

A Big Data Imperative: Driving Big Action
source link: https://www.kaushik.net/avinash/big-data-imperative-driving-big-action/
Go to the source link to view the article. You can view the picture content, updated content and better typesetting reading experience. If the link is broken, please click the button below to view the snapshot at that time.
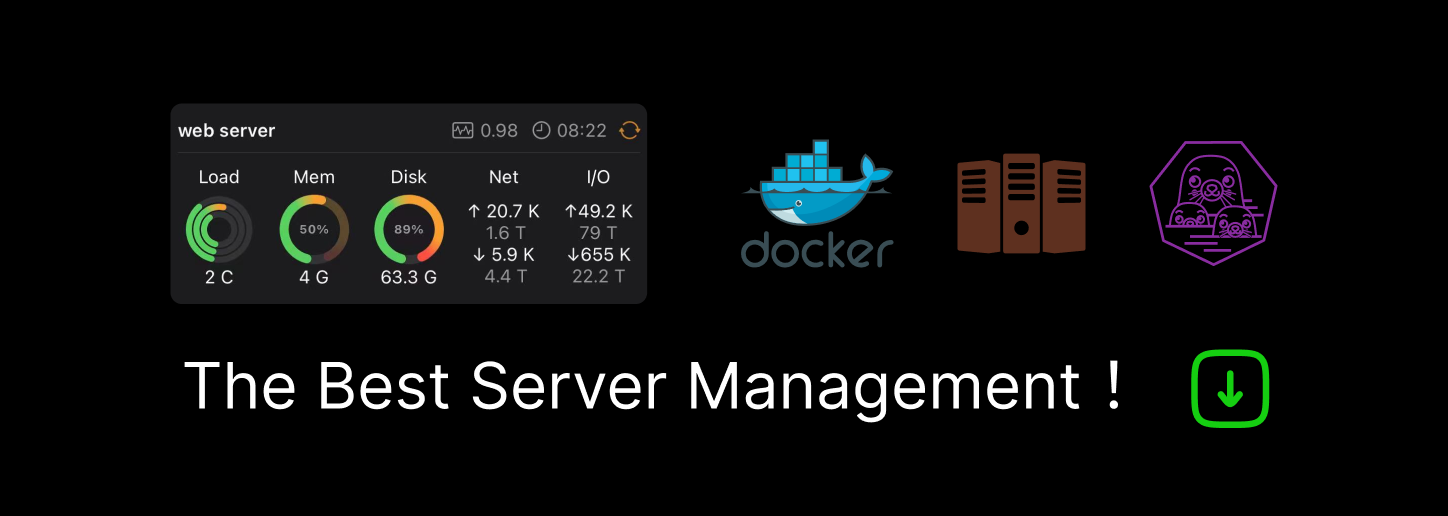
A Big Data Imperative: Driving Big Action
Is there anything in the analytics space that is so full of promise and hype and sexiness and possible awesomeness than "big data?" I don't think so.
So what is big data really? No one quite knows.
As I interpret it, big data is the collection of massive databases of structured and unstructured data. The data sources include traditional (now considered puny) sources like corporate ERP/CRM systems and non-traditional (massive) sources like every technical ping from every human or mechanical sensor, all web behavior by everyone across the entire Internet, increasingly digital data from analog sources like hospitals or the atmosphere, and (good lord!) our collective tweeted wisdom.
That is a lot, right?
Because so much of the big data talk is focused on the promise of zettabytes of data, big data also tends to be about massively parallel computing, fantastic storage systems, the "cloud," Hadoop and MapReduce and other such deeply technical delights.
That explains why so much of big data talk comes from Oracle, IBM, Microsoft, SAP and other vendors. And not so much from practitioners, yet.
I believe in the promise of big data and the awesomeness of the insights that can come from it. But that should not come as a surprise. All the way back in 2007, I was evangelizing the value of moving away from the "small data" world of clickstream data to the "bigger data" world of using multiple data sources to make smarter decisions on the web. Clickstream + qualitative data + rigorous statistical analysis of outcomes + deep mining of data from competitive intelligence sources + rapid experiments + more.
Here's the "bigger web analytics data" picture from 2007… Multiplicity!
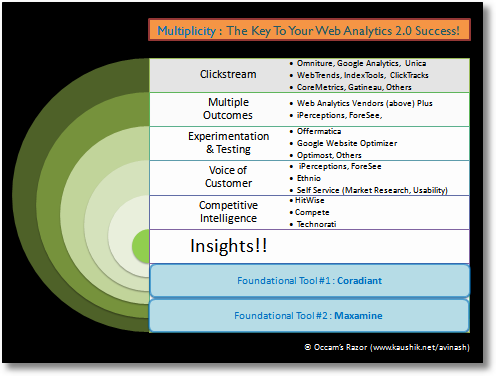
The big data we are dealing with today puts the 2007 picture to shame. We have even more types of data, becoming ever more complex, distributed across multiple existences, and we are left with the task of parsing out terabytes of noise to get to a megabyte of signal.
