

Woo for its own sake | Locklin on science
source link: https://scottlocklin.wordpress.com/2021/01/08/woo-for-its-own-sake/
Go to the source link to view the article. You can view the picture content, updated content and better typesetting reading experience. If the link is broken, please click the button below to view the snapshot at that time.
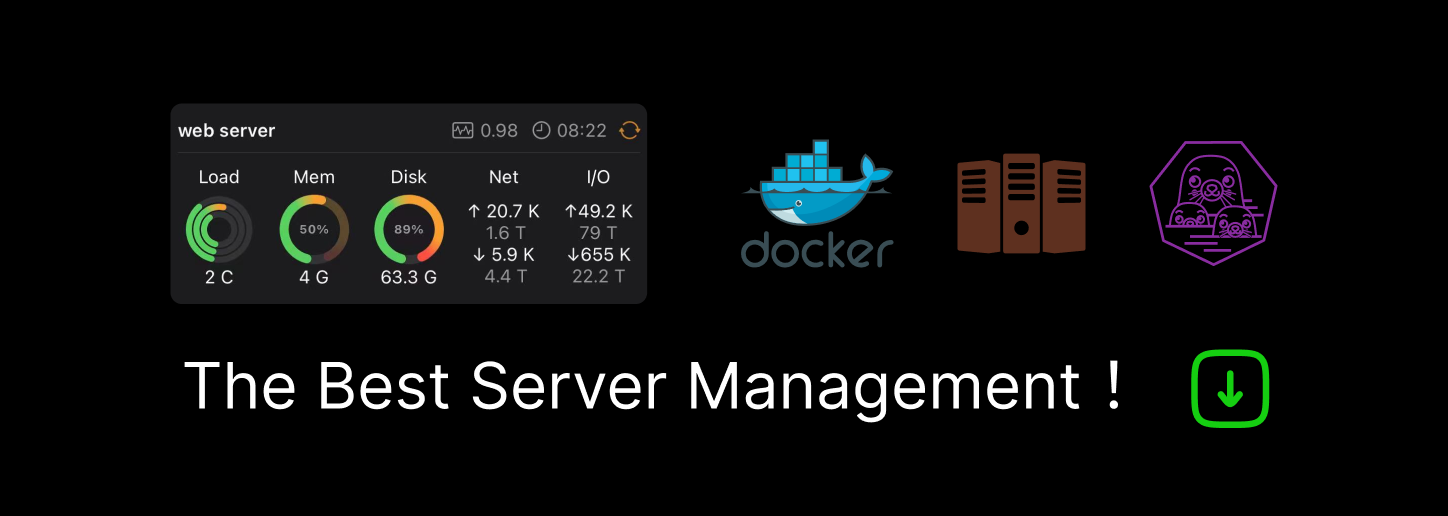
Woo for its own sake
Software development is a funny profession. It covers people who do stuff ranging from register twiddling in device drivers and OS guts, to people who serve web content, to “big data” statisticians, to devops infrastructure, to people who write javascript and html front ends on electron apps. To a certain extent, software engineering is grossly underpaid. If software engineers were allowed to capture more of the value they create, we’d have vastly fewer billionaires and more software engineers with normal upper middle class lifestyles, such as houses owned in the clear and successful reproductive lifecycles. The underpaid are often compensated in self esteem.
By “compensated in self esteem” I don’t mean they have high self esteem; I mean the manager saying “dude yer so fookin smart brah” kind. This is the same brainlet payment system in place in the present day “hard sciences” with people writing bullshit papers nobody cares about, or, like, journalists and other “twitter activists” who believe themselves to be intellectual workers rather than the snitches and witch hunters they actually are. Basically, nerd gets a pat on the head instead of a paycheck.
Once in a while, independent minded programmers demand more. They may or may not be “so fookin smart,” but they think they are. Their day jobs consist of unpleasant plumbing tasks, keeping various Rube Goldberg contraptions functioning and generally eating soylent and larva-burgers and claiming to like it. As such, most programmers long to do something fancy, like develop a web server based on Category Theory, or write a stack of really cool lisp macros for generating ad server callbacks, or add some weird new programming language of dubious utility to an already complex and fragile stack.

Allowing your unicycle-riding silver pants mentat to write the prototype in Haskell to keep him from getting a job at the Hedge Fund may make some HR sense. But if you’re going to rewrite the thing in Java so a bunch of offshore midwits can keep it running, maybe the “adulting” thing to do is just write it in Java in the first place.
I’m not shitting on Haskell in particular, though there is an argument to be made for looking askance at using it in production. Haskell is mostly a researchy/academicy language. I don’t know, but I strongly suspect its run of the mill libraries dealing with stuff like network and storage is weak and not fully debugged. Why do I suspect this? In part from casual observation, but also from sociology. Haskell is a fancy language with people doing fancy things in it. One of the valuable things about popular but boring languages is that the code has been traversed many times, and routine stuff you’re likely to use in production is probably well debugged. This isn’t always true, but it’s mostly true. The other benefit to boring languages is people concentrate on the problem, rather than the interesting complexities of the language itself.
