

Training a Fish Detector with NVIDIA DetectNet (Part 2/2)
source link: https://jkjung-avt.github.io/detectnet-training/
Go to the source link to view the article. You can view the picture content, updated content and better typesetting reading experience. If the link is broken, please click the button below to view the snapshot at that time.
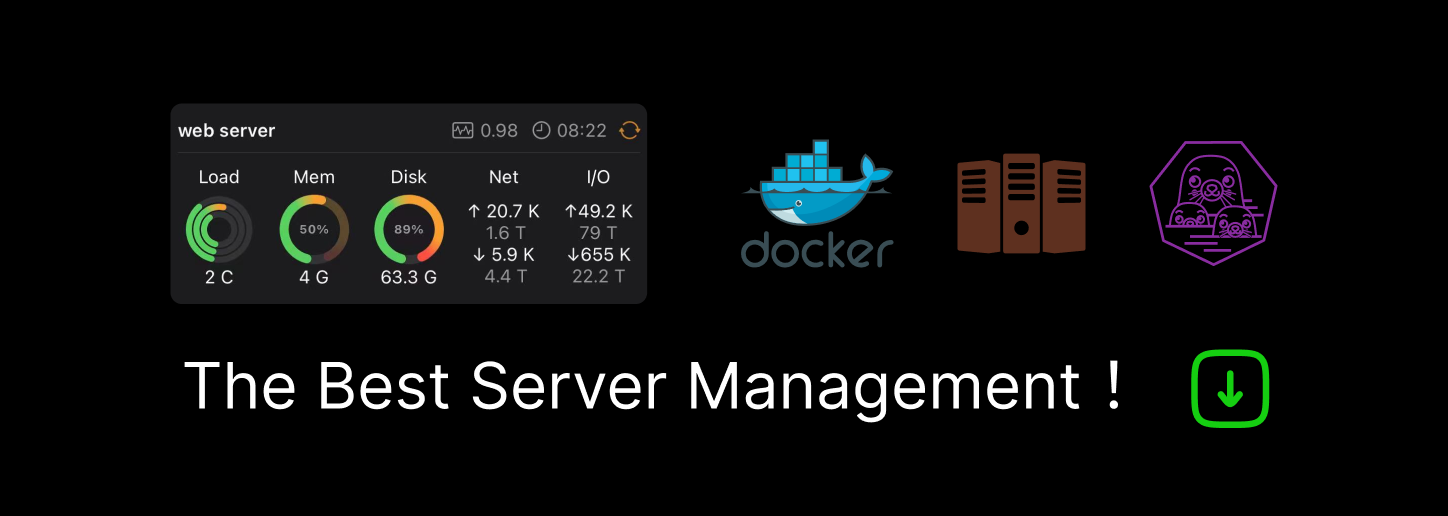
Training a Fish Detector with NVIDIA DetectNet (Part 2/2)
Sep 8, 2017
As described in my previous post, Training a Fish Detector with NVIDIA DetectNet (Part 1/2), I’ve prepared Kaggle Fisheries image data with labels ready for DetectNet training. It’s time to load the data to my DIGITS server and do the training.
Training a DetectNet model with DIGITS is mostly straightforward, except that I had to modify image width and height correctly (1280x720) in the prototxt file (more on this later). I basically followed the Object Detection example (with KITTI dataset) in the NVIDIA/DIGITS GitHub repository.
I first loaded the Object Detection dataset into DIGITS.
