidence measure. In this paper, we introduce label-only membership inference attacks. Instead of relying on confidence scores, our attacks evaluate the robustness of a model's predicted labels under perturbations to obtain a fine-grained membership signal. These perturbations include common data augmentations or adversarial examples. We empirically show that our label-only membership inference attacks perform on par with prior attacks that required access to model confidences. We further demonstrate that label-only attacks break multiple defenses against membership inference attacks that (implicitly or explicitly) rely on a phenomenon we call confidence masking. These defenses modify a model's confidence scores in order to thwart attacks, but leave the model's predicted labels unchanged. Our label-only attacks demonstrate that confidence-masking is not a viable defense strategy against membership inference. Finally, we investigate worst-case label-only attacks, that infer membership for a small number of outlier data points. We show that label-only attacks also match confidence-based attacks in this setting. We find that training models with differential privacy and (strong) L2 regularization are the only known defense strategies that successfully prevents all attacks. This remains true even when the differential privacy budget is too high to offer meaningful provable guarantees.
Submission history
From: Christopher A. Choquette-Choo [view email][v1] Tue, 28 Jul 2020 15:44:31 UTC (652 KB)
[v2] Fri, 22 Jan 2021 01:42:20 UTC (677 KB)
Recommend
About Joyk
Aggregate valuable and interesting links.
Joyk means Joy of geeK