That last part is what I love to focus on, what I worry about, what I think everyone should focus on. It is great that we have big data. It is greater that we have such amazing promise in that big data. It is sucky that almost no one knows what to do with it in the context of driving actual business value.
Hence my interest in big data is not about the zettabytes or Hadoop or unstructured variables or one of the n technical things that seem to dominate big data conversations.
My interest is deeply and passionately rooted in trying to figure out how to ride big data all the way to the bank (or world peace). How to find insights? How to structure organizations that will use this data to ensure that they get timely value from it? How to drive action? How to find frameworks that force a different type of thinking so we don't make the mistakes we so brilliantly have made in the world of small data?
If we don't answer all those hows big data will be a big disappointment.
Avoiding big disappointment and the hows were on my mind as I prepared my keynote for Strata 2012 Big Data conference . My goal was to take my TED-ish 15-minute timeslot to present my perspective on why driving big action was the big imperative for big data.
It was an incredible challenge, thanks to Strata co-chairs Edd Dumbill and Alistair Croll. In this post, I want to share the result with you.
I'd structured my keynote into three big pieces:
00:00 – 01:15 Intro. My new favorite data quote by Zack Matere, a Kenyan farmer.
01:15 – 04:05 Part 1. The current flawed data org structure, its challenges, and the new optimal org structure to truly bring big action to big data.
04:05 – 06:20 Part 2. A framework, inspired by Donald Rumsfeld, for big data vendors to think about when creating solutions and the unique space in which big data analysts should actually play in (only the "unknown unknowns!").
06:20 – 10:25 Part 3A. My first, tactical, example: How to auto magically solve the problem of having millions of rows of data, and not knowing how to find the 15 valuable rows that could have a huge business impact. Leveraging interestingness!
10:25 – 15:00 Part 3B. My second, strategic, example: Leveraging predict, mine, correlate to shift away from data puking to, even more auto magically, find trends in the data that truly are the unknown unknowns and identify causal factors for those trends so that we can move from data to action at light speed.
Here's the keynote…
[You can also watch this video on YouTube. You're also welcome to Like, Share, Tweet, Facebook, +1 it on YouTube as well.]
It is not my hope to encourage you to copy/paste the strategy outlined, or to use the tools shown.
My hope is to simply inspire you to think a little differently about organization design, share a framework to influence the focus of your analysis, and find the types of practical solutions that will really spark profitability from all this big data.
I welcome your feedback and thoughts on the video and the solutions via comments. Please also share your experience with big data. Any big or small success you've had would be inspiring to all of us.
Preparing for my keynote also got me thinking about all the implications of big data and my own longish career in trying to create superb decision support systems. The database has moved from my floppy disk (true story) to an infinite storage cloud, yet, amazingly, some of the biggest challenges have remained the same.
So big data revolutionaries…
Six Rules That Should Govern Your Big Data Existence.
Here are some rules from my experience in the small data world that I've come to believe also apply to the big data world, perhaps even more so. As you go about your big data journey you'll meet with even more immense success if you consider these valuable life lessons:
1. Don't buy the hype of big data and throw millions of dollars away. But don't stand still.
Take 15% of your decision making budget and give it to one really, really smart person (Ninja! OK, Data Scientist) and give that person the freedom to experiment in the cloud with big data possibilities for your companies.
It is cheap. You can do dirty data warehousing pretty darn fast. You can find all the ugly warts and problems. You can be much smarter when you start to mainstream big data into your company, while preserving the data awesomeness that already exists in your company.
Structure your big data efforts, at least initially, to fail faster while failing forward. Don't build the biggest, baddest big data environment over 32 months, only to realize it was your biggest, baddest mistake.
2. Big thinking about what big data should be solving for is supremely important.
I can't think of any other time in our lives where we could literally swim endlessly in an ocean of data, without having anything to show for it. Big data is that world. If you don't know where you are going, you will get there and you'll be miserable (if your company has not fired you already, in which case you'll be miserable and sad).
I've championed the need to leverage frameworks like the Digital Marketing & Measurement Model, in the web context, to ensure that the analysis we do is deeply and powerfully grounded in what's important to the business. You have to have that one page, even if it is roughly defined by your Sr. Management. Have something.
If your management refuses, or is not visionary enough to provide you with even basic starting points, then build one by yourself. All it takes is a little business analysis. Here's my post: Five Steps to Finding a Purpose for your Analysis.
When you have access to all this data, the answers you find will be surprising, the insights you deliver will be brilliant, and your impact on the business will be huge. But that can only happen if there is a model that defines the purpose of your sweet big data adventures.
3. The 10/90 rule for magnificent data success still holds true.
For every $100 you have available to invest in making smart decisions, invest $10 in tools and vendor services, and invest $90 in big brains (aka people, aka analysis ninjas, aka you!).
