

83 项开源视觉 SLAM 方案够你用了吗?
source link: https://mp.weixin.qq.com/s/aIyqItWndQsooJ8-o3kSoA
Go to the source link to view the article. You can view the picture content, updated content and better typesetting reading experience. If the link is broken, please click the button below to view the snapshot at that time.
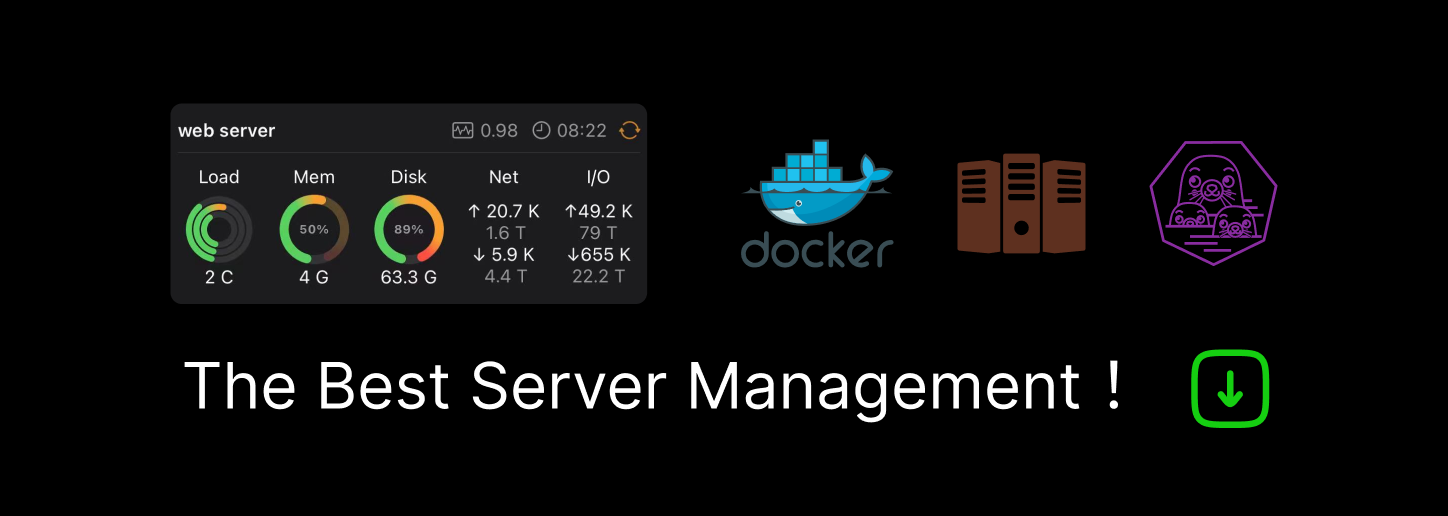
83 项开源视觉 SLAM 方案够你用了吗?
点击上方“3D视觉工坊”,选择“星标”
干货第一时间送达
前言
1. 本文由知乎作者小吴同学同步发布于https://zhuanlan.zhihu.com/p/115599978/并持续更新。
2. 本文简单将各种开源视觉SLAM方案分为以下 7 类(固然有不少文章无法恰当分类):
·Geometric SLAM
·Semantic / Learning SLAM
·Multi-Landmarks / Object SLAM
·VIO / VISLAM
·Dynamic SLAM
·Mapping
·Optimization
3. 由于本人自 2019 年 3 月开始整理,所以以下的代码除了经典的框架之外基本都集中在 19-20 年;此外个人比较关注 VO、物体级 SLAM 和多路标 SLAM,所以以下内容收集的也不完整,无法涵盖视觉 SLAM 的所有研究,仅作参考。
一、Geometric SLAM(20 项)
这一类是传统的基于特征点、直接法或半直接法的 SLAM,虽说传统,但 2019 年也新诞生了 9 个开源方案。
1. PTAM
论文:Klein G, Murray D. Parallel tracking and mapping for small AR workspaces[C]//Mixed andAugmented Reality, 2007. ISMAR 2007. 6th IEEE and ACM International Symposiumon. IEEE, 2007: 225-234.
代码:https://github.com/Oxford-PTAM/PTAM-GPL
工程地址:http://www.robots.ox.ac.uk/~gk/PTAM/
作者其他研究:http://www.robots.ox.ac.uk/~gk/publications.html
2. S-PTAM(双目 PTAM)
论文:Taihú Pire,Thomas Fischer, Gastón Castro,Pablo De Cristóforis, Javier Civera and Julio Jacobo Berlles. S-PTAM: Stereo Parallel Tracking and Mapping. Robotics and AutonomousSystems, 2017.
代码:https://github.com/lrse/sptam
作者其他论文:Castro G,Nitsche M A, Pire T, et al. Efficient on-board Stereo SLAM throughconstrained-covisibility strategies[J]. Robotics and Autonomous Systems, 2019.
