

A Novel Approach to Feature Importance — Shapley Additive Explanations
source link: https://mc.ai/a-novel-approach-to-feature-importance - shapley-additive-explanations/
Go to the source link to view the article. You can view the picture content, updated content and better typesetting reading experience. If the link is broken, please click the button below to view the snapshot at that time.
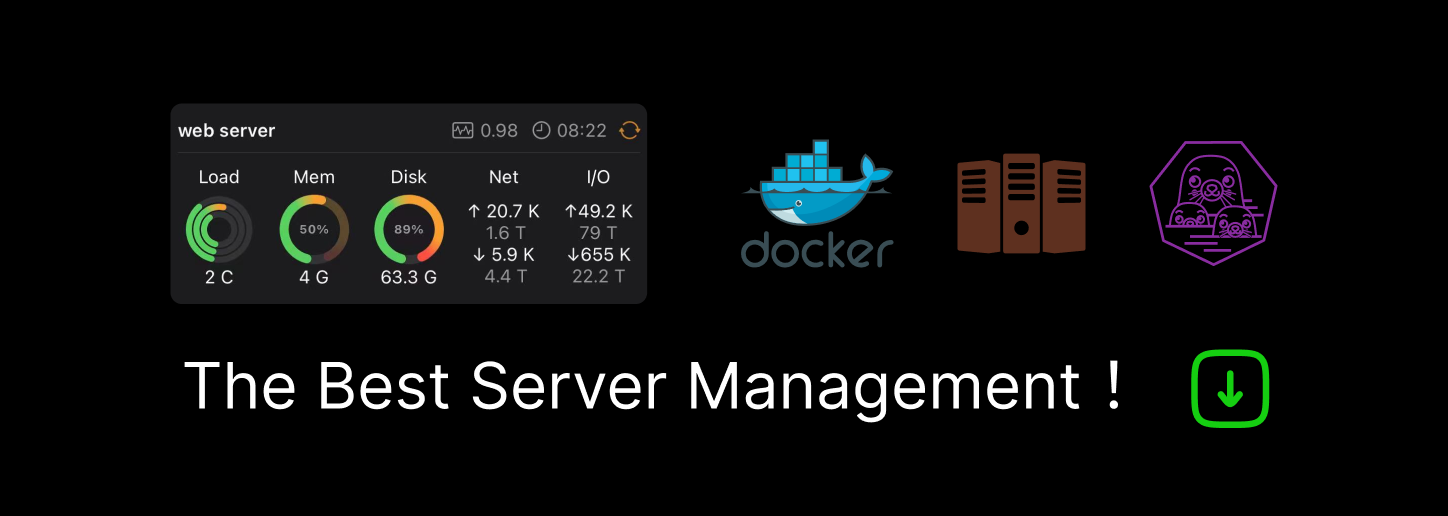
Background
There are many different ways of increasing your model understanding and feature importance is one of them. Feature importance helps you estimate how much each feature of your data contributed to the model’s prediction. After performing feature importance tests, you can figure out which features are making the most impact on your model’s decision making. You can act on this by removing the features which have a low impact on the model’s predictions and focussing on making improvements to the more significant features. This can improve model performance significantly.
There are many ways to calculate feature importance. Some of the basic methods which use statmodels
and scikit-learn
have been discussed in the article here
. However, a lot of people have written about conventional methods, hence, I want to discuss a new approach called Shapely Additive Explanations (ShAP). This method is considered somewhat better than the traditional sckit-learn methods because many of these methods can be inconsistent, which means that the features that are most important may not always be given the highest feature importance score. One example is that in the tree-based models which might give two equally important features different scores based on what level of splitting was done using the features. The features which split the model first might be given higher importance. This is the motivation for using the latest feature attribution method, Shapley Additive Explanations.
Introduction
Let’s start with an example to get some intuition behind this method. Let’s say you’re Mark Cuban and you own a basketball team, let’s say, Dallas Mavericks and you have 3 players —Dirk Nowitzki (A), Michael Finley (B), Jason Kidd (C). You want to determine how much each player contributes to the final score of the team and obviously this does not mean that we just calculate the number of baskets each of them scored because that might work here but won’t work from a machine learning perspective. We want to be able to quantify how much impact their presence has on their team’s performance that extends beyond just calculating the number of baskets each player might have scored. The second reason is that not all of them might be playing in the same position. One of the players might play at an offensive position and the other might play at a defensive position and we want to be able to take that into account as well.
One approach is that you calculate the team’s performance with and without Player A. The impact of player A can be the difference between the team’s performance with and without player A.
Impact of A = Team Performance with A - Team performance without A
This can be extended to each of the players and we can calculate their importance individually. This is the main intuition behind Shapely Additive Explanations. We estimate how important a model is by seeing how well the model performs with and without that feature for every combination of features. It is important to note that Shapley Additive Explanations calculates the local feature importance for every observation which is different from the method used in scikit-learn which computes the global feature importance. You can understand that the importance of a feature may not be uniform across all data points. So, local feature importance calculates the importance of each feature for each data point. A global measure refers to a single ranking of all features for the model. Local feature importance becomes relevant in certain cases as well, like, loan application where each data point is an individual person to ensure fairness and equity. I can also think of a hybrid example, like, credit card fraud detection where each person has multiple transactions. While each person will have a different feature importance ranking, there needs to be a global measure for all transactions to detect outliers in the transactions. I am writing this article from a financial perspective in mind and for that global feature importance is more relevant. You can get the global measure by aggregating the local feature importances for each data point.
Note:- This is just an example and comparing the player stats may not be the owner’s job but I like Mark Cuban on Shark Tank and, hence, the example.
This method calculates something called Shapley values and based on coalition game theory. It was first introduced in 2017 by Lundberg, Scott M. and Su-In Lee [1]. The feature values of a data instance act as players in a coalition. Shapley values tell us how to fairly distribute the “payout” (= the prediction) among the features. A “player” can be an individual feature or a group of features.
How To Calculate the Shapley Values for one feature?
This value is the average marginal contribution of a feature value across all the possible combinations of features. Let’s extend the previous example and look at the number of points the team scored in every match for a season. We want to know how much Player A contributes to the points the team scores in a match. We will, hence, calculate the contribution of the feature Player A when it is added to a coalition of Player B and Player C.
Note:-For this experiment, we need to have all the trials already done with and without each player. I am assuming that in a season there are matches where we can get the relevant data because there must be at least one match where each player was not picked while the other two were. Secondly, this is just an example and the metric could be anything from point difference to tournament rankings. I have just taken the total points for the ease of explanation.
Step 1:Combination of Player B and Player C without Player A
For this case, we can take an average of the points scored in all matches where players B and C were playing and Player A wasn’t. We can also just sample one random example but I think the average/median is a better measure. Let’s say the average was equal to 65 points.
Step 2:Combination of Player B, Player A and Player C
In this step, we will take an average of all those matches where Players A, B and C were playing and let’s say that value is equal to 85 points.
Hence, the contribution of A is 85–65 = 20 points. Intuitive enough, right? If you took one random sample then you should perform this experiment multiple times and average the difference.
The Shapley value is the average of all the marginal contributions to all possible coalitions. The computation time increases exponentially with the number of features. One solution to keep the computation time manageable is to compute contributions for only a few samples of the possible coalitions. [2]
You can look at this notebook for a more detailed explanation. Enough theory! Let’s get our hands dirty with some code.
Recommend
About Joyk
Aggregate valuable and interesting links.
Joyk means Joy of geeK