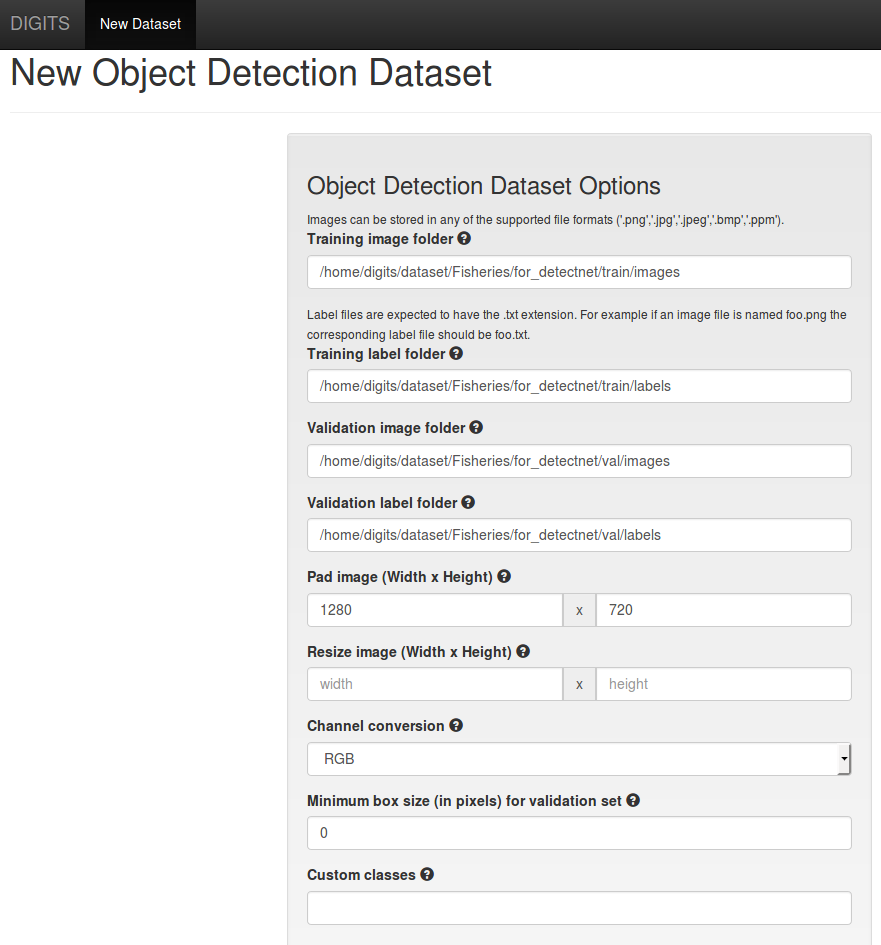
Next I created an Object Detection model to be trained with the dataset. Following the DIGITS Object Detection KITTI example, I set Subtract Mean
to None
, set Solver type
to Adam
, set Base Learning Rate
to 0.0001
with (advanced) Exponential Decay
Policy and 0.95
Gamma value, set Batch size
to 2
and set Batch Accumulation
to 5
. Note that training the DetectNet on a GTX-1080 with 8GB memory, I was only able to fit at most 2
1080x720 input images as a batch to the GPU.