3. MonoSLAM
论文:Davison A J, Reid I D, Molton N D, et al. MonoSLAM:Real-time single camera SLAM[J]. IEEE transactions on patternanalysis and machine intelligence, 2007, 29(6): 1052-1067.
代码:https://github.com/hanmekim/SceneLib2
4. ORB-SLAM2
论文:Mur-Artal R, Tardós J D. Orb-slam2: Anopen-source slam system for monocular, stereo, and rgb-d cameras[J]. IEEETransactions on Robotics, 2017, 33(5): 1255-1262.
代码:https://github.com/raulmur/ORB_SLAM2
作者其他论文:
单目半稠密建图:Mur-Artal R, Tardós J D. Probabilistic Semi-Dense Mapping from Highly AccurateFeature-Based Monocular SLAM[C]//Robotics: Science and Systems. 2015,2015.
VIORB:Mur-Artal R, Tardós J D. Visual-inertialmonocular SLAM with map reuse[J]. IEEE Robotics and AutomationLetters, 2017, 2(2): 796-803.
多地图:Elvira R, Tardós J D, Montiel J M M. ORBSLAM-Atlas: arobust and accurate multi-map system[J]. arXiv preprint arXiv:1908.11585, 2019.
以下 5, 6, 7, 8 几项是 TUM 计算机视觉组全家桶
5. DSO
论文:Engel J, Koltun V, Cremers D. Direct sparseodometry[J]. IEEE transactions on pattern analysis and machineintelligence, 2017, 40(3): 611-625.
代码:https://github.com/JakobEngel/dso
双目 DSO:Wang R, Schworer M, Cremers D. Stereo DSO: Large-scale direct sparse visual odometry withstereo cameras[C]//Proceedings of the IEEE International Conference onComputer Vision. 2017: 3903-3911.
VI-DSO:Von Stumberg L, Usenko V, Cremers D. Direct sparsevisual-inertial odometry using dynamic marginalization[C]//2018 IEEEInternational Conference on Robotics and Automation (ICRA). IEEE, 2018:2510-2517.
6. LDSO
高翔在 DSO 上添加闭环的工作
论文:Gao X, Wang R, Demmel N, et al. LDSO: Directsparse odometry with loop closure[C]//2018 IEEE/RSJ InternationalConference on Intelligent Robots and Systems (IROS). IEEE, 2018:2198-2204.
代码:https://github.com/tum-vision/LDSO
7. LSD-SLAM
论文:Engel J, Schöps T, Cremers D. LSD-SLAM: Large-scale direct monocular SLAM[C]//Europeanconference on computer vision. Springer, Cham, 2014: 834-849.
代码:https://github.com/tum-vision/lsd_slam
8. DVO-SLAM
论文:Kerl C, Sturm J, Cremers D. Dense visualSLAM for RGB-D cameras[C]//2013 IEEE/RSJ International Conferenceon Intelligent Robots and Systems. IEEE, 2013: 2100-2106.
代码 1:https://github.com/tum-vision/dvo_slam
代码 2:https://github.com/tum-vision/dvo
其他论文:
Kerl C, Sturm J,Cremers D. Robust odometry estimation for RGB-D cameras[C]//2013 IEEEinternational conference on robotics and automation. IEEE, 2013:3748-3754.
Steinbrücker F,Sturm J, Cremers D. Real-time visual odometry from dense RGB-D images[C]//2011 IEEEinternational conference on computer vision workshops (ICCV Workshops). IEEE, 2011:719-722.
9. SVO
苏黎世大学机器人与感知课题组
论文:Forster C, Pizzoli M, Scaramuzza D. SVO: Fast semi-direct monocular visual odometry[C]//2014 IEEEinternational conference on robotics and automation (ICRA). IEEE, 2014:15-22.
代码:https://github.com/uzh-rpg/rpg_svo
Forster C, ZhangZ, Gassner M, et al. SVO: Semidirect visual odometry for monocular andmulticamera systems[J]. IEEE Transactions on Robotics, 2016,33(2): 249-265.
10. DSM
论文:Zubizarreta J, Aguinaga I, Montiel J M M. Direct sparsemapping[J]. arXiv preprint arXiv:1904.06577, 2019.
代码:https://github.com/jzubizarreta/dsm
11. openvslam
论文:Sumikura S,Shibuya M, Sakurada K. OpenVSLAM: A Versatile Visual SLAM Framework[C]//Proceedingsof the 27th ACM International Conference on Multimedia. 2019: 2292-2295.
代码:https://github.com/xdspacelab/openvslam
12. se2lam(地面车辆位姿估计的视觉里程计)
论文:Zheng F, Liu Y H. Visual-OdometricLocalization and Mapping for Ground Vehicles Using SE (2)-XYZ Constraints[C]//2019International Conference on Robotics and Automation (ICRA). IEEE, 2019:3556-3562.
