

Github the-do-loop-blog/blockBootstrap.sas at master · sascommunities/the-do-loo...
source link: https://github.com/sascommunities/the-do-loop-blog/blob/master/block-bootstrap/blockBootstrap.sas
Go to the source link to view the article. You can view the picture content, updated content and better typesetting reading experience. If the link is broken, please click the button below to view the snapshot at that time.
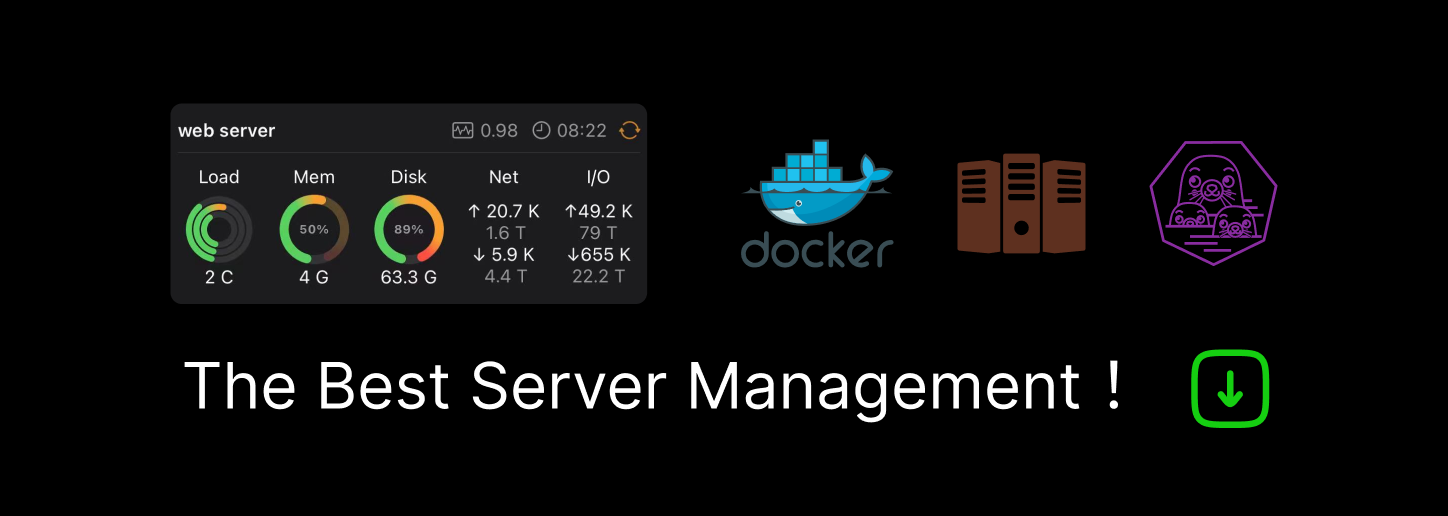
the-do-loop-blog/blockBootstrap.sas at master · sascommunities/the-do-loop-blog · GitHubPermalink
227 lines (201 sloc) 7.7 KB
/* SAS program to accompany the following articles: "The simple block bootstrap for time series in SAS" by Rick Wicklin, published 06JAN2021 on The DO Loop blog: https://blogs.sas.com/content/iml/2021/01/06/simple-block-bootstrap-sas.html "The moving block bootstrap for time series" by Rick Wicklin, published 13JAN2021 on The DO Loop blog: https://blogs.sas.com/content/iml/2021/01/13/moving-block-bootstrap-sas.html This program shows how to perform two bootstrap techniques for time series: the simple block bootstrap and the moving block bootstrap. */
/*********** THE DATA *************/ /* A time series requires block resampling of residuals, which are a stationary series. Case resampling for ordinary least squares regression https://blogs.sas.com/content/iml/2018/10/29/bootstrap-regression-residual-resampling.html can't be applied to time series because the error terms in a time series analysis are not assumes to be independent. Sashelp.Air has 144 months of data. Twelve months is a good block length, but that would result in 12 blocks of length 12. For clarity, let's drop the first year of data, which results in 11 blocks of length 12. */ data Air; set Sashelp.Air; if Date >= '01JAN1950'd; Time = _N_; run; title "Original Series: Air Travel"; proc sgplot data=Air; series x=Time y=Air; xaxis grid; yaxis grid; run;
/* Use AUTOREG to decompose series as Y = Predicted + Residuals Similar to Getting Started example in PROC AUTOREG */ proc autoreg data=Air plots=none outest=RegEst; AR12: model Air = Time / nlag=12; output out=OutReg pm=Pred rm=Resid; /* mean prediction and residuals */ ods select FinalModel.ParameterEstimates ARParameterEstimates; run;
title "Mean Prediction and Residuals from AR Model"; proc sgplot data=OutReg; series x=Time y=Pred; series x=Time y=Resid; refline 0 / axis=y; xaxis values=(24 to 144 by 12) grid valueshint; run;
/********* THE BOOTSTRAP **********/ /* Wikipedia has several forms of block bootstrap: 1. Simple block bootstrap: Choose block length, L. Use nonoverlapping blocks of size L. 2. Moving block bootstrap (MBB): B1=1:L, B2=2:L+1, etc. Randomly choose set of n/L blocks. 3. Stationary bootstrap: Randomly vary block length. Politis & Romano (1994), "The Stationary Bootstrap," JASA, 89 (428): 1303–1313. */ /**************************/ /* SIMPLE BLOCK BOOTSTRAP */ /**************************/ %let L = 12; proc iml; call randseed(12345);
/* the original series is Y = Pred + Resid */ use OutReg; read all var {'Time' 'Pred' 'Resid'}; close;
/* For the Simple Block Bootstrap, the length of the series (n) must be divisible by the block length (L). */ n = nrow(Pred); /* length of series */ L = &L; /* length of each block */ k = n / L; /* number of non-overlapping blocks */ if k ^= int(k) then ABORT "The series length is not divisible by the block length"; print n L k;
/* Trick: reshape data into k x L matrix. Each row is block of length L */ P = shape(Pred, k, L); R = shape(Resid, k, L); /* non-overlapping residuals (also k x L) */