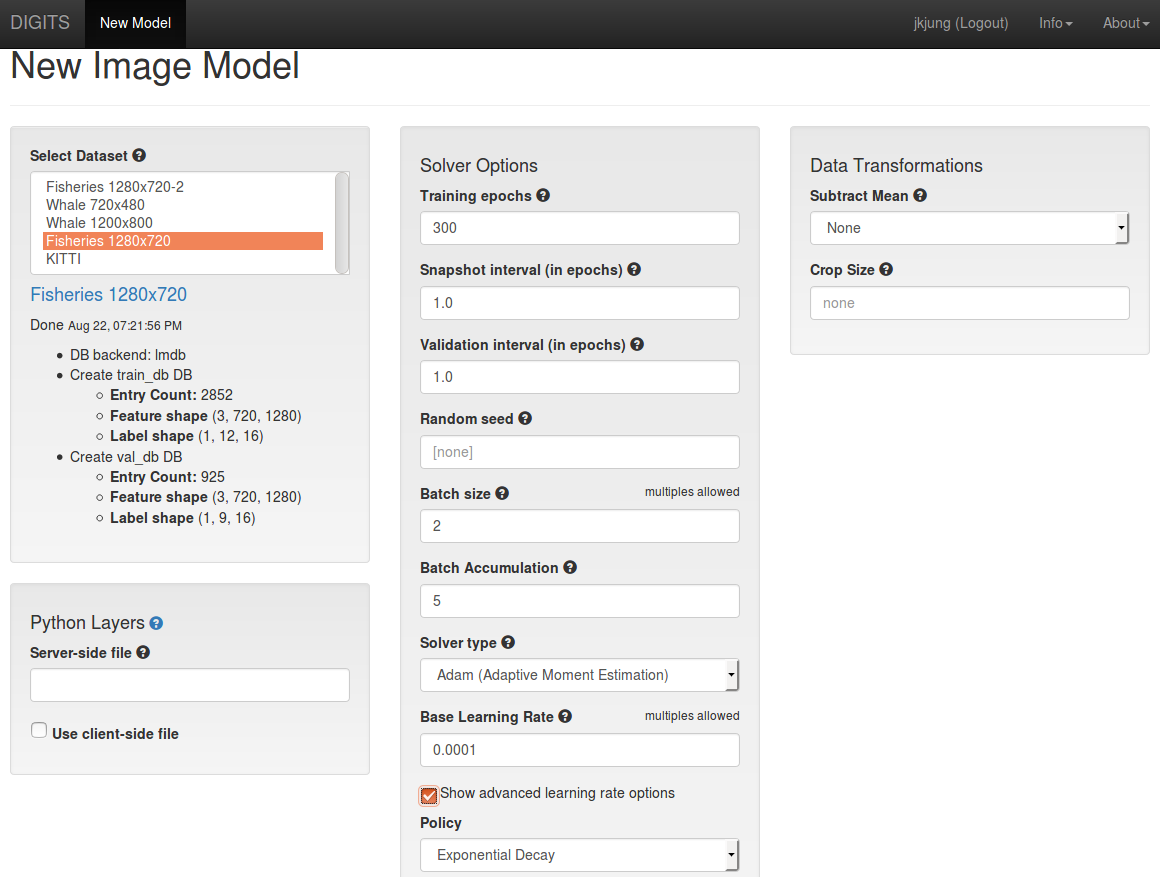
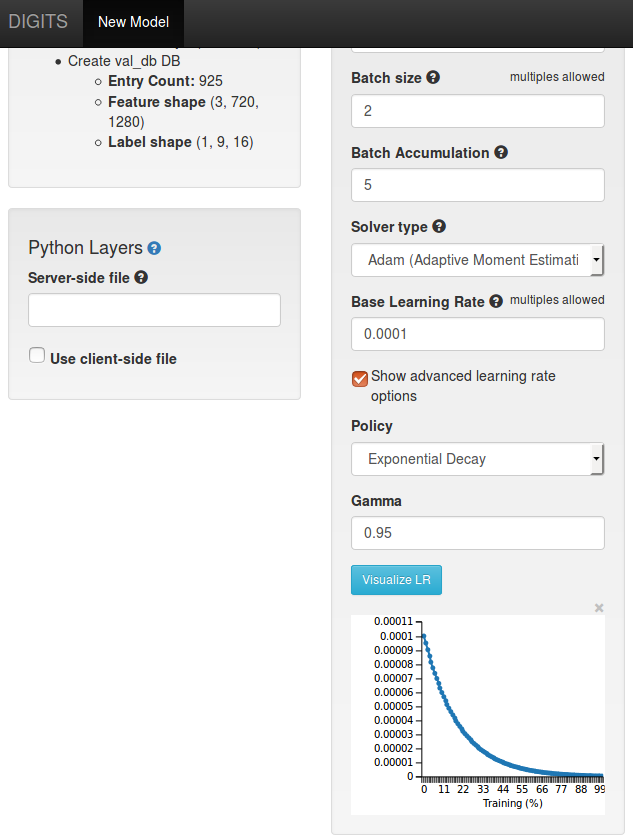
I then copied and pasted the example detectnet_network.prototxt as my Custom Network
. And I did 2 important modifications here.
- I modified all image sizes from 1248x352 (or 1248x384) to 1280x720 in the prototxt. There are 6 occurences in total. Refer to the screenshot below for 2 of such occurences.
- I used a caffemodel (DNN weights) which had been pre-trained with KITTI dataset. With this transfer learning trick, I think the network should be able to learn faster.