代码:https://github.com/izhengfan/se2lam
作者的另外一项工作
论文:Zheng F, Tang H,Liu Y H. Odometry-vision-basedground vehicle motion estimation with se (2)-constrained se (3) poses[J]. IEEEtransactions on cybernetics, 2018, 49(7): 2652-2663.
代码:https://github.com/izhengfan/se2clam
13. GraphSfM(基于图的并行大尺度 SFM)
论文:Chen Y, Shen S,Chen Y, et al. Graph-BasedParallel Large Scale Structure from Motion[J]. arXivpreprint arXiv:1912.10659, 2019.
代码:https://github.com/AIBluefisher/GraphSfM
14. LCSD_SLAM(松耦合的半直接法单目 SLAM)
论文:Lee S H, Civera J. Loosely-Coupledsemi-direct monocular SLAM[J]. IEEE Robotics and AutomationLetters, 2018, 4(2): 399-406.
代码:https://github.com/sunghoon031/LCSD_SLAM;谷歌学术 ;演示视频
作者另外一篇关于单目尺度的文章代码开源:Lee S H, deCroon G. Stability-based scale estimation for monocular SLAM[J]. IEEERobotics and Automation Letters, 2018, 3(2): 780-787.
15. RESLAM(基于边的 SLAM)
论文:Schenk F, Fraundorfer F. RESLAM: Areal-time robust edge-based SLAM system[C]//2019 International Conference onRobotics and Automation (ICRA). IEEE, 2019: 154-160.
代码:https://github.com/fabianschenk/RESLAM
16. scale_optimization(将单目 DSO 拓展到双目)
论文:Mo J, Sattar J. ExtendingMonocular Visual Odometry to Stereo Camera System by Scale Optimization[C].International Conference on Intelligent Robots and Systems (IROS), 2019.
代码:https://github.com/jiawei-mo/scale_optimization
17. BAD-SLAM(直接法 RGB-D SLAM)
论文:Schops T, Sattler T, Pollefeys M. BAD SLAM: Bundle Adjusted Direct RGB-D SLAM[C]//Proceedingsof the IEEE Conference on Computer Vision and Pattern Recognition. 2019:134-144.
代码:https://github.com/ETH3D/badslam
18. GSLAM(集成 ORB-SLAM2,DSO,SVO 的通用框架)
论文:Zhao Y, Xu S, Bu S, et al. GSLAM: A general SLAM framework and benchmark[C]//Proceedingsof the IEEE International Conference on Computer Vision. 2019:1110-1120.
代码:https://github.com/zdzhaoyong/GSLAM
19. ARM-VO(运行于 ARM 处理器上的单目 VO)
论文:Nejad Z Z, Ahmadabadian A H. ARM-VO: an efficient monocular visual odometry for groundvehicles on ARM CPUs[J]. Machine Vision and Applications, 2019:1-10.
代码:https://github.com/zanazakaryaie/ARM-VO
20. cvo-rgbd(直接法 RGB-D VO)
论文:Ghaffari M, Clark W, Bloch A, et al. ContinuousDirect Sparse Visual Odometry from RGB-D Images[J]. arXivpreprint arXiv:1904.02266, 2019.
代码:https://github.com/MaaniGhaffari/cvo-rgbd
二、Semantic / Learning SLAM(12 项)
SLAM 与深度学习相结合的工作当前主要体现在两个方面,一方面是将语义信息参与到建图、位姿估计等环节中,另一方面是端到端地完成 SLAM 的某一个步骤(比如 VO,闭环等)。个人对后者没太关注,也同样欢迎大家在issue中分享。
21. MsakFusion
论文:Runz M, Buffier M, Agapito L. Maskfusion:Real-time recognition, tracking and reconstruction of multiple moving objects[C]//2018 IEEEInternational Symposium on Mixed and Augmented Reality (ISMAR). IEEE, 2018:10-20.
代码:https://github.com/martinruenz/maskfusion
22. SemanticFusion
论文:McCormac J, Handa A, Davison A, et al. Semanticfusion:Dense 3d semantic mapping with convolutional neural networks[C]//2017 IEEEInternational Conference on Robotics and automation (ICRA). IEEE, 2017:4628-4635.
代码:https://github.com/seaun163/semanticfusion
23. semantic_3d_mapping
论文:Yang S, Huang Y, Scherer S. Semantic 3Doccupancy mapping through efficient high order CRFs[C]//2017IEEE/RSJ International Conference on Intelligent Robots and Systems (IROS).IEEE, 2017: 590-597.
代码:https://github.com/shichaoy/semantic_3d_mapping
24. Kimera(实时度量与语义定位建图开源库)
论文:Rosinol A, AbateM, Chang Y, et al. Kimera: anOpen-Source Library for Real-Time Metric-Semantic Localization and Mapping[J]. arXivpreprint arXiv:1910.02490, 2019.
