

OpenCV开发笔记(七十二):红胖子8分钟带你使用opencv+dnn+tensorFlow识别物体
source link: https://segmentfault.com/a/1190000037586502
Go to the source link to view the article. You can view the picture content, updated content and better typesetting reading experience. If the link is broken, please click the button below to view the snapshot at that time.
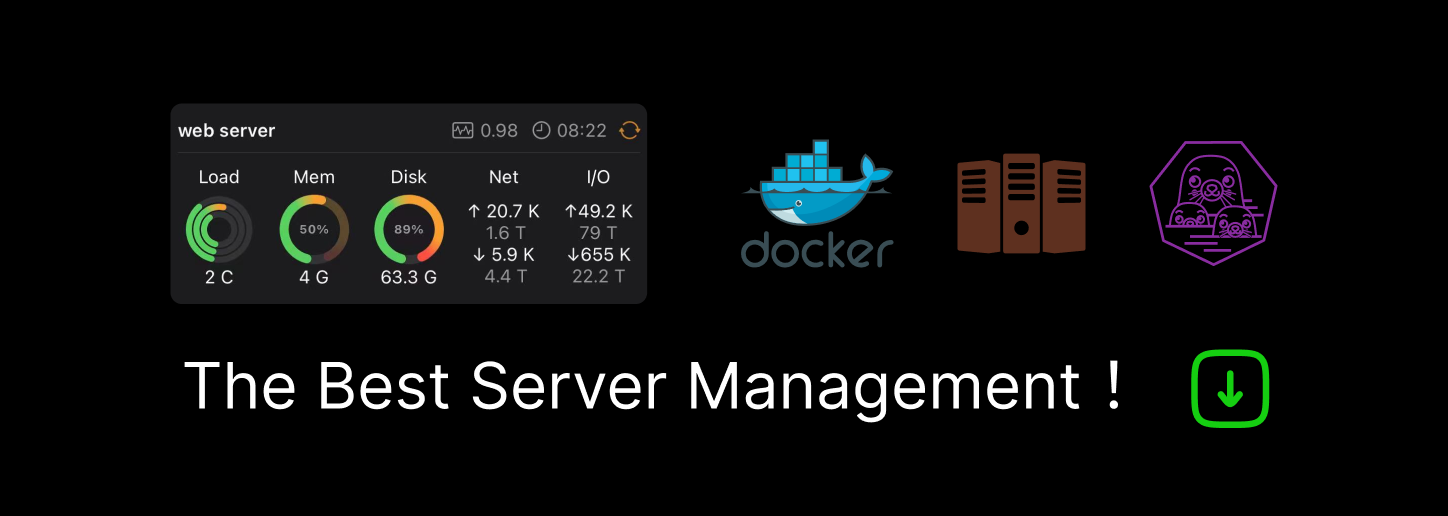
若该文为原创文章,转载请注明原文出处
本文章博客地址: https://blog.csdn.net/qq21497936/article/details/109194717
各位读者,知识无穷而人力有穷,要么改需求,要么找专业人士,要么自己研究
红胖子(红模仿)的博文大全:开发技术集合(包含Qt实用技术、树莓派、三维、OpenCV、OpenGL、ffmpeg、OSG、单片机、软硬结合等等)持续更新中...(点击传送门)OpenCV开发专栏(点击传送门)
上一篇:《 OpenCV开发笔记(七十一):红胖子8分钟带你深入级联分类器训练 》
下一篇:持续补充中…
前言
级联分类器的效果并不是很好,准确度相对深度学习较低,本章使用opencv通过tensorflow深度学习,检测已有模型的分类。
Demo
可以猜测,1其实是人,18序号类是狗,因为笔者未找到对应的分类具体信息。
Tensorflow模型下载
https://github.com/opencv/opencv_extra
(注意:未找到对应的分类具体信息。)
OpenCV深度识别基本流程
opencv3.4.x支持了各种模型。
支持的模型
opencv3.4.x支持一下深度学习的模型:
- caffe:.caffemodel
官网: http://caffe.berkeleyvision.org
- tensorflow:.pb
官网: https://www.tensorflow.org
- torch:.t7 | .net
官网: http://torch.ch
- darknet:.weights官网: https://pjreddie.com/darknet
- DLDT:.bin
操作步骤:tensorflow
- 步骤一 :加载模型和配置文件,建立神经网络。
根据不同的模型,使用cv::dnn::readNetFromXXX系列函数进行读取,opencv3.4.x系列支持的dnn模型(支持模型往上看)。
举例tensorflow模型如下:
std::string weights = "E:/qtProject/openCVDemo/dnnData/" \ "ssd_mobilenet_v1_coco_2017_11_17/frozen_inference_graph.pb"; std::string prototxt = "E:/qtProject/openCVDemo/dnnData/" \ "ssd_mobilenet_v1_coco_2017_11_17.pbtxt"; cv::dnn::Net net = cv::dnn::readNetFromTensorflow(weights, prototxt);
- 步骤二 :将要预测的图片加入到神经网络中
加入之后,需要识别图片,那么需要把图片输入到神经网络当中去,如下:
cv::Mat mat; cv::Mat blob; mat = cv::imread("E:/testFile/14.jpg"); cv::dnn::blobFromImage(mat, blob);
- 步骤三 :分类预测,获取识别的结果
输入之后,就进行识别,识别是向前预测(分类预测),并且拿到结果。
cv::Mat prob = net.forward();
对于预测的结果,存于cv::Mat类型的prob,然后需要统一对prob进行处理,使其成为我们可以使用的数据,代码如下:
cv::Mat detectionMat(prob.size[2], prob.size[3], CV_32F, prob.ptr<float>());
对于从结果prob转换为detectionMat后,其结构如下:
cv::Mat为多行七列,每一行代表一个检测到的分类,具体列信息如下表:
(注意:具体的使用,请参照“ 步骤四 ”)
- 步骤四 :对达到置信度的可以通过输出的mat进行分类和框选