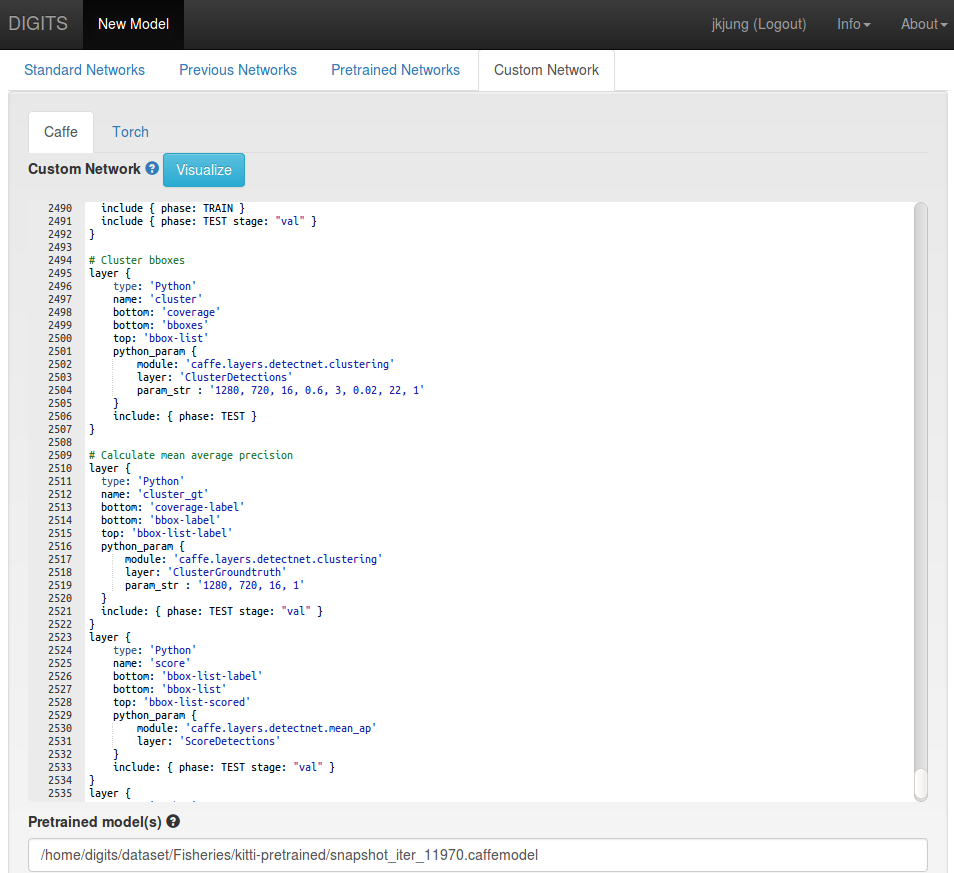
I trained the DetectNet model for 300 epochs in the first round. As a result I got a model with validation precision 75.3%, recall 76.0% and mAP 64.4. (By the way, training this model for 300 epochs on my GTX-1080 desktop PC took roughly 21 hours.)
