

实现 MNN 模型的可视化工具
source link: http://satanwoo.github.io/2020/02/06/MNN-Visual/
Go to the source link to view the article. You can view the picture content, updated content and better typesetting reading experience. If the link is broken, please click the button below to view the snapshot at that time.
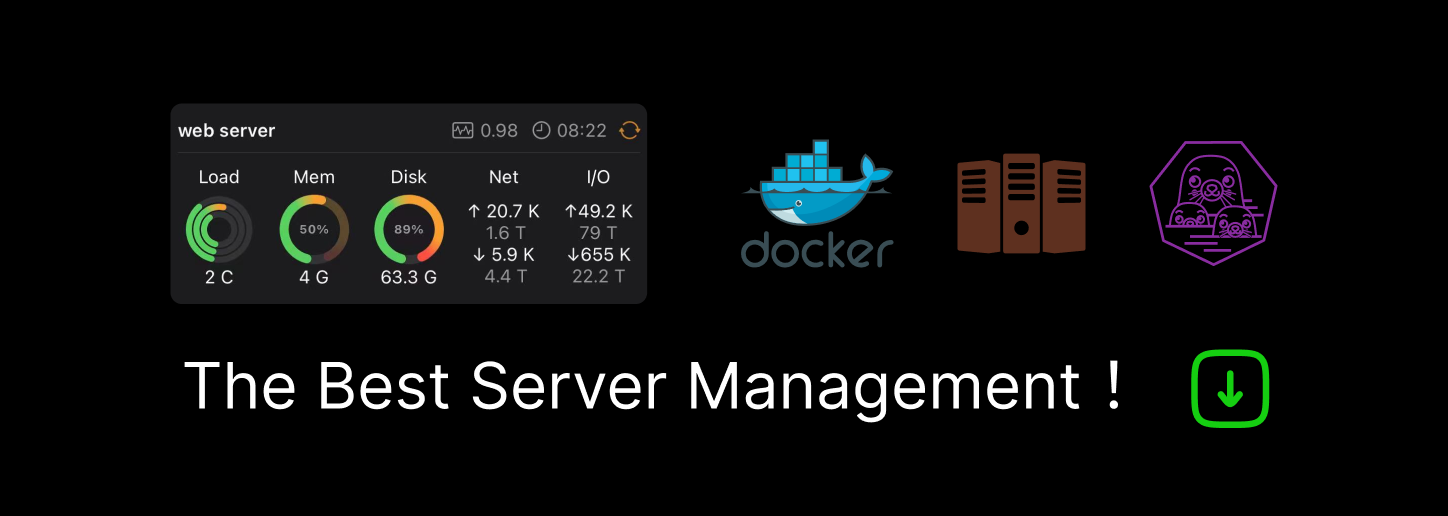
实现 MNN 模型的可视化工具
Netron
是一个支持 Tensorflow
,PyTorch
,MXNet
,NCNN
, PaddlePaddle
等深度模型格式的可视化框架。去年国庆前的时候我稍微研究了下相关的代码,重点关注其将其是如何设计出一套兼容不同模型格式表征,用来归一化展现不同的深度学习框架模型。
研究完成后,我利用如下两个 Commit
作为 Pull Request
提交给了作者,用以支持 MNN
的模型可视化。
从中也不难看出我扎实的英语表述能力(我果然是个国际化人才)。
这篇文章会从架构设计、标准定义、巧用JS解析等几个方面来阐述
整体上,按照我个人的理解,Netron
的架构可以简要展现如下:
最基础的应用部分及运行环境,是 Electron
这个跨平台框架直接呈现的。
当然,一些诸如基础zip/gzip用于解压等等的库我们也统一归类到支撑里。
然后是一套经典的 MVC
的结构,app.js
作为整体的 controller ,负责整个应用的功能逻辑,如导出图片、菜单管理、保存加载等等。这一层我们需要的做事非常少,只要将 MNN
支持的模型后缀 .mnn
注册进去即可。 然后是是对应的 view.js
,这块实际上还是一层 controller
,类比我们常说的子控制器,专门用于处理主视图的逻辑,如下图所示:
从这块开始,我们就要注意了,因为这里开始通过工厂方法对应的根据读取文件类型的不同,托管给了不同的自定义 xxx.js
来处理后续步骤。 比如.mar
,model
,prototxt
等格式的模型会首先托管给 mxnet.js
来处理。如果存在重名,则按照先后顺序依次尝试。
view.ModelFactoryService = class {
constructor(host) {
this._host = host;
this._extensions = [];
this.register('./onnx', [ '.onnx', '.pb', '.pbtxt', '.prototxt' ]);
this.register('./mxnet', [ '.mar', '.model', '.json', '.params' ]);
this.register('./keras', [ '.h5', '.hd5', '.hdf5', '.keras', '.json', '.model' ]);
this.register('./coreml', [ '.mlmodel' ]);
this.register('./caffe', [ '.caffemodel', '.pbtxt', '.prototxt', '.pt' ]);
this.register('./caffe2', [ '.pb', '.pbtxt', '.prototxt' ]);
this.register('./pytorch', [ '.pt', '.pth', '.pkl', '.h5', '.t7', '.model', '.dms', '.pth.tar', '.ckpt', '.bin' ]);
this.register('./torch', [ '.t7' ]);
this.register('./torchscript', [ '.pt', '.pth' ]);
this.register('./mnn', ['.mnn', '.tflite']);
this.register('./tflite', [ '.tflite', '.lite', '.tfl', '.bin' ]);
this.register('./tf', [ '.pb', '.meta', '.pbtxt', '.prototxt', '.json' ]);
this.register('./sklearn', [ '.pkl', '.joblib', '.model' ]);
this.register('./cntk', [ '.model', '.cntk', '.cmf', '.dnn' ]);
this.register('./openvino', [ '.xml' ]);
this.register('./darknet', [ '.cfg' ]);
this.register('./paddle', [ '.paddle', '__model__' ]);
this.register('./ncnn', [ '.param', '.bin', '.cfg.ncnn', '.weights.ncnn']);
this.register('./dl4j', [ '.zip' ]);
this.register('./mlnet', [ '.zip']);
}
在这上层是一层标准定义层,用于抹平不同模型之间的表达方式,用归一化的逻辑来进行处理,至于怎么把自己的模型表征映射成归一化的逻辑,就需要编写对应 xxx.js
来自行处理,后文会以 MNN
来进行举例。