You see it in smaller ways too; people who feel like every line of code has to be innovative: new elliptic curves, new network protocols, new block ciphers, new ZNP systems; to a crucial money oriented application that would have been really cool and have a much smaller attack surface if you had bestowed only one innovation on it. I guess this sort of thing is like bike-shedding or Yak-shaving, but it’s really something more perverse. If you have a job doing shit with computers, you are presumably solving real world problems which someone pays for. Maybe, you know, you should solve the problem instead of being a unicycle riding silver pants juggling chainsaws.
You see a lot of it in the cryptocurrency community, in part because there is enough money floating around, the lunatics are often running the asylum, in part for its undeserved reputation as being complicated (it’s just a shared database with rules and checksums; Bram more or less did the hard part in the summer of 2000 while my buddy Gerald was sleeping on his couch). For example: this atrocity by Gnosis. Gnosis is an interesting project which I hope is around for a long time. They’re doing a ton of very difficult things. Recently they decided to offer multi-token batch auctions. Why? I have no freaking idea. It’s about as necessary and in demand as riding to work in silver pants on a unicycle. Worse though: from an engineering perspective, it involves mixed integer programming, which is, as every sane person knows, NP-hard.
This is a danger in putting software developers or programmers in charge. These guys are often child-like in their enthusiasm for new and shiny things. Engineers are different: they’re trying to solve a problem. Engineers understand it’s OK to solve the problem with ephemeral, trashy, but fast-to-market solutions if the product manager is going to change it all next week. Engineers also plan for the future when the software is critical infrastructure that lives and fortunes may depend on. Engineers don’t build things that require mixed integer programming unless it’s absolutely necessary to solve a real world problem. If they juggle on unicycles, they do it on their own time; not at work.
Consider an engineering solution for critical infrastructure from a previous era; that of providing motive power for small fishing boats. Motors were vastly superior to sail for this task. In the early days of motorized fishing, in some cases until fairly recently, there was no radio to call for help if something goes wrong. You’re out there in the vastness on your own; possibly by yourself, with nothing but your wits and your vessel. There’s probably not much in the way of supply lines when you’re at shore either. So the motors of the early days were extremely reliable. Few, robust moving parts, simple two stroke semi diesel operation, runs on any fuel, requires no electricity to start; just an old fashioned vaporizing torch which runs on your fuel; in a pinch you could start a fire of log books. You glance at such a thing and you know it is designed for robust operation. Indeed the same engines have been used more or less continuously for decades; they only turn at 500 rpm, and drive the propeller directly rather than through a gearbox.
Such engines are useful enough they remain in use to this day; new ones of roughly this design are still sold by the Sabb company in Norway. They’re not as environmentally friendly or fuel efficient as modern ones (though close in the latter measure), but they’re definitely more reliable where it counts. When you look at this in the engine room, you are filled with confidence Mr. Scott will keep the warp drives running. If you find some jackass on a unicycle back there (who will probably try to stick a solar powered Sterling engine in the thing), maybe not so much.
I don’t think long term software engineering looks much different from this. Stuff you can trust looks like a giant one-piston semidiesel. You make it out of well known, well traversed and well tested parts. There are a couple of well regarded essays on the boringness yet awesomeness of golang. Despite abundant disagreement I think there is a lot to that. Nobody writes code in golang because of its extreme beauty or interesting abstractions. It is a boring garbage collected thing that looks like C for grownups, or Java not designed by 90s era object oriented nanotech fearing imbeciles. I think it bothers a lot of people that it’s not complicated enough. I’m not shilling for it, but I think anyone who overlooks it for network oriented coding because it’s boring or they think it’s “slow” because it doesn’t use functors or borrow checkers or whatever is a unicycle riding idiot though. Again looking at blockchain land; Geth (written in golang) has mostly been a rock, where the (Rust) Parity team struggles to maintain parity with feature roll outs and eventually exploded into multiple code bases the last time I checked. There’s zero perceptible performance difference between them.
There’s a Joel Spolsky on (Peter Seibel interview with) JWZ which I always related to on complexification of the software process:
One principle duct tape programmers understand well is that any kind of coding technique that’s even slightly complicated is going to doom your project. Duct tape programmers tend to avoid C++, templates, multiple inheritance, multithreading, COM, CORBA, and a host of other technologies that are all totally reasonable, when you think long and hard about them, but are, honestly, just a little bit too hard for the human brain.