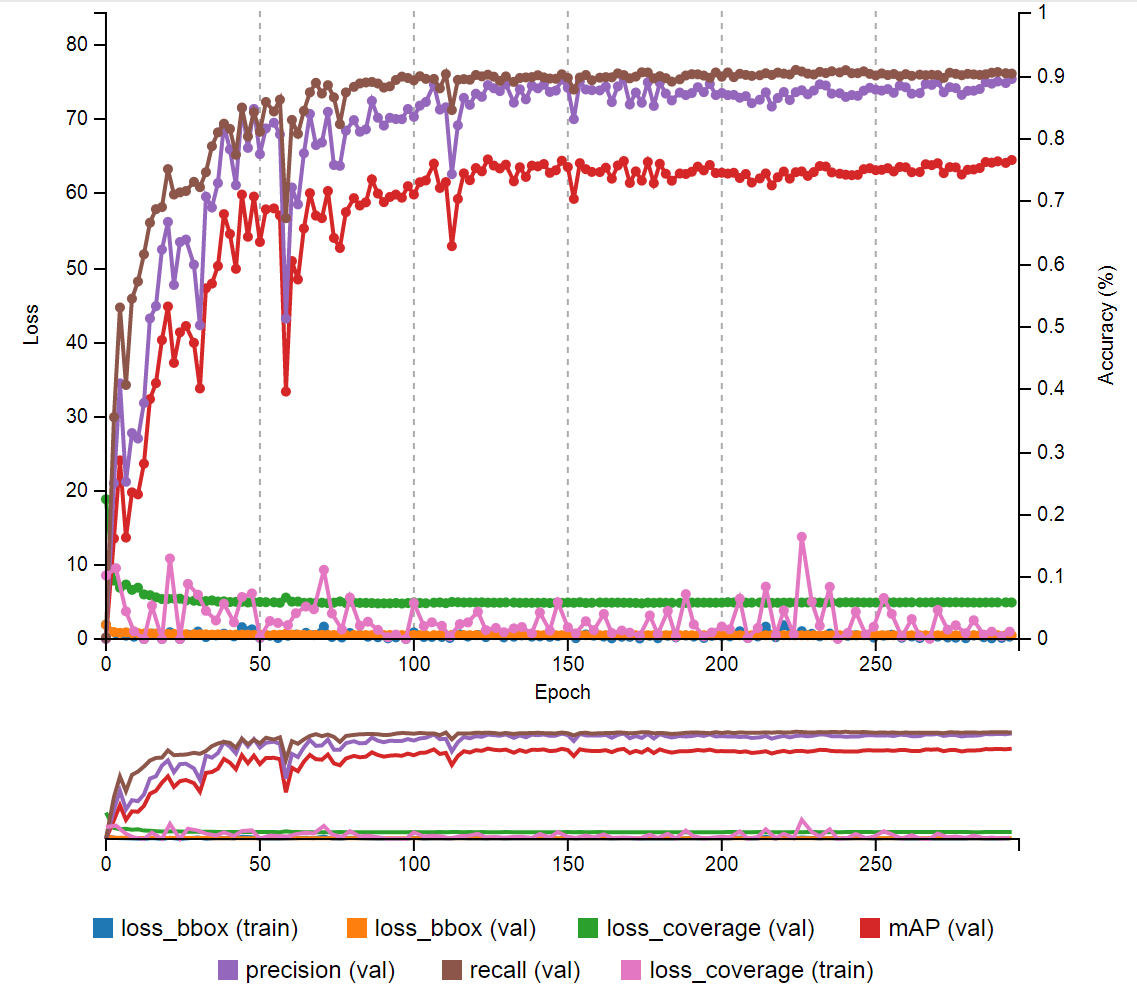
I took the result of the first round, fine-tuned a few parameters, lowering the learning rate a little bit, and trained the model again for 300 epochs. The result improved a little bit. In the end I had a DetectNet model with validation precision 86.77%, recall 87.12% and mAP 78.6.
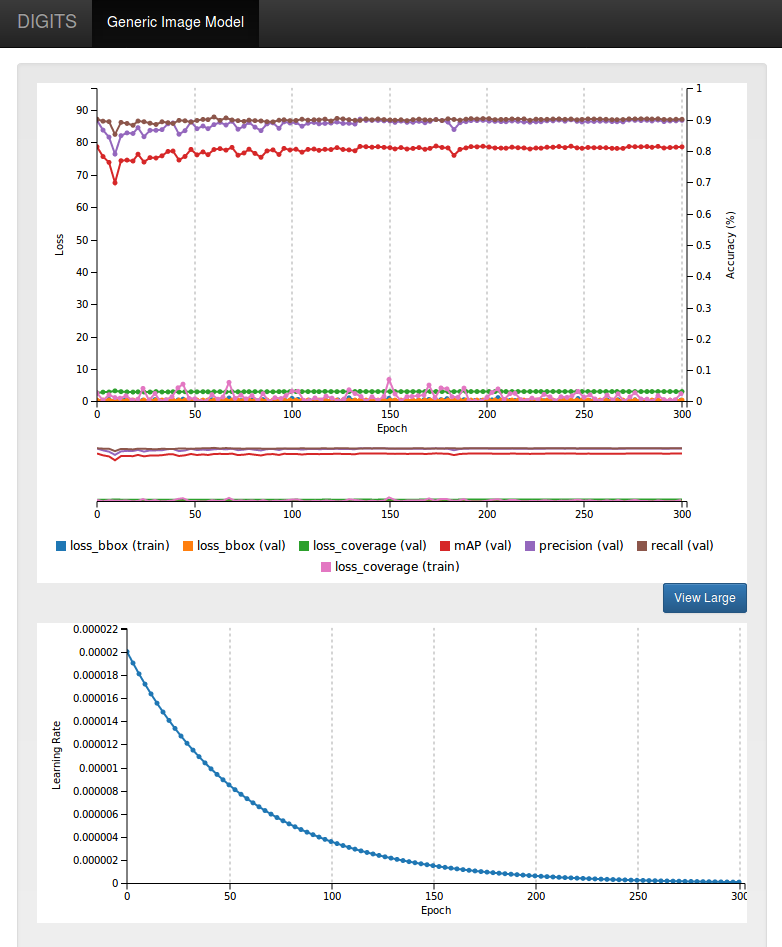