最上层就是对应各个深度框架自行的逻辑处理了。其中包含了数据格式及对应解析(如 flatbuffer
)、内容校验、构图等等,后文也会用 MNN
举例说明。
这一环是一个很不起眼但是却非常重要的环节。 每种深度模型框架都有其自定义的模块结构和模块构成,一般都以 Flatbuffer Schema
的形式构成。(当然也有例外)以MNN
为例,其对应的模型结构大致如下图所示:
同理, TFLite
的模型也可见 TFLite.schema
,不再赘述。
从定义中不难看出,TFLite
有 model
,graph
,SubGraph
等;而 MNN
对应的就是Net
;再往下一层 TFLite
有 Operator
和 Options
;而 MNN
有 OP
和OPParameter
;至于 NCNN
则是 Layer
。
如果是从整个架构角度去兼容不同的框架,必然会有着大量的 messy code
。因此作者定义了一套标准表征,让不同的深度模型自己去解析,然后附着自身的逻辑到这同一套表征上。
Model
,表示模型的静态表示。Graph
,表示模型的计算图表示。Node
,一个操作对应一个节点。Tensor
,输入输出数据。Parameter
,对应的属性。Argument
,对应的属性值。
上述
Parameter
和Argument
可以简单认为一一对应吧,都认为是属性值即可。
一图胜千言,下图比较好的展现了术语和对应的表征:
这样不同的框架模型只要在自己对应的 xxx.js
中,把图,OP
层对应的数据填充至对应的地方即可。
这里依然以 MNN
举例:
- 我们不存在
subgraph
的概念,直接把Model
和Graph
等价于一个net
即可。 - 从
net
中取出oplist
,对应创建成Node
。 - 从
oplist
中每个op
,取出对应的tensorIndex
,根据net
的tensorName
和tensorIndex
来创建对应的tensor
。 - 从
op
中根据opparameter
的种类,从op.main
中取出不同的数据来填入paramter / argument
,这块是解析的大头,如果没想好方式,就会非常浪费时间,下文重点说。
诸如 MNN
,TFlite
都选用了 Flatbuffer
来进行数据的保存,而官方的 flatc
程序支持直接根据定义的 schema
文件生成对应的 generated.js
,命令如下:
./flatc -s ~/yourPathTo/MNN/schema/default/Type.fbs
这个我看了下很多的同学的在处理多 Schema
定义的时候是对应的一个个生成 generated.js
,这样维护成本比较大,既然我们的已经使用了 include
机制,我们直接在生成过程中合并即可,如下所示:
./flatc --js -I ~/yourPathTo/MNN/schema/default/ ~/yourPathTo/MNN/schema/default/MNN.fbs --gen-all
这里有两个参数注意下:
-I
,表示include
从哪个路径进行搜索。--gen-all
,表示自动对生成的所有文件合并。
生成代码大致如下:
/**
* @param {number} i
* @param {flatbuffers.ByteBuffer} bb
* @returns {MNN.Blob}
*/
MNN.Blob.prototype.__init = function(i, bb) {
this.bb_pos = i;
this.bb = bb;
return this;
};
/**
* @param {flatbuffers.ByteBuffer} bb
* @param {MNN.Blob=} obj
* @returns {MNN.Blob}
*/
MNN.Blob.getRootAsBlob = function(bb, obj) {
return (obj || new MNN.Blob).__init(bb.readInt32(bb.position()) + bb.position(), bb);
};
/**
* @param {flatbuffers.ByteBuffer} bb
* @param {MNN.Blob=} obj
* @returns {MNN.Blob}
*/
MNN.Blob.getSizePrefixedRootAsBlob = function(bb, obj) {
return (obj || new MNN.Blob).__init(bb.readInt32(bb.position()) + bb.position(), bb);
};
具体关于 FlatBuffer
的细节,可以阅读我之前的文章,不再赘述。
避免冗余解析流程
上文提到 根据 OpParameter 来获取 main
中的数据,然后依次填入 parameter / argument
是比较耗费精力的步骤。我们所有的 OpParameter
类型有 74种(还在不断更新)
MNN.OpParameter = {
NONE: 0,
QuantizedAdd: 1,
ArgMax: 2,
AsString: 3,
Axis: 4,
BatchNorm: 5,
BinaryOp: 6,
Blob: 7,
CastParam: 8,
Convolution2D: 9,
Crop: 10,
CropAndResize: 11,
Dequantize: 12,
DetectionOutput: 13,
Eltwise: 14,
ExpandDims: 15,
Fill: 16,
Flatten: 17,
Gather: 18,
GatherV2: 19,