代码:https://github.com/MIT-SPARK/Kimera
25. NeuroSLAM(脑启发式 SLAM)
论文:Yu F, Shang J, Hu Y, et al. NeuroSLAM: a brain-inspired SLAM system for 3Denvironments[J]. Biological Cybernetics, 2019: 1-31.
代码:https://github.com/cognav/NeuroSLAM
第四作者就是 Rat SLAM 的作者,文章也比较了十余种脑启发式的 SLAM
26. gradSLAM(自动分区的稠密 SLAM)
论文:Jatavallabhula K M, Iyer G, Paull L. gradSLAM:Dense SLAM meets Automatic Differentiation[J]. arXivpreprint arXiv:1910.10672, 2019.
代码(预计 20 年 4 月放出):https://github.com/montrealrobotics/gradSLAM
27. ORB-SLAM2 + 目标检测/分割的方案语义建图
https://github.com/floatlazer/semantic_slam
https://github.com/qixuxiang/orb-slam2_with_semantic_labelling
https://github.com/Ewenwan/ORB_SLAM2_SSD_Semantic
28. SIVO(语义辅助特征选择)
论文:Ganti P, Waslander S. NetworkUncertainty Informed Semantic Feature Selection for Visual SLAM[C]//2019 16thConference on Computer and Robot Vision (CRV). IEEE, 2019: 121-128.
代码:https://github.com/navganti/SIVO
29. FILD(临近图增量式闭环检测)
论文:Shan An, Guangfu Che, Fangru Zhou,Xianglong Liu, Xin Ma, Yu Chen.Fast and Incremental Loop Closure Detection usingProximity Graphs. pp. 378-385, The 2019 IEEE/RSJ International Conferenceon Intelligent Robots and Systems (IROS2019)
代码:https://github.com/AnshanTJU/FILD
30. object-detection-sptam(目标检测与双目 SLAM)
论文:Pire T, Corti J, Grinblat G. Online Object Detection and Localization on Stereo VisualSLAM System[J]. Journal of Intelligent & Robotic Systems, 2019:1-10.
代码:https://github.com/CIFASIS/object-detection-sptam
31. Map Slammer(单目深度估计 + SLAM)
论文:Torres-Camara J M, Escalona F, Gomez-DonosoF, et al. Map Slammer: Densifying Scattered KSLAM 3D Maps withEstimated Depth[C]//Iberian Robotics conference. Springer, Cham, 2019:563-574.
代码:https://github.com/jmtc7/mapSlammer
32. NOLBO(变分模型的概率 SLAM)
论文:Yu H, Lee B. Not Only LookBut Observe: Variational Observation Model of Scene-Level 3D Multi-ObjectUnderstanding for Probabilistic SLAM[J]. arXiv preprint arXiv:1907.09760, 2019.
代码:https://github.com/bogus2000/NOLBO
三、Multi-Landmarks / Object SLAM(12 项)
其实多路标的点、线、平面 SLAM 和物体级 SLAM 完全可以分类在 Geometric SLAM 和 Semantic SLAM 中,但个人对这一方向比较感兴趣(也是我的研究生课题),所以将其独立出来,开源方案相对较少,但很有意思。
33. PL-SVO(点线 SVO)
论文:Gomez-Ojeda R, Briales J, Gonzalez-JimenezJ. PL-SVO: Semi-direct Monocular Visual Odometry by combiningpoints and line segments[C]//Intelligent Robots and Systems(IROS), 2016 IEEE/RSJ International Conference on. IEEE, 2016:4211-4216.
代码:https://github.com/rubengooj/pl-svo
34. stvo-pl(双目点线 VO)
论文:Gomez-Ojeda R, Gonzalez-Jimenez J. Robust stereo visual odometry through a probabilisticcombination of points and line segments[C]//2016 IEEE International Conferenceon Robotics and Automation (ICRA). IEEE, 2016: 2521-2526.
代码:https://github.com/rubengooj/stvo-pl
35. PL-SLAM(点线 SLAM)
论文:Gomez-Ojeda R, Zuñiga-Noël D, Moreno F A,et al. PL-SLAM: aStereo SLAM System through the Combination of Points and Line Segments[J]. arXivpreprint arXiv:1705.09479, 2017.
代码:https://github.com/rubengooj/pl-slam
Gomez-Ojeda R,Moreno F A, Zuñiga-Noël D, et al.PL-SLAM: a stereo SLAM system through the combination ofpoints and line segments[J]. IEEE Transactions on Robotics, 2019,35(3): 734-746.
36. PL-VIO
论文:He Y, Zhao J, Guo Y, et al. PL-VIO:Tightly-coupled monocular visual–inertial odometry using point and linefeatures[J]. Sensors, 2018, 18(4): 1159.