Finally I tested my trained DetectNet model with the Test Many
function in DIGITS. I could see that the model indeed had about 80% accuracy in detecting fishes on newly unseen test images (from test_stg1.zip).
Here is an example for which the model made a correct prediction.
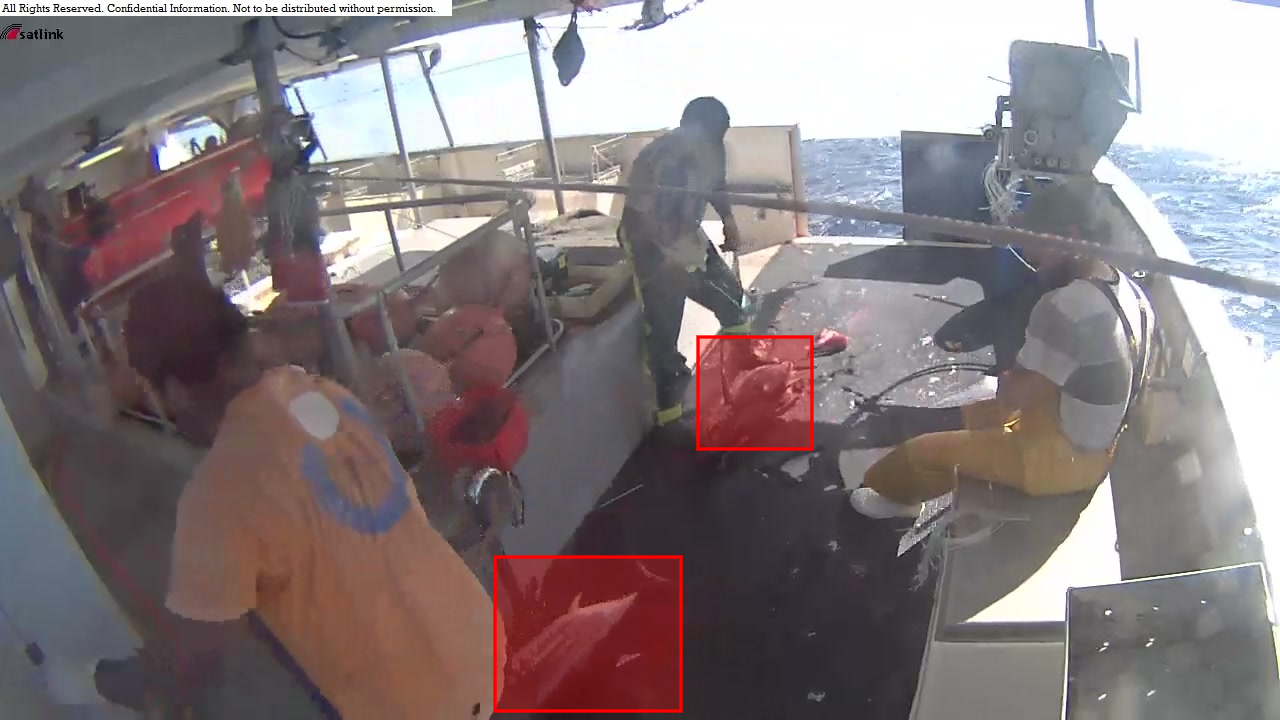
And here is an example for which the model had clearly missed a fish.