Sure, there’s nothing officially wrong with trying to write multithreaded code in C++ on Windows using COM. But it’s prone to disastrous bugs, the kind of bugs that only happen under very specific timing scenarios, because our brains are not, honestly, good enough to write this kind of code. Mediocre programmers are, frankly, defensive about this, and they don’t want to admit that they’re not able to write this super-complicated code, so they let the bullies on their team plow away with some godforsaken template architecture in C++ because otherwise they’d have to admit that they just don’t feel smart enough to use what would otherwise be a perfectly good programming technique FOR SPOCK. Duct tape programmers don’t give a shit what you think about them. They stick to simple basic and easy to use tools and use the extra brainpower that these tools leave them to write more useful features for their customers.
I don’t think this captures the perverseness and destructiveness of people who try to get fancy for no reason, nor do I think JWZ was a “duct tape programmer” -he was an engineer, and that’s why his products actually shipped.
I say this as an aficionado of a couple of fancy and specialized languages I use on a regular basis. I know that it is possible to increase programmer productivity through language choice, and often times, runtime performance really doesn’t suffer. Languages like OCaML, APL and Lisp have demonstrated that small teams can deliver complex high performance software that works reliably. Delphi and Labview are other examples of high productivity languages; the former for its amazing IDE, and the latter for representing state machines as flow charts and providing useful modules for hardware. The problem is that large teams probably can’t deliver complex high performance software that works reliably using these tools. One also must pay a high price up front in learning to deal with them at all, depending on where you come from (not so much with Labview). From a hiring manager or engineer’s perspective, the choice to develop in a weird high productivity language is fraught. What happens if the thing crashes at 4 in the morning? Do you have enough spare people someone can be raised on the telephone to fix it? What if it’s something up the dependency tree written by an eccentric who is usually mountaineering in the Alps? For mission critical production code, the human machine that keeps it running can’t be ignored. If your mentat gets hit by a bus or joins the circus as a unicycle juggler and the code breaks in production you’re in deep sheeyit. The idea that it won’t ever break because muh technology is retarded and the towers of jelly that are modern OS/language/framework stacks are almost without exception going to break when you update things.
The “don’t get fancy” maxim applies in spades to something like data science. There are abundant reasons to just use Naive Bayes in production code for something like sentiment analysis. They’re easy to debug and they have a trivial semi-supervised mode using the EM algorithm if you’re short of data. For unsupervised clustering or decomposition it’s hard to beat geometric approaches like single-linkage/dbscan or PCA. For regression or classification models, linear regression is pretty good, or gradient boost/random forest/KNN. Most of the time, your real problem is shitty data, so using the most accurate tool is completely useless.
Using the latest tool is even worse. 99 times out of 100, the latest woo in machine learning is not an actual improvement over existing techniques. 100% of the time it is touted as a great revolution because it beat some other technique … on a carefully curated data set. Such results are trumpeted by the researcher because …. WTF else do you expect them to do? They just spent a year or two developing a new technique; the professor is trying to get tenure or be a big kahuna, and the student is trying to get a job by being expert in the new technique. What are they going to tell you? That their new technique was kind of dumb and worthless?
I’ve fallen for this a number of times now; I will admit my sins. I fooled around a bit with t-SNE while I was at Ayasdi, and I could never get it to do anything sane. I just assumed I was a moron who couldn’t use this advanced piece of technology. No, actually, t-SNE is kind of bullshit; a glorified random number generator that once in a while randomly finds an interesting embedding. SAX looked cool because it embodied some ideas I had been fooling around with for almost a decade, but even the author admits it is horse shit. At this point when some new thing comes along, especially if people are talking about it in weeb-land forums, I pretty much ignore it, unless it is being touted to me by a person who has actually used it on a substantive problem with unambiguously excellent results. Matrix profiles looks like one of these; SAX dude dreamed it up, and like SAX, it appears to be an arbitrary collection of vaguely common sense things to do that’s pretty equivalent to any number of similar techniques dating back over the last 40 years.
There are innovations in data science tools. But most of them since boosting are pretty marginal in their returns, or only apply to corner cases you’re unlikely to encounter. Some make it easier to see what’s going on, some find problems with statistical estimators, but mostly you’re going to get better payoff by getting better at the basics. Everyone is so in love with woo, the guy who can actually do a solid estimate of mean differences is going to provide a lot more value than the guy who knows about the latest PR release from UC Riverside.
Good old numerical linear algebra, which everyone roundly ignores, is a more interesting subject than machine learning in current year. How many of you know about using CUR decompositions in your PCA calculations? Ever look at some sloppy PCA and wonder which rows/columns produced most of the variance? Well, that’s what a CUR decomposition is. Obviously looking at the top 3 most important of each isn’t going to be as accurate as looking at the regular PCA, but it sure can be helpful. Nuclear Norm and non-negative matrix factorizations all look like they do useful things. They don’t get shilled; just quietly used by engineering types who find them helpful.

Recommend
About Joyk
Aggregate valuable and interesting links.
Joyk means Joy of geeK