cv::Mat detectionMat(prob.size[2], prob.size[3], CV_32F, prob.ptr<float>()); // 置信度预制,大于执行度的将其使用rect框出来 float confidenceThreshold = 0.75; for(int i = 0; i < detectionMat.rows; i++) { float confidence = detectionMat.at<float>(i, 2); if (confidence > confidenceThreshold) { // 高于置信度的,获取其x、y、以及对应的宽度高度,进行框选 int classId = (detectionMat.at<float>(i, 1)); int xLeftBottom = static_cast<int>(detectionMat.at<float>(i, 3) * mat.cols); int yLeftBottom = static_cast<int>(detectionMat.at<float>(i, 4) * mat.rows); int xRightTop = static_cast<int>(detectionMat.at<float>(i, 5) * mat.cols); int yRightTop = static_cast<int>(detectionMat.at<float>(i, 6) * mat.rows); cv::Rect object((int)xLeftBottom, (int)yLeftBottom, (int)(xRightTop - xLeftBottom), (int)(yRightTop - yLeftBottom)); cv::rectangle(mat, object, cv::Scalar(0, 255, 0), 2); qDebug() << __FILE__ << __LINE__ << classId << confidence << confidenceThreshold << object.x << object.y << object.width << object.height; } }
函数原型
读取tensorflow模型与配置文件函数原型
Net readNetFromTensorflow(const String &model, const String &config = String());
从文件中读取。
- 参数一 :用二进制协议描述网络体系结构的.pb文件的路径;
- 参数二 :包含protobuf格式的文本图形定义的.pbtxt文件的路径。生成的网络对象由文本图构建,使用来自二进制的权重让我们更灵活些;
Net readNetFromTensorflow(const std::vector<uchar>& bufferModel, const std::vector<uchar>& bufferConfig = std::vector<uchar>());
从缓存中读取。
- 参数一 :包含pb文件内容的bufferModel缓冲区;
- 参数二 :包含pbtxt文件内容的bufferConfig缓冲区;
Net readNetFromTensorflow(const char *bufferModel, size_t lenModel, const char *bufferConfig = NULL, size_t lenConfig = 0);
- 参数一 :包含pb文件内容的bufferModel缓冲区;
- 参数二 :bufferModel缓冲长度;
- 参数三 :包含pbtxt文件内容的bufferConfig缓冲区;
- 参数四 :bufferConfig缓冲长度;
读取图片(需要识别的)函数原型
Mat blobFromImage(InputArray image, double scalefactor=1.0, const Size& size = Size(), const Scalar& mean = Scalar(), bool swapRB=false, bool crop=false, int ddepth=CV_32F);
void blobFromImage(InputArray image, OutputArray blob, double scalefactor=1.0, const Size& size = Size(), const Scalar& mean = Scalar(), bool swapRB=false, bool crop=false, int ddepth=CV_32F);.
