

Building Diversified Portfolios that Outperform Out-of-Sample
source link: https://papers.ssrn.com/sol3/papers.cfm?abstract_id=2708678
Go to the source link to view the article. You can view the picture content, updated content and better typesetting reading experience. If the link is broken, please click the button below to view the snapshot at that time.
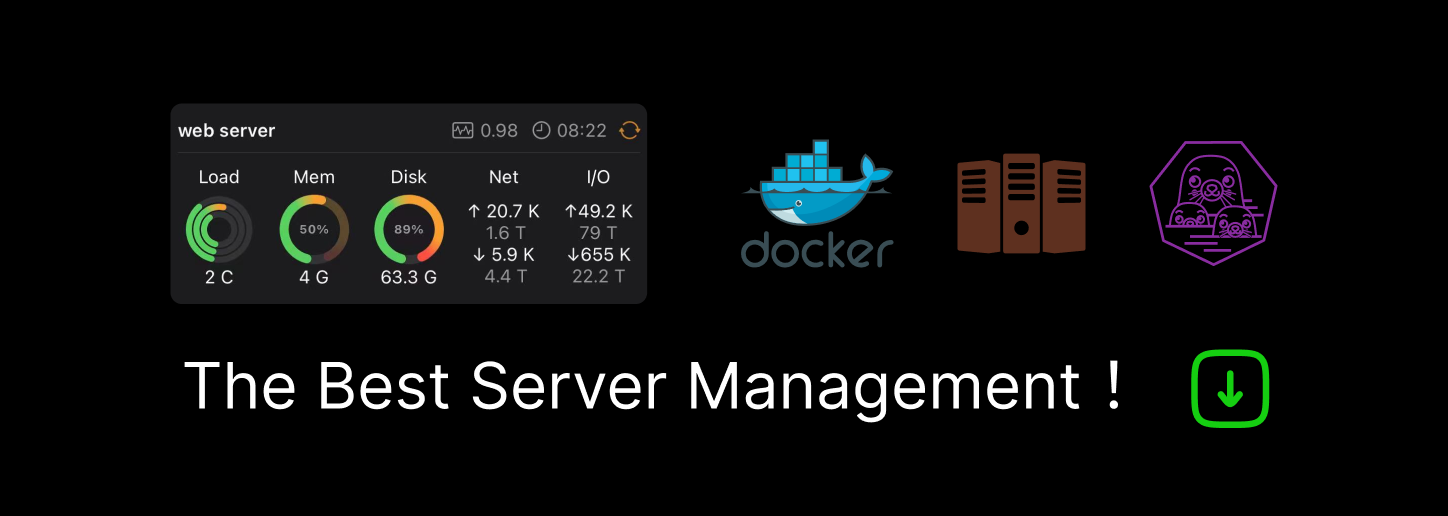
Building Diversified Portfolios that Outperform Out-of-Sample
31 Pages
Posted: 17 Jul 2019
Marcos Lopez de Prado
Cornell University - Operations Research & Industrial Engineering; True Positive Technologies
Date Written: May 23, 2016
Abstract
This paper introduces the Hierarchical Risk Parity (HRP) approach. HRP portfolios address three major concerns of quadratic optimizers in general and Markowitz’s CLA in particular: Instability, concentration and underperformance.
HRP applies modern mathematics (graph theory and machine learning techniques) to build a diversified portfolio based on the information contained in the covariance matrix. However, unlike quadratic optimizers, HRP does not require the invertibility of the covariance matrix. In fact, HRP can compute a portfolio on an ill-degenerated or even a singular covariance matrix, an impossible feat for quadratic optimizers. Monte Carlo experiments show that HRP delivers lower out-of-sample variance than CLA, even though minimum-variance is CLA’s optimization objective. HRP also produces less risky portfolios out-of-sample compared to traditional risk parity methods.
A presentation can be found at http://ssrn.com/abstract=2713516.
Keywords: Risk parity, tree graph, cluster, dendogram, linkage, metric space
JEL Classification: G0, G1, G2, G15, G24, E44
Suggested Citation: Suggested Citation
Recommend
About Joyk
Aggregate valuable and interesting links.
Joyk means Joy of geeK