

A Case Study Using Regression-Based Driver Analysis | XM Institute
source link: https://www.xminstitute.com/blog/regression-driver-analysis-retail/
Go to the source link to view the article. You can view the picture content, updated content and better typesetting reading experience. If the link is broken, please click the button below to view the snapshot at that time.
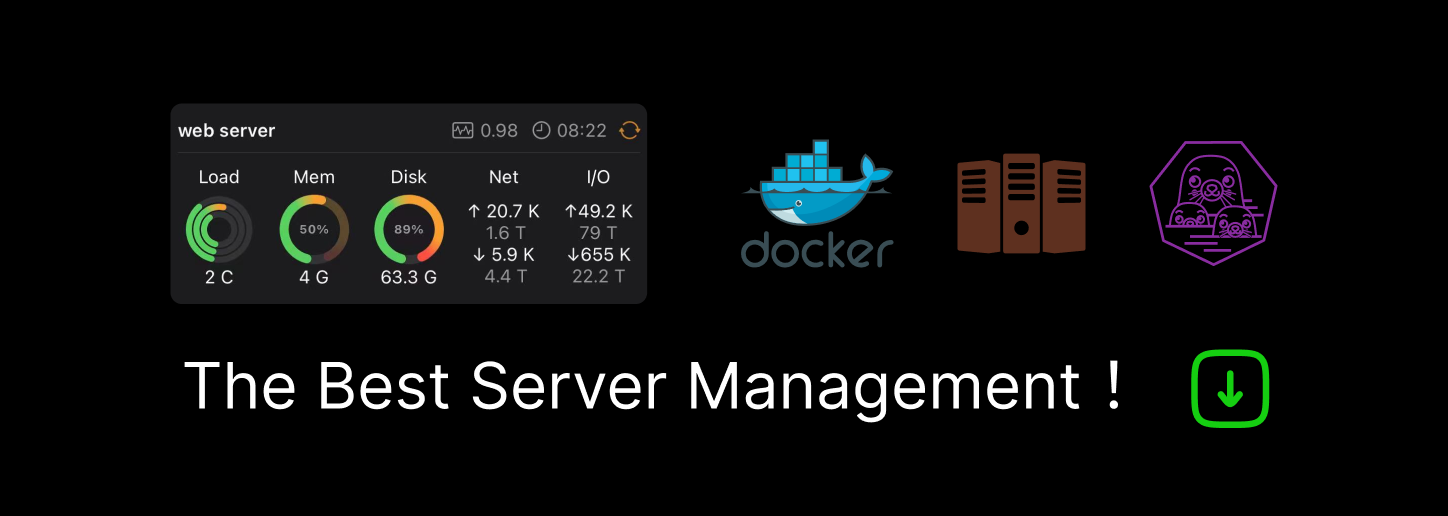
Make the Investment or Not? A Case Study Using Regression-Based Driver Analysis
Carol Haney and Elizabeth Dean , February 17, 2021
We often find organizations wondering how to use their data to make better decisions.
One of the tools that we frequently recommend is regression-based driver analysis. This approach helps to identify which underlying operational activities (drivers) will have the most impact on improving a metric (outcome) that you care about. In other words, it shows which of multiple drivers are most predictive of a specific outcome.
To help explain the approach, we’ll walk through how a retailer used it to prioritize its customer experience investments. Pre-COVID, a retail client of ours used a transactional feedback survey to keep track of five critical functional in-store drivers on a daily basis: cleanliness, stock availability, speed of checkout, knowledgeable staff, and friendliness of staff.
Retailer Example: To Invest In Cleanliness or Not?
In the month of June, the cleanliness driver’s satisfaction took a six-percentage point plummet, which was statistically different from May’s score. During that same period, the client’s outcome measure –“Likelihood to Return” — dropped a point, but the drop was not statistically significant.
The client launched into full fix-it-mode: All hands on deck! Review cleaning staff! Should we bring in new cleaning contractors? Should we upgrade store fixtures? Should we change the bathroom cleaning schedule? Pay close attention to trash cans in stores’ atriums!
These were all laudable and potential improvements, but the change in cleaning contractors and upgrade of store fixtures were going to cost the company thousands of dollars and significant staff time for retraining. Before committing to those investments, our client asked us to take a look at their data. The main question to answer before money was spent: are these activities worth the investment? And maybe even more fundamental: would the current state of cleanliness key customers from revisiting the store?
To find the answer based on existing data, we analyzed how the five critical functional in-store drivers affected the Likelihood to Return. Here’s what we did:
- First, we ran a linear regression with all five functional drivers as independent variables and the outcome measure – Likelihood to Return – as the dependent variable. When we did, we were surprised by the results: controlling for all the independent variables, speed of checkout, stock availability, and friendliness of staff were predictive of Likelihood to Return, but cleanliness and knowledgeable staff were not (given the current timeframe and current state of all five drivers).
- Second, we looked at the relative importance each of the driver variables had on Likelihood to Return. Relative Importance is an extension of regression that accounts for situations where the input variables (our driver variables) are correlated with one another, a very common issue in survey research. It allows us to see which driver variables are most important, relative to each other, in predicting our outcome variable. The results are shown as a series of percentages for each variable that collectively add up to 100%. It turned out that cleanliness had a very low relative importance on Likelihood to Return.
The analysis was pretty clear, the client had a directional issue to fix with cleanliness, but not an emergency and definitely not an issue that required thousands of dollars for new fixtures and other costly improvements.
The bottom line: Make smarter investment choices with regression-based driver analysis
Carol Haney, Ph.D., is a Distinguished Researcher with Qualtrics, specializing in CX
Elizabeth Dean, is a Senior XM Scientist with Qualtrics, specializing in market research design
Recommend
About Joyk
Aggregate valuable and interesting links.
Joyk means Joy of geeK