代码:https://github.com/HeYijia/PL-VIO
VINS + 线段:https://github.com/Jichao-Peng/VINS-Mono-Optimization
37. lld-slam(用于 SLAM 的可学习型线段描述符)
论文:Vakhitov A, Lempitsky V. Learnable line segment descriptor for visual SLAM[J]. IEEEAccess, 2019, 7: 39923-39934.
代码:https://github.com/alexandervakhitov/lld-slam;Video
点线结合的工作还有很多,国内的比如 + 上交邹丹平老师的 Zou D, Wu Y, Pei L, et al. StructVIO:visual-inertial odometry with structural regularity of man-made environments[J]. IEEETransactions on Robotics, 2019, 35(4): 999-1013. + 浙大的 Zuo X, Xie X, Liu Y, et al. Robust visualSLAM with point and line features[C]//2017 IEEE/RSJ InternationalConference on Intelligent Robots and Systems (IROS). IEEE, 2017:1775-1782.
38. PlaneSLAM
论文:Wietrzykowski J. On the representation of planes for efficient graph-basedslam with high-level features[J]. Journal of Automation MobileRobotics and Intelligent Systems, 2016, 10.
代码:https://github.com/LRMPUT/PlaneSLAM
作者另外一项开源代码,没有找到对应的论文:https://github.com/LRMPUT/PUTSLAM
39. Eigen-Factors(特征因子平面对齐)
论文:Ferrer G. Eigen-Factors: Plane Estimation for Multi-Frame andTime-Continuous Point Cloud Alignment[C]//2019 IEEE/RSJ InternationalConference on Intelligent Robots and Systems (IROS). IEEE, 2019:1278-1284.
代码:https://gitlab.com/gferrer/eigen-factors-iros2019
40. PlaneLoc
论文:Wietrzykowski J, Skrzypczyński P. PlaneLoc:Probabilistic global localization in 3-D using local planar features[J]. Roboticsand Autonomous Systems, 2019, 113: 160-173.
代码:https://github.com/LRMPUT/PlaneLoc
41. Pop-up SLAM
论文:Yang S, Song Y, Kaess M, et al. Pop-up slam:Semantic monocular plane slam for low-texture environments[C]//2016IEEE/RSJ International Conference on Intelligent Robots and Systems (IROS).IEEE, 2016: 1222-1229.
代码:https://github.com/shichaoy/pop_up_slam
42. Object SLAM
论文:Mu B, Liu S Y, Paull L, et al. Slam withobjects using a nonparametric pose graph[C]//2016 IEEE/RSJ InternationalConference on Intelligent Robots and Systems (IROS). IEEE, 2016:4602-4609.
代码:https://github.com/BeipengMu/objectSLAM
43. voxblox-plusplus(物体级体素建图)
论文:Grinvald M, Furrer F, Novkovic T, et al. Volumetricinstance-aware semantic mapping and 3D object discovery[J]. IEEERobotics and Automation Letters, 2019, 4(3): 3037-3044.
代码:https://github.com/ethz-asl/voxblox-plusplus
44. Cube SLAM
论文:Yang S, Scherer S. Cubeslam:Monocular 3-d object slam[J]. IEEE Transactions on Robotics, 2019,35(4): 925-938.
代码:https://github.com/shichaoy/cube_slam
也有很多有意思的但没开源的物体级 SLAM
Ok K, Liu K,Frey K, et al. RobustObject-based SLAM for High-speed Autonomous Navigation[C]//2019International Conference on Robotics and Automation (ICRA). IEEE, 2019:669-675.
Li J, Meger D,Dudek G. SemanticMapping for View-Invariant Relocalization[C]//2019International Conference on Robotics and Automation (ICRA). IEEE, 2019:7108-7115.
Nicholson L,Milford M, Sünderhauf N. Quadricslam:Dual quadrics from object detections as landmarks in object-oriented slam[J]. IEEERobotics and Automation Letters, 2018, 4(1): 1-8.
四、VIO / VISLAM(10 项)
在传感器融合方面只关注了视觉 + 惯导,其他传感器像 LiDAR,GPS 关注较少(SLAM 太复杂啦 -_-! )。视惯融合的新工作也相对较少,基本一些经典的方案就够用了。
45. msckf_vio
论文:Sun K, Mohta K, Pfrommer B, et al. Robust stereovisual inertial odometry for fast autonomous flight[J]. IEEERobotics and Automation Letters, 2018, 3(2): 965-972.
代码:https://github.com/KumarRobotics/msckf_vio
46. rovio
论文:Bloesch M, Omari S, Hutter M, et al. Robust visual inertial odometry using a direct EKF-basedapproach[C]//2015 IEEE/RSJ international conference onintelligent robots and systems (IROS). IEEE, 2015: 298-304.
