

最全的损失函数汇总
source link: http://mp.weixin.qq.com/s?__biz=MzI5MDUyMDIxNA%3D%3D&%3Bmid=2247499079&%3Bidx=3&%3Bsn=5738fb46353b3ce456abba80b689d1aa
Go to the source link to view the article. You can view the picture content, updated content and better typesetting reading experience. If the link is broken, please click the button below to view the snapshot at that time.
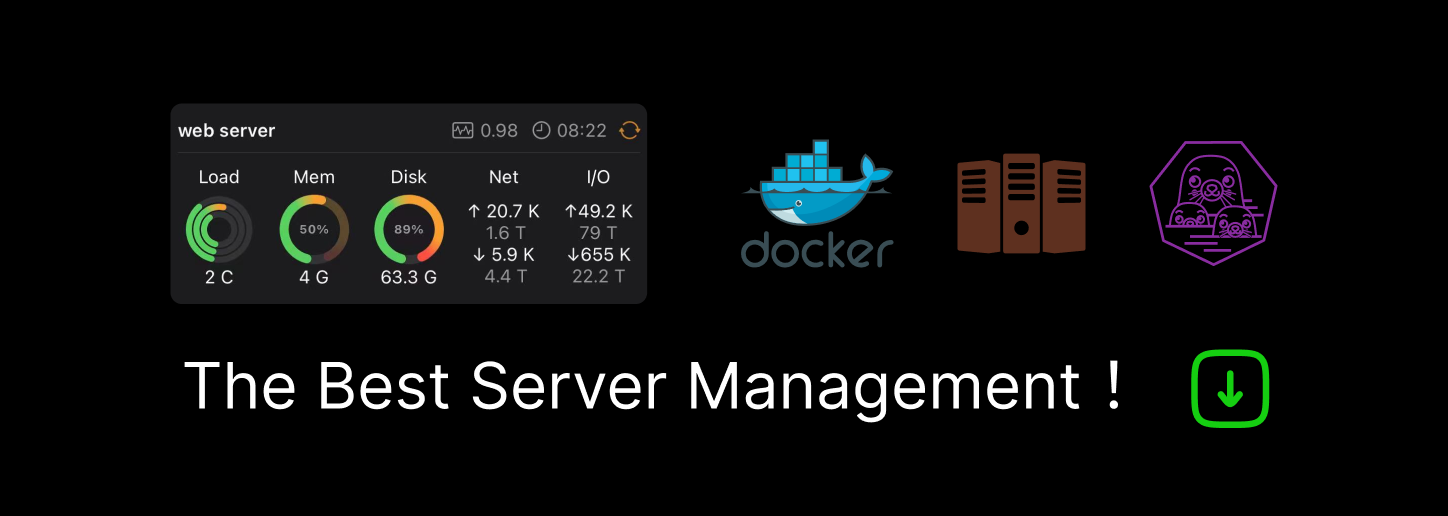
加入极市专业CV交流群,与 1 0000+来自港科大、北大、清华、中科院、CMU、腾讯、百度 等名校名企视觉开发者互动交流!
同时提供每月大咖直播分享、真实项目需求对接、干货资讯汇总,行业技术交流。关注 极市平台 公众号 , 回复 加群, 立刻申请入群~
作者|mingo_敏
来源|https://blog.csdn.net/shanglianlm/article/details/85019768
本文仅作学术分享,如有侵权,请联系后台作删文处理。
tensorflow和pytorch很多都是相似的,这里以pytorch为例。
1. L1范数损失 L1Loss
计算 output 和 target 之差的绝对值。
torch.nn.L1Loss(reduction='mean')
参数:
reduction-三个值,none: 不使用约简;mean:返回loss和的平均值;sum:返回loss的和。默认:mean。
2 均方误差损失 MSELoss
计算 output 和 target 之差的均方差。
torch.nn.MSELoss(reduction='mean')
参数:
reduction-三个值,none: 不使用约简;mean:返回loss和的平均值;sum:返回loss的和。默认:mean。
3 交叉熵损失 CrossEntropyLoss
当训练有 C 个类别的分类问题时很有效. 可选参数 weight 必须是一个1维 Tensor, 权重将被分配给各个类别. 对于不平衡的训练集非常有效。
在多分类任务中,经常采用 softmax 激活函数+交叉熵损失函数,因为交叉熵描述了两个概率分布的差异,然而神经网络输出的是向量,并不是概率分布的形式。所以需要 softmax激活函数将一个向量进行“归一化”成概率分布的形式,再采用交叉熵损失函数计算 loss。