Mat blobFromImages(InputArrayOfArrays images, double scalefactor=1.0, Size size = Size(), const Scalar& mean = Scalar(), bool swapRB=false, bool crop=false, int ddepth=CV_32F);
void blobFromImages(InputArrayOfArrays images, OutputArray blob, double scalefactor=1.0, Size size = Size(), const Scalar& mean = Scalar(), bool swapRB=false, bool crop=false, int ddepth=CV_32F);
从图像创建区域。可选择从中心调整和裁剪图像。
- 参数一 :图像输入图像(1、3或4通道);
- 参数二 :大小输出图像的空间大小;
- 参数三 :从通道中减去平均值的平均标量。价值是有意的,如果image有BGR顺序,swapRB为真,则按(mean-R,mean-G,mean-B)顺序排列;
- 参数四 :图像值的缩放因子乘数;
- 参数五 :swapRB标志,指示交换第一个和最后一个通道,在三通道图像是必要的;
- 参数六 :裁剪标志,指示调整大小后是否裁剪图像;
- 参数七 :输出blob的深度,选择CV_32F或CV_8U;
设置神经网络输入函数原型
void cv::dnn::Net::setInput(InputArray blob, const String& name = "", double scalefactor = 1.0, const Scalar& mean = Scalar());
设置网络的新输入值。
- 参数一 :一个新的blob。应具有CV_32F或CV_8U深度。
- 参数二 :输入层的名称。
- 参数三 :可选的标准化刻度。
- 参数四 :可选的平均减去值。
深度检测识别(向前预测)函数原型
void cv::dnn::Net::Mat forward(const String& outputName = String());
向前预测,返回指定层的第一个输出的blob,一般是返回最后一层,可使用cv::Net::getLayarNames()获取所有的层名称。
- 参数一 :outputName需要获取输出的层的名称
Demo源码
void OpenCVManager::testTensorflow() { // 训练好的模型以及其模型的后缀名 // .caffemodel (Caffe, http://caffe.berkeleyvision.org/) // .pb (TensorFlow, https://www.tensorflow.org/) // .t7 | *.net (Torch, http://torch.ch/) // .weights (Darknet, https://pjreddie.com/darknet/) // .bin (DLDT, https://software.intel.com/openvino-toolkit) // https://github.com/opencv/opencv/wiki/TensorFlow-Object-Detection-API std::string weights = "E:/qtProject/openCVDemo/dnnData/" \ "ssd_mobilenet_v1_coco_2017_11_17/"frozen_inference_graph.pb"; std::string prototxt = "E:/qtProject/openCVDemo/dnnData/" \ "ssd_mobilenet_v1_coco_2017_11_17.pbtxt"; cv::dnn::Net net = cv::dnn::readNetFromTensorflow(weights, prototxt); if(net.empty()) { qDebug() << __FILE__ << __LINE__ << "net is empty!!!"; return; } cv::Mat mat; cv::Mat blob; // 获得所有层的名称和索引 std::vector<cv::String> layerNames = net.getLayerNames(); int lastLayerId = net.getLayerId(layerNames[layerNames.size() - 1]); cv::Ptr<cv::dnn::Layer> lastLayer = net.getLayer(cv::dnn::DictValue(lastLayerId)); qDebug() << __FILE__ << __LINE__ << QString(lastLayer->type.c_str()) << QString(lastLayer->getDefaultName().c_str()) << QString(layerNames[layerNames.size()-1].c_str()); #if 0 // 视频里面的识别 cv::VideoCapture capture; if(!capture.open("E:/testFile/4.avi")) { qDebug() << __FILE__ << __LINE__ << "Failed to open videofile!!!"; return; } #endif while(true) { #if 1 // 读取图片识别 mat = cv::imread("E:/testFile/15.jpg"); if(!mat.data) { qDebug() << __FILE__ << __LINE__ << "Failed to read image!!!"; return; } #else // 视频里面的识别 capture >> mat; if(mat.empty()) { cv::waitKey(0); break; } #endif cv::dnn::blobFromImage(mat, blob); net.setInput(blob); // 推理预测:可以输入预测的图层名称 // cv::Mat prob = net.forward("detection_out"); cv::Mat prob = net.forward(); // 显示识别花费的时间 std::vector<double> layersTimes; double freq = cv::getTickFrequency() / 1000; double t = net.getPerfProfile(layersTimes) / freq; std::string label = cv::format("Inference time: %.2f ms", t); cv::putText(mat, label, cv::Point(0, 15), cv::FONT_HERSHEY_SIMPLEX, 0.5, cv::Scalar(0, 255, 0)); cv::Mat detectionMat(prob.size[2], prob.size[3], CV_32F, prob.ptr<float>()); // 置信度预制,大于执行度的将其使用rect框出来 float confidenceThreshold = 0.75; for(int i = 0; i < detectionMat.rows; i++) { float confidence = detectionMat.at<float>(i, 2); if (confidence > confidenceThreshold) { // 高于置信度的,获取其x、y、以及对应的宽度高度,进行框选 int classId = (detectionMat.at<float>(i, 1)); int xLeftBottom = static_cast<int>(detectionMat.at<float>(i, 3) * mat.cols); int yLeftBottom = static_cast<int>(detectionMat.at<float>(i, 4) * mat.rows); int xRightTop = static_cast<int>(detectionMat.at<float>(i, 5) * mat.cols); int yRightTop = static_cast<int>(detectionMat.at<float>(i, 6) * mat.rows); cv::Rect object((int)xLeftBottom, (int)yLeftBottom, (int)(xRightTop - xLeftBottom), (int)(yRightTop - yLeftBottom)); cv::rectangle(mat, object, cv::Scalar(0, 255, 0), 2); qDebug() << __FILE__ << __LINE__ << classId << confidence << confidenceThreshold << object.x << object.y << object.width << object.height; } } cv::imshow(_windowTitle.toStdString(), mat); cv::waitKey(0); } }
对应工程模板v1.64.0
openCVDemo_v1.64.0_基础模板_tensorFlow分类检测.rar。
入坑
入坑一:加载模型时候错误
错误
原因
.pb模型文件与.pbtxt文件不对应,版本也有关系。
解决
更换模型,使用正确的pb与pbtxt对应的文件。
上一篇:《 OpenCV开发笔记(七十一):红胖子8分钟带你深入级联分类器训练 》
下一篇:持续补充中…
Recommend
About Joyk
Aggregate valuable and interesting links.
Joyk means Joy of geeK