代码:https://github.com/ethz-asl/rovio
47. R-VIO
论文:Huai Z, Huang G. Robocentricvisual-inertial odometry[C]//2018 IEEE/RSJ InternationalConference on Intelligent Robots and Systems (IROS). IEEE, 2018:6319-6326.
代码:https://github.com/rpng/R-VIO
48. okvis
论文:Leutenegger S, Lynen S, Bosse M, et al. Keyframe-based visual–inertial odometry using nonlinearoptimization[J]. The International Journal of Robotics Research, 2015,34(3): 314-334.
代码:https://github.com/ethz-asl/okvis
49. VIORB
论文:Mur-Artal R, Tardós J D. Visual-inertialmonocular SLAM with map reuse[J]. IEEE Robotics and AutomationLetters, 2017, 2(2): 796-803.
代码:https://github.com/jingpang/LearnVIORB(VIORB 本身是没有开源的,这是王京大佬复现的一个版本)
50. VINS-mono
论文:Qin T, Li P, Shen S. Vins-mono: Arobust and versatile monocular visual-inertial state estimator[J]. IEEETransactions on Robotics, 2018, 34(4): 1004-1020.
代码:https://github.com/HKUST-Aerial-Robotics/VINS-Mono
双目版 VINS-Fusion:https://github.com/HKUST-Aerial-Robotics/VINS-Fusion
移动段 VINS-mobile:https://github.com/HKUST-Aerial-Robotics/VINS-Mobile
51. VINS-RGBD
论文:Shan Z, Li R, Schwertfeger S. RGBD-InertialTrajectory Estimation and Mapping for Ground Robots[J]. Sensors, 2019,19(10): 2251.
代码:https://github.com/STAR-Center/VINS-RGBD
52. Open-VINS
论文:Geneva P, Eckenhoff K, Lee W, et al. Openvins: A research platform for visual-inertialestimation[C]//IROS 2019 Workshop on Visual-Inertial Navigation:Challenges and Applications, Macau, China. IROS 2019.
代码:https://github.com/rpng/open_vins
53. versavis(多功能的视惯传感器系统)
论文:Tschopp F, RinerM, Fehr M, et al. VersaVIS—AnOpen Versatile Multi-Camera Visual-Inertial Sensor Suite[J]. Sensors, 2020,20(5): 1439.
代码:https://github.com/ethz-asl/versavis
54. CPI(视惯融合的封闭式预积分)
论文:Eckenhoff K, Geneva P, Huang G. Closed-form preintegration methods for graph-basedvisual–inertial navigation[J]. The International Journal ofRobotics Research, 2018.
代码:https://github.com/rpng/cpi
五、Dynamic SLAM(5 项)
动态 SLAM 也是一个很值得研究的话题,这里不太好分类,很多工作用到了语义信息或者用来三维重建,收集的方案相对较少,欢迎补充issue。
55. DynamicSemanticMapping(动态语义建图)
论文:Kochanov D, Ošep A, Stückler J, et al. Scene flow propagation for semantic mapping and objectdiscovery in dynamic street scenes[C]//Intelligent Robots and Systems(IROS), 2016 IEEE/RSJ International Conference on. IEEE, 2016:1785-1792.
代码:https://github.com/ganlumomo/DynamicSemanticMapping
56. DS-SLAM(动态语义 SLAM)
论文:Yu C, Liu Z, Liu X J, et al. DS-SLAM: Asemantic visual SLAM towards dynamic environments[C]//2018IEEE/RSJ International Conference on Intelligent Robots and Systems (IROS).IEEE, 2018: 1168-1174.
代码:https://github.com/ivipsourcecode/DS-SLAM
57. Co-Fusion(实时分割与跟踪多物体)
论文:Rünz M, Agapito L. Co-fusion:Real-time segmentation, tracking and fusion of multiple objects[C]//2017 IEEEInternational Conference on Robotics and Automation (ICRA). IEEE, 2017:4471-4478.
代码:https://github.com/martinruenz/co-fusion
58. DynamicFusion
论文:Newcombe R A, Fox D, Seitz S M. Dynamicfusion: Reconstruction and tracking of non-rigidscenes in real-time[C]//Proceedings of the IEEE conference oncomputer vision and pattern recognition. 2015: 343-352.
代码:https://github.com/mihaibujanca/dynamicfusion
59. ReFusion(动态场景利用残差三维重建)
论文:Palazzolo E, Behley J, Lottes P, et al. ReFusion: 3DReconstruction in Dynamic Environments for RGB-D Cameras Exploiting Residuals[J]. arXivpreprint arXiv:1905.02082, 2019.
代码:https://github.com/PRBonn/refusion
六、Mapping(18 项)
针对建图的工作一方面是利用几何信息进行稠密重建,另一方面很多工作利用语义信息达到了很好的语义重建效果,三维重建本身就是个很大的话题,开源代码也很多,以下方案收集地可能也不太全。
60. InfiniTAM(跨平台 CPU 实时重建)
论文:Prisacariu V A,Kähler O, Golodetz S, et al. Infinitam v3: A framework for large-scale 3dreconstruction with loop closure[J]. arXiv preprint arXiv:1708.00783, 2017.