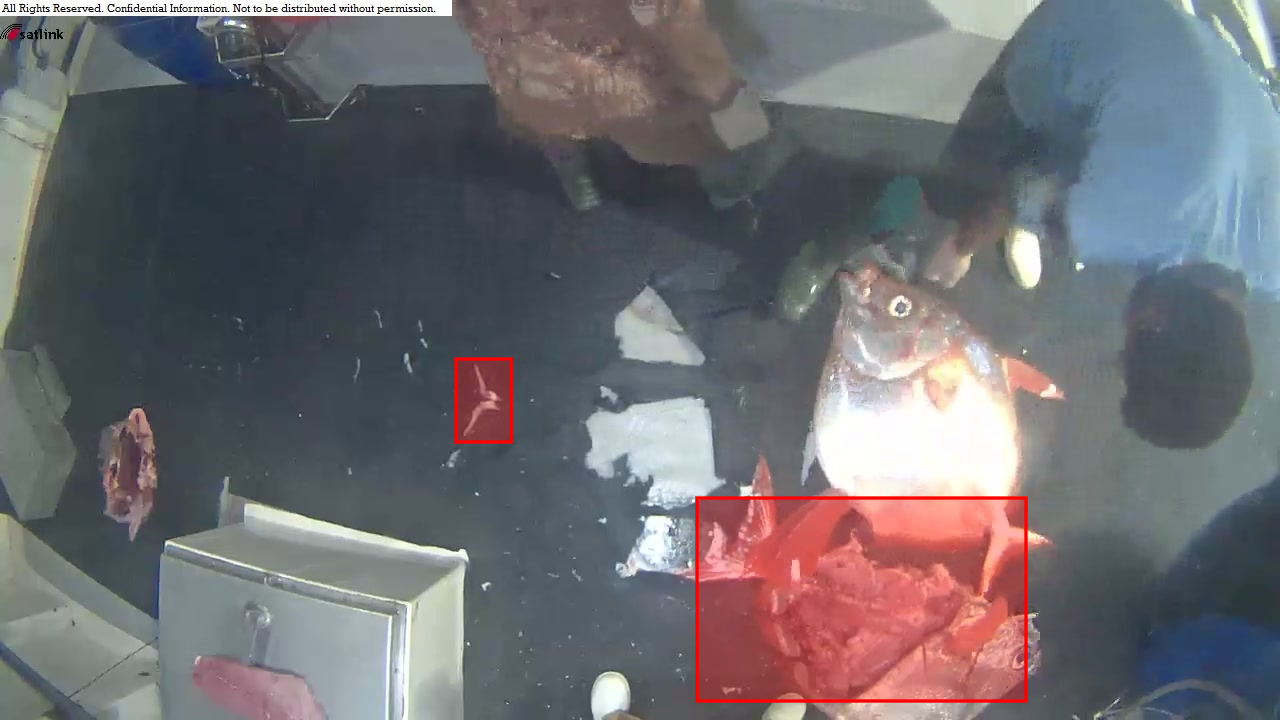
Finally, I tried to deploy the fish detector onto Jetson TX2. I downloaded the final (epoch #300) network snapshot from DIGITS, and copied the files onto Jetson TX2. I had to manually remove the last Python layer in the deploy.protxt
. I also modified detectnet-camera/detectnet-camera.cpp
to use my Logitech C920 USB camera (/dev/video1) as video input. Then I ran the jetson-inference demo code by:
$ cd ~/project/jetson-inference/build/aarch64/bin
$ ./detectnet-camera \