InnerProduct: 20,
Input: 21,
Interp: 22,
LRN: 23,
LSTM: 24,
MatMul: 25,
NonMaxSuppressionV2: 26,
Normalize: 27,
PackParam: 28,
Permute: 29,
Plugin: 30,
Pool: 31,
PRelu: 32,
PriorBox: 33,
Proposal: 34,
QuantizedAvgPool: 35,
QuantizedBiasAdd: 36,
QuantizedConcat: 37,
QuantizedLogistic: 38,
QuantizedMatMul: 39,
QuantizedMaxPool: 40,
QuantizedRelu: 41,
QuantizedRelu6: 42,
QuantizedReshape: 43,
QuantizedSoftmax: 44,
QuantizeMaxMin: 45,
QuantizeV2: 46,
Range: 47,
Rank: 48,
ReduceJoin: 49,
ReductionParam: 50,
Relu: 51,
Relu6: 52,
RequantizationRange: 53,
Requantize: 54,
Reshape: 55,
Resize: 56,
RoiPooling: 57,
Scale: 58,
Selu: 59,
Size: 60,
Slice: 61,
SliceTf: 62,
SpaceBatch: 63,
SqueezeParam: 64,
StridedSliceParam: 65,
TensorConvertInfo: 66,
TfQuantizedConv2D: 67,
TopKV2: 68,
Transpose: 69,
UnaryOp: 70,
MomentsParam: 71,
RNNParam: 72,
BatchMatMulParam: 73,
QuantizedFloatParam: 74
};
以 Convolution2D
举例,它又有几个对应的参数:weight
,bias
,quanParameter
,symmetricQuan
,padX
,padY
,kernelX
,kernelY
等等,需要解析。
一开始我采用了人肉的解析方式,代码就成了 if else
加上一大堆解析代码:
mnn_private.Convolution2DAttrBuilder = class {
constructor() {}
buildAttributes(metadata, parameter) {
//var common = parameter.common();
var attributes = [];
var common = parameter.common();
attributes.push(new mnn.Attribute(metadata, "padX", common.padX(), true));
attributes.push(new mnn.Attribute(metadata, "padY", common.padY(), true));
attributes.push(new mnn.Attribute(metadata, "kernelX", common.kernelX(), true));
attributes.push(new mnn.Attribute(metadata, "kernelY", common.kernelY(), true));
attributes.push(new mnn.Attribute(metadata, "strideX", common.strideX(), true));
attributes.push(new mnn.Attribute(metadata, "strideY", common.strideY(), true));
attributes.push(new mnn.Attribute(metadata, "dilateX", common.dilateX(), true));
attributes.push(new mnn.Attribute(metadata, "dilateY", common.dilateY(), true));
attributes.push(new mnn.Attribute(metadata, "padMode", mnn.schema.PadModeName[common.dilateY()], true));
attributes.push(new mnn.Attribute(metadata, "group", common.group(), true));
attributes.push(new mnn.Attribute(metadata, "outputCount", common.outputCount(), true));
attributes.push(new mnn.Attribute(metadata, "inputCount", common.inputCount(), true));
attributes.push(new mnn.Attribute(metadata, "relu", common.relu(), true));
attributes.push(new mnn.Attribute(metadata, "relu6", common.relu6(), true));
//var quanParameter = parameter.quanParameter();
var weights = [];
for (var w = 0; w < parameter.weightLength(); w++) {