代码:https://github.com/victorprad/InfiniTAM
61. BundleFusion
论文:Dai A, Nießner M, Zollhöfer M, et al. Bundlefusion:Real-time globally consistent 3d reconstruction using on-the-fly surfacereintegration[J]. ACM Transactions on Graphics (TOG), 2017,36(4): 76a.
代码:https://github.com/niessner/BundleFusion
62. KinectFusion
论文:Newcombe R A, Izadi S, Hilliges O, et al. KinectFusion: Real-time dense surface mapping and tracking[C]//2011 10thIEEE International Symposium on Mixed and Augmented Reality. IEEE, 2011:127-136.
代码:https://github.com/chrdiller/KinectFusionApp
63. ElasticFusion
论文:Whelan T, Salas-Moreno R F, Glocker B, etal. ElasticFusion: Real-time dense SLAM and light sourceestimation[J]. The International Journal of Robotics Research, 2016,35(14): 1697-1716.
代码:https://github.com/mp3guy/ElasticFusion
64. Kintinuous
ElasticFusion 同一个团队的工作,帝国理工 Stefan Leutenegger
论文:Whelan T, Kaess M, Johannsson H, et al. Real-time large-scale dense RGB-D SLAM with volumetricfusion[J]. The International Journal of Robotics Research, 2015,34(4-5): 598-626.
代码:https://github.com/mp3guy/Kintinuous
65. ElasticReconstruction
论文:Choi S, Zhou Q Y, Koltun V. Robust reconstruction of indoor scenes[C]//Proceedingsof the IEEE Conference on Computer Vision and Pattern Recognition. 2015:5556-5565.
代码:https://github.com/qianyizh/ElasticReconstruction
66. FlashFusion
论文:Han L, Fang L. FlashFusion:Real-time Globally Consistent Dense 3D Reconstruction using CPU Computing[C]. RSS, 2018.
代码(一直没放出来):https://github.com/lhanaf/FlashFusion
67. RTAB-Map(激光视觉稠密重建)
论文:Labbé M, Michaud F. RTAB‐Map as an open‐source lidar and visual simultaneouslocalization and mapping library for large‐scale and long‐term online operation[J]. Journal ofField Robotics, 2019, 36(2): 416-446.
代码:https://github.com/introlab/rtabmap
68. RobustPCLReconstruction(户外稠密重建)
论文:Lan Z, Yew Z J, Lee G H. Robust Point Cloud Based Reconstruction of Large-ScaleOutdoor Scenes[C]//Proceedings of the IEEE Conference on ComputerVision and Pattern Recognition. 2019: 9690-9698.
代码:https://github.com/ziquan111/RobustPCLReconstruction
69. plane-opt-rgbd(室内平面重建)
论文:Wang C, Guo X. Efficient Plane-Based Optimization of Geometry and Texturefor Indoor RGB-D Reconstruction[C]//Proceedings of the IEEE Conferenceon Computer Vision and Pattern Recognition Workshops. 2019: 49-53.
代码:https://github.com/chaowang15/plane-opt-rgbd
70. DenseSurfelMapping(稠密表面重建)
论文:Wang K, Gao F, Shen S. Real-timescalable dense surfel mapping[C]//2019 International Conference onRobotics and Automation (ICRA). IEEE, 2019: 6919-6925.
代码:https://github.com/HKUST-Aerial-Robotics/DenseSurfelMapping
71. surfelmeshing(网格重建)
论文:Schöps T, Sattler T, Pollefeys M. Surfelmeshing:Online surfel-based mesh reconstruction[J]. IEEE Transactions on PatternAnalysis and Machine Intelligence, 2019.
代码:https://github.com/puzzlepaint/surfelmeshing
72. DPPTAM(单目稠密重建)
论文:Concha Belenguer A, Civera Sancho J. DPPTAM: Dense piecewise planar tracking and mapping from amonocular sequence[C]//Proc. IEEE/RSJ Int. Conf. Intell. Rob. Syst. 2015(ART-2015-92153).
代码:https://github.com/alejocb/dpptam
相关研究:基于超像素的单目 SLAM:UsingSuperpixels in Monocular SLAM ICRA 2014 ;谷歌学术
73. VI-MEAN(单目视惯稠密重建)
论文:Yang Z, Gao F, Shen S. Real-time monocular dense mapping on aerial robots usingvisual-inertial fusion[C]//2017 IEEE International Conference onRobotics and Automation (ICRA). IEEE, 2017: 4552-4559.