I will admit that Oracle and IBM and SAS and solid state drives are very expensive. Nine times that to invest in big brains might seem egregious. Perhaps it is. Let the 10/90 rule be an inspiration to simply over-invest (way over-invest) in people, because without that investment big data will absolutely, positively, be a big disappointment for your company.
Computers and artificial intelligence are simply not there yet. Hence your BFF is natural intelligence. :)
4. Shoot for right time data, not real time data.
Real time data is almost insane to shoot for because even for the smallest decisions, you'll have to do a lot of analysis first (5 hours), then present it to your superior (1 hour), who will add two bullet items and send it to a team of people (20 hours), who will in turn argue about priorities and how much the data is wrong (16 days), but ultimately come to an agreement because the deadline to make the decision passed 7 days ago (20 seconds), and send the data to the big boss who'll read just the first part of the executive summary (3 days), and decide that the data is telling her something counter to what she has always known works, and she'll make a decision based on her gut feel (5 seconds), and some action will be taken (14 days).
Total up those numbers. Was the real time data of any real value?
Ok so that is way over the top. But every company has a complex decision making structure that is time consuming and therefore unable to react in real time. If you can't react in real time, why do you need real time data?
Understand when is the right time for data in your organization. Shoot for systems and processes that match delivery of data (better still, insights ) to that time frame. You'll have less stress. You'll focus on big, important, strategic things (real time data is really good at driving the best companies to do tactical silly things). And you'll save a lot of money, because real time everything is really expensive!
Here's one way to check if you really need real time data: Does a human have to be involved from data receipt to taking action? If the answer is yes, then you don't need real time data, you need right time data. If the answer is no (say you have intelligence/rules driven automated systems), then you need real time data.
5. "Data quality sucks, just get over it."
That is the title of my post from June 2006. And look how far we've come. :)
The core thrust of my post was that data on the web will never get to 95% clean and it will have big holes and it will be sparse in some areas. We should aim to collect, process and store data as cleanly as humanly possible, but after that we should move on to using the data, because we will still have more data about the web than what God's blessed any other channel with. Let's not become the type of people who continue to waste time on quality beyond the point of diminishing returns. Let's not become persistent javascript hackers and sprop variable tweakers at the cost of delivering value from data now.
Multiply all of that a million times when it comes to big data. We will have dirty data. We will have no idea what to do with videos or spoken text or (omg!) social media overload. We will be missing primary keys. We will suffer from a lack of clean meta data (or sometimes any meta data!). We will realize the shallow limits of sentiment analysis. We will cry from the pain of the painful business process fixes that usually result in good data.
And yet, we are standing on a mountain of gold.
Do the best you can in terms of collecting, processing, and storing data of the cleanest possible quality. Know when to shift to data analysis. Start making decisions. Make small ones at first. (Remember, even they will be revolutionary, as these datasets have never come together!) Make bigger ones over time, as you understand the limitations of what you are dealing with.
Here's the kiss of death: Big data implementation projects where the first touch of an Analyst will come 18 months after the project was first conceived. You see, the world would have changed so dramatically in 18 months that nothing you possibly spec'ed for is relevant any more.
Think smart. Move fast. Slowly become Godlike over time.
6. Eliminating noise is even more important than finding a signal.
This might be a little controversial. But stay with me.
Thus far in the history data analysis the objective for our queries has been trying to find the signal amongst all the noise in the data. That has worked very well. We had clean business questions. The data size was smaller and the data set was more complete and we often knew what we were looking for. Known knowns and known unknowns. (See video above.)
With big data, it is so much more important to be magnificent at knowing what to ignore. You must know how to separate out all the noise in the disparate huge datasets to even have a fighting chance to start to look for the signal.
It is amazing but true. If you are not magnificent at knowing what to ignore, you'll never get a chance to pay attention to the stuff to which you should be paying attention.
Your business savvy. Your analytical gut instinct. Tuning your algorithms to first ignore and then hunt for insights. That is what will have a material impact.
Six simple rules for you revolutionaries to follow to ensure, well, revolutionary success.
Notice, none of them have to do with hardware or Hadoop. One important reason is that I'm solving for the CEO and not the CIO/CTO, so it is a matter of perspective. The second (main) reason is that we do face some big data technology challenges for now, but the things that will determine if big data will deliver big value have nothing to with technology. They have to do with the six rules above.
If you are really thinking big data value, think CEO and not CIO/CTO. It will dramatically change the focus of your work, in a good way.
As always, it's your turn now.
Did you find the keynote to be of value? Did you find the framework to be of value? Will it drive you to change your approach to big data? With regards to the rules above … is there one rule above that is your favorite? Is there one that should have been there but is missing? What is the biggest big data advice you would share from your experience?
Please share your wisdom, recommendations, and feedback via comments.
Thank you.
Recommend
About Joyk
Aggregate valuable and interesting links.
Joyk means Joy of geeK