weights.push(parameter.weight(w));
}
attributes.push(new mnn.Attribute(metadata, "weights", weights, true));
var bias = [];
for (var b = 0; b < parameter.biasLength(); b++) {
bias.push(parameter.bias(b));
}
attributes.push(new mnn.Attribute(metadata, "bias", bias, true));
return attributes;
}
get hasMain() {
return true;
}
这样的代码如果写完74个 OpParameter
,可维护性和后续的扩展也不够。
我们要巧用 JavaScript
的 Reflect
能力以及属性等于与字符串值属性的特性
_buildAttributes(metadata, op, net, args) {
var opParameter = op.mainType();
var opParameterName = mnn.schema.OpParameterName[opParameter];
// 获取对应的类型
var mainConstructor = mnn.schema[opParameterName];
var opParameterObject = null;
if (typeof mainConstructor === 'function') {
var mainTemplate = Reflect.construct(mainConstructor, []);
opParameterObject = op.main(mainTemplate);
}
this._recursivelyBuildAttributes(metadata, net, opParameterObject, this._attributes);
}
_recursivelyBuildAttributes(metadata, net, opParameterObject, attributeHolders) {
if (!opParameterObject) return;
var attributeName;
var attributeNames = [];
var attributeNamesMap = {};
for (attributeName of Object.keys(Object.getPrototypeOf(opParameterObject))) {
if (attributeName != '__init') {
attributeNames.push(attributeName);
}
attributeNamesMap[attributeName] = true;
}
var attributeArrayNamesMap = {};
for (attributeName of Object.keys(attributeNamesMap)) {
if (attributeNamesMap[attributeName + 'Length']) { attributeArrayNamesMap[attributeName] = true;
attributeNames = attributeNames.filter((item) => item != (attributeName + 'Array') && item != (attributeName + 'Length'));
}
}
for (attributeName of attributeNames) {
if (opParameterObject[attributeName] && typeof opParameterObject[attributeName] == 'function') {
var value = null;
if (attributeArrayNamesMap[attributeName]) {
var array = [];
var length = opParameterObject[attributeName + 'Length']();
//var a = opParameterObject[attributeName + 'Array']();
for (var l = 0; l < length; l++) {
array.push(opParameterObject[attributeName + 'Length'](l));
}
value = array;
}
else {
value = opParameterObject[attributeName]();
if (typeof value === 'object') {
this._recursivelyBuildAttributes(metadata, net, value, attributeHolders);
value = null;
}
}
if (value) {
var attribute = new mnn.Attribute(metadata, attributeName, value);
attributeHolders.push(attribute);
}
}
}
}
区区50多行代码就可以完成所有 OpParamater
及其对应的属性解析。
Recommend
About Joyk
Aggregate valuable and interesting links.
Joyk means Joy of geeK