代码:https://github.com/dvorak0/VI-MEAN
74. REMODE(单目概率稠密重建)
论文:Pizzoli M, Forster C, Scaramuzza D. REMODE: Probabilistic, monocular dense reconstruction inreal time[C]//2014 IEEE International Conference on Robotics andAutomation (ICRA). IEEE, 2014: 2609-2616.
原始开源代码:https://github.com/uzh-rpg/rpg_open_remode
与 ORB-SLAM2 结合版本:https://github.com/ayushgaud/ORB_SLAM2https://github.com/ayushgaud/ORB_SLAM2
75. DeepFactors(实时的概率单目稠密 SLAM)
帝国理工学院戴森机器人实验室
论文:Czarnowski J, Laidlow T, Clark R, et al. DeepFactors:Real-Time Probabilistic Dense Monocular SLAM[J]. arXivpreprint arXiv:2001.05049, 2020.
代码:https://github.com/jczarnowski/DeepFactors(还未放出)
其他论文:Bloesch M,Czarnowski J, Clark R, et al. CodeSLAM—learning a compact, optimisable representationfor dense visual SLAM[C]//Proceedings of the IEEE conference oncomputer vision and pattern recognition. 2018: 2560-2568.
76. probabilistic_mapping(单目概率稠密重建)
港科沈邵劼老师团队
论文:Ling Y, Wang K, Shen S. Probabilisticdense reconstruction from a moving camera[C]//2018IEEE/RSJ International Conference on Intelligent Robots and Systems (IROS).IEEE, 2018: 6364-6371.
代码:https://github.com/ygling2008/probabilistic_mapping
另外一篇稠密重建文章的代码一直没放出来Github:Ling Y, Shen S. Real‐timedense mapping for online processing and navigation[J]. Journal ofField Robotics, 2019, 36(5): 1004-1036.
77. ORB-SLAM2 单目半稠密建图
论文:Mur-Artal R, Tardós J D. Probabilistic Semi-Dense Mapping from Highly AccurateFeature-Based Monocular SLAM[C]//Robotics: Science and Systems. 2015,2015.
代码(本身没有开源,贺博复现的一个版本):https://github.com/HeYijia/ORB_SLAM2
加上线段之后的半稠密建图
论文:He S, Qin X, Zhang Z, et al. Incremental3d line segment extraction from semi-dense slam[C]//2018 24thInternational Conference on Pattern Recognition (ICPR). IEEE, 2018:1658-1663.
代码:https://github.com/shidahe/semidense-lines
作者在此基础上用于指导远程抓取操作的一项工作:https://github.com/atlas-jj/ORB-SLAM-free-space-carving
七、Optimization(6 项)
个人感觉优化可能是 SLAM 中最难的一部分了吧 +_+ ,我们一般都是直接用现成的因子图、图优化方案,要创新可不容易,分享山川小哥d的入坑指南https://zhuanlan.zhihu.com/p/53972892。
78. 后端优化库
GTSAM:https://github.com/borglab/gtsam
g2o:https://github.com/RainerKuemmerle/g2o
ceres:http://ceres-solver.org/
79. ICE-BA
论文:Liu H, Chen M, Zhang G, et al. Ice-ba: Incremental, consistent and efficient bundleadjustment for visual-inertial slam[C]//Proceedings of the IEEE Conferenceon Computer Vision and Pattern Recognition. 2018: 1974-1982.
代码:https://github.com/baidu/ICE-BA
80. minisam(因子图最小二乘优化框架)
论文:Dong J, Lv Z. miniSAM: AFlexible Factor Graph Non-linear Least Squares Optimization Framework[J]. arXivpreprint arXiv:1909.00903, 2019.
代码:https://github.com/dongjing3309/minisam
81. SA-SHAGO(几何基元图优化)
论文:Aloise I, Della Corte B, Nardi F, et al. Systematic Handling of Heterogeneous Geometric Primitivesin Graph-SLAM Optimization[J]. IEEE Robotics and AutomationLetters, 2019, 4(3): 2738-2745.
代码:https://srrg.gitlab.io/sashago-website/index.html#
82. MH-iSAM2(SLAM 优化器)
论文:Hsiao M, Kaess M. MH-iSAM2:Multi-hypothesis iSAM using Bayes Tree and Hypo-tree[C]//2019International Conference on Robotics and Automation (ICRA). IEEE, 2019:1274-1280.
代码:https://bitbucket.org/rpl_cmu/mh-isam2_lib/src/master/
83. MOLA(用于定位和建图的模块化优化框架)
论文:Blanco-Claraco J L. A ModularOptimization Framework for Localization and Mapping[J]. Proc. ofRobotics: Science and Systems (RSS), FreiburgimBreisgau, Germany, 2019,2.
代码:https://github.com/MOLAorg/mola
上述内容,如有侵犯版权,请联系作者,会自行删文。
Recommend
About Joyk
Aggregate valuable and interesting links.
Joyk means Joy of geeK