/* Example: Illustrate one step of the Simple Block Bootstrap: generate a bootstrap resample by randomly mixing the residual blocks */ idx = sample(1:nrow(R), k); /* sample (w/ replacement) of size k from the set 1:k */ YBoot = P + R[idx,]; title "One Bootstrap Resample"; title2 "Simple Block Bootstrap"; refs = "refline " + char(do(12,nrow(Pred),12)) + " / axis=x;"; call series(Time, YBoot) other=refs; /*---- end of example ----*/ /* The simple block bootstrap repeats this process B times and usually writes the resamples to a SAS data set. */ B = 1000; J = nrow(R); /* J=k for non-overlapping blocks, but prepare for moving blocks */ SampleID = j(n,1,.); create BootOut var {'SampleID' 'Time' 'YBoot'}; /* create outside of loop */ do i = 1 to B; SampleId[,] = i; /* fill array. See https://blogs.sas.com/content/iml/2013/02/18/empty-subscript.html */ idx = sample(1:J, k); /* sample of size k from the set 1:k */ YBoot = P + R[idx,]; append; /* append each bootstrap sample */ end; close BootOut; QUIT;
/* Analyze the bootstrap samples by using a BY statement. See https://blogs.sas.com/content/iml/2012/07/18/simulation-in-sas-the-slow-way-or-the-by-way.html */ proc autoreg data=BootOut plots=none outest=BootEst noprint; by SampleID; AR12: model YBoot = Time / nlag=12; run;
/* OPTIONAL: Use PROC MEANS or PROC UNIVARIATE to estimate standard errors and CIs */ proc means data=BootEst mean stddev P5 P95; var Intercept Time; run;
title "Distribution of Parameter Estimates"; proc sgplot data=BootEst; scatter x=Intercept y=Time; xaxis grid; yaxis grid; refline 77.5402 / axis=x; refline 2.7956 / axis=y; run; /* You can also bootstrap other statistics, such as the AR estimates */ /* title "Distribution of Two AR Estimates"; proc sgplot data=BootEst; scatter x=_A_1 y=_A_2; xaxis grid; yaxis grid; refline -0.829192 / axis=x; refline 0.514420 / axis=y; run; */ /**************************/ /* MOVING BLOCK BOOTSTRAP */ /**************************/ %let L = 12; proc iml; call randseed(12345);
use OutReg; read all var {'Time' 'Pred' 'Resid'}; close;
/* Restriction for Simple Block Bootstrap: The length of the series (n) must be divisible by the number of blocks (k) so that all blocks have the same length (L) */ n = nrow(Pred); /* length of series */ L = &L; /* length of each block */ k = n / L; /* number of random blocks to use */ if k ^= int(k) then ABORT "The series length is not divisible by the block length";
/* Trick: Reshape data into k x L matrix. Each row is block of length L */ P = shape(Pred, k, L); /* there are k rows for Pred */ J = n - L + 1; /* total number of overlapping blocks to choose from */ R = j(J, L, .); /* there are n-L+1 blocks of residuals */ Resid = rowvec(Resid); /* make Resid into row vector so we don't need to transpose each row */ do i = 1 to J; R[i,] = Resid[ , i:i+L-1]; /* fill each row with a block of residuals */ end;
/*---- Example ----*/ /* illustrate one random bootstrap resample */ idx = sample(1:J, k); /* sample of size k from the set 1:J */ YBoot = P + R[idx,]; title "One Bootstrap Resample"; title2 "Moving Block Bootstrap"; refs = "refline " + char(do(12,nrow(Pred),12)) + " / axis=x;"; call series(Time, YBoot) other=refs; /*---- end of example ----*/
/* The moving block bootstrap repeats this process B times and usually writes the resamples to a SAS data set. */ B = 1000; SampleID = j(n,1,.); create BootOut var {'SampleID' 'Time' 'YBoot'}; /* create outside of loop */ do i = 1 to B; SampleId[,] = i; idx = sample(1:J, k); /* sample of size k from the set 1:J */ YBoot = P + R[idx,]; append; end; close BootOut; QUIT;
/* Analyze the bootstrap samples by using a BY statement. See https://blogs.sas.com/content/iml/2012/07/18/simulation-in-sas-the-slow-way-or-the-by-way.html */ proc autoreg data=BootOut plots=none outest=BootEst noprint; by SampleID; AR12: model YBoot = Time / nlag=12; run;
/* OPTIONAL: Use PROC MEANS or PROC UNIVARIATE to estimate standard errors and CIs */ proc means data=BootEst mean stddev P5 P95; var Intercept Time _A:; run;
title "Distribution of Parameter Estimates"; proc sgplot data=BootEst; scatter x=Intercept y=Time; *ellipse x=Intercept y=Time; xaxis grid; yaxis grid; refline 77.5402 / axis=x; refline 2.7956 / axis=y; run; /* title "Distribution of Two AR Estimates"; proc sgplot data=BootEst; scatter x=_A_1 y=_A_2; ellipse x=_A_1 y=_A_2; xaxis grid; yaxis grid; refline -0.829192 / axis=x; refline 0.514420 / axis=y; run; */
Recommend
About Joyk
Aggregate valuable and interesting links.
Joyk means Joy of geeK