-model /home/nvidia/project/jetson-inference/data/networks/DetectNet-Fisheries/snapshot_iter_88200.caffemodel \
-prototxt /home/nvidia/project/jetson-inference/data/networks/DetectNet-Fisheries/deploy.protxt \
-input_blob data \
-output_cvg coverage \
-output_bbox bboxes
So there I had it: a real-time fish detector on Jetson TX2, which is capable of processing 1280x720 images at 7.6 frames per second…
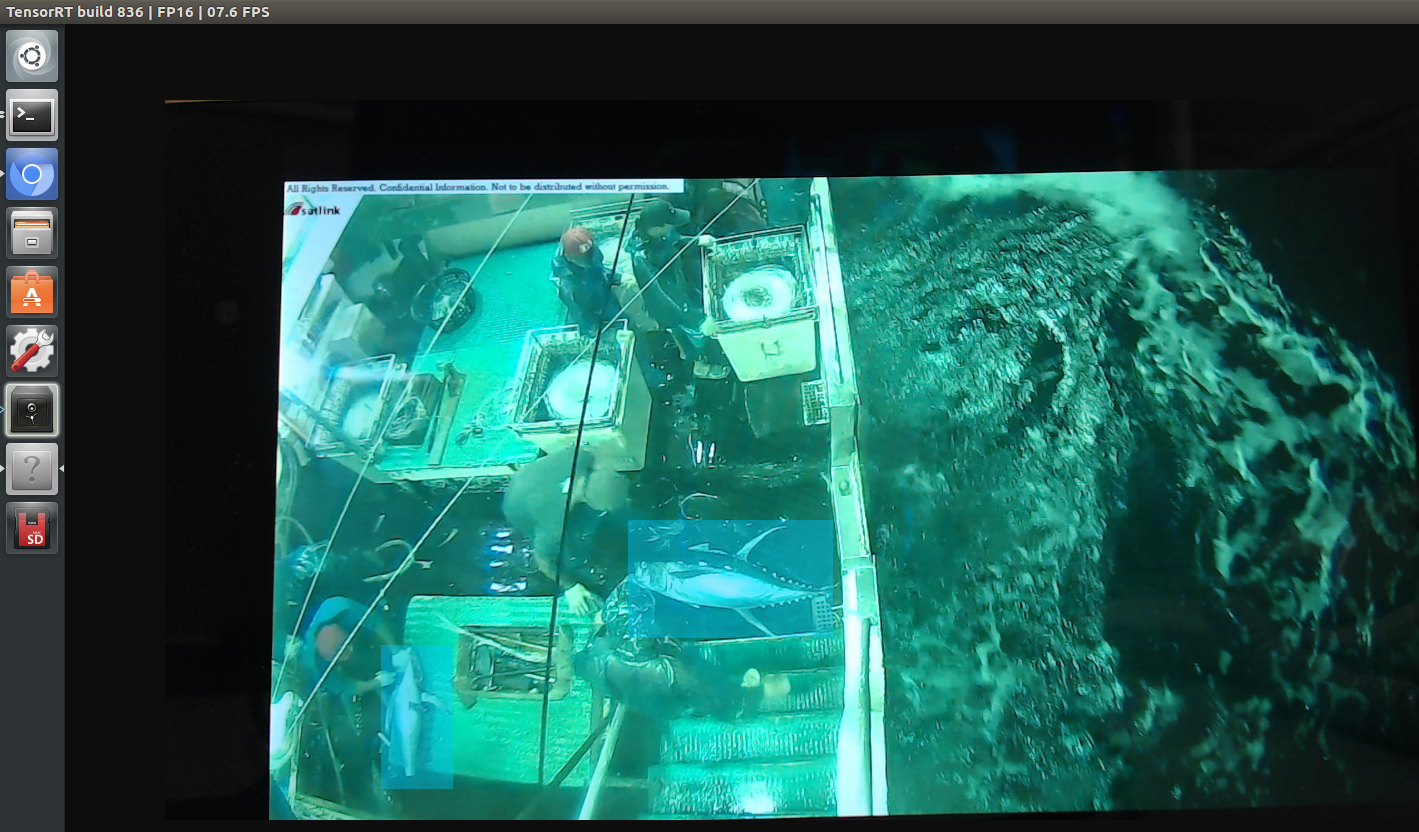
Recommend
About Joyk
Aggregate valuable and interesting links.
Joyk means Joy of geeK