torch.nn.CrossEntropyLoss(weight=None,ignore_index=-100, reduction='mean')
参数:
weight (Tensor, optional) – 自定义的每个类别的权重. 必须是一个长度为 C 的 Tensor ignore_index (int, optional) – 设置一个目标值, 该目标值会被忽略, 从而不会影响到 输入的梯度。 reduction-三个值,none: 不使用约简;mean:返回loss和的平均值;sum:返回loss的和。默认:mean。
4 KL 散度损失 KLDivLoss
计算 input 和 target 之间的 KL 散度。KL 散度可用于衡量不同的连续分布之间的距离, 在连续的输出分布的空间上(离散采样)上进行直接回归时 很有效.
torch.nn.KLDivLoss(reduction='mean')
参数:
reduction-三个值,none: 不使用约简;mean:返回loss和的平均值;sum:返回loss的和。默认:mean。
5 二进制交叉熵损失 BCELoss
二分类任务时的交叉熵计算函数。用于测量重构的误差, 例如自动编码机. 注意目标的值 t[i] 的范围为0到1之间.
torch.nn.BCELoss(weight=None, reduction='mean')
参数:
weight (Tensor, optional) – 自定义的每个 batch 元素的 loss 的权重. 必须是一个长度为 “nbatch” 的 的 Tensor
6 BCEWithLogitsLoss
BCEWithLogitsLoss损失函数把 Sigmoid 层集成到了 BCELoss 类中. 该版比用一个简单的 Sigmoid 层和 BCELoss 在数值上更稳定, 因为把这两个操作合并为一个层之后, 可以利用 log-sum-exp 的 技巧来实现数值稳定.
torch.nn.BCEWithLogitsLoss(weight=None, reduction='mean', pos_weight=None)
参数:
weight (Tensor, optional) – 自定义的每个 batch 元素的 loss 的权重. 必须是一个长度 为 “nbatch” 的 Tensor
7 MarginRankingLoss
torch.nn.MarginRankingLoss(margin=0.0, reduction='mean')
对于 mini-batch(小批量) 中每个实例的损失函数如下:
参数:
margin:默认值0
8 HingeEmbeddingLoss
torch.nn.HingeEmbeddingLoss(margin=1.0, reduction='mean')
对于 mini-batch(小批量) 中每个实例的损失函数如下:

参数:
margin:默认值1
9 多标签分类损失 MultiLabelMarginLoss
torch.nn.MultiLabelMarginLoss(reduction='mean')
对于mini-batch(小批量) 中的每个样本按如下公式计算损失:
10 平滑版L1损失 SmoothL1Loss
也被称为 Huber 损失函数。
torch.nn.SmoothL1Loss(reduction='mean')

其中

11 2分类的logistic损失 SoftMarginLoss
torch.nn.SoftMarginLoss(reduction='mean')

12 多标签 one-versus-all 损失 MultiLabelSoftMarginLoss
torch.nn.MultiLabelSoftMarginLoss(weight=None, reduction='mean')

13 cosine 损失 CosineEmbeddingLoss
torch.nn.CosineEmbeddingLoss(margin=0.0, reduction='mean')
参数:
margin:默认值0
14 多类别分类的hinge损失 MultiMarginLoss
torch.nn.MultiMarginLoss(p=1, margin=1.0, weight=None, reduction='mean')
参数:
p=1或者2 默认值:1
margin:默认值1
15 三元组损失 TripletMarginLoss
和孪生网络相似,具体例子:给一个A,然后再给B、C,看看B、C谁和A更像。

torch.nn.TripletMarginLoss(margin=1.0, p=2.0, eps=1e-06, swap=False, reduction='mean')
其中:
16 连接时序分类损失 CTCLoss
CTC连接时序分类损失,可以对没有对齐的数据进行自动对齐,主要用在没有事先对齐的序列化数据训练上。比如语音识别、ocr识别等等。
torch.nn.CTCLoss(blank=0, reduction='mean')
参数:
reduction-三个值,none: 不使用约简;mean:返回loss和的平均值;sum:返回loss的和。默认:mean。
17 负对数似然损失 NLLLoss
负对数似然损失. 用于训练 C 个类别的分类问题.
torch.nn.NLLLoss(weight=None, ignore_index=-100, reduction='mean')
参数:
weight (Tensor, optional) – 自定义的每个类别的权重. 必须是一个长度为 C 的 Tensor ignore_index (int, optional) – 设置一个目标值, 该目标值会被忽略, 从而不会影响到 输入的梯度.
18 NLLLoss2d
对于图片输入的负对数似然损失. 它计算每个像素的负对数似然损失.
torch.nn.NLLLoss2d(weight=None, ignore_index=-100, reduction='mean')
参数:
weight (Tensor, optional) – 自定义的每个类别的权重. 必须是一个长度为 C 的 Tensor reduction-三个值,none: 不使用约简;mean:返回loss和的平均值;sum:返回loss的和。默认:mean。
19 PoissonNLLLoss
目标值为泊松分布的负对数似然损失
torch.nn.PoissonNLLLoss(log_input=True, full=False, eps=1e-08, reduction='mean')
参数:
log_input (bool, optional) – 如果设置为 True , loss 将会按照公 式 exp(input) - target * input 来计算, 如果设置为 False , loss 将会按照 input - target * log(input+eps) 计算. full (bool, optional) – 是否计算全部的 loss, i. e. 加上 Stirling 近似项 target * log(target) - target + 0.5 * log(2 * pi * target). eps (float, optional) – 默认值: 1e-8
参考资料:
pytorch loss function 总结
http://www.voidcn.com/article/p-rtzqgqkz-bpg.html
推荐阅读
添加极市小助手微信 (ID : cv-mart) ,备注: 研究方向-姓名-学校/公司-城市 (如:目标检测-小极-北大-深圳),即可申请加入 极市技术交流群 ,更有 每月大咖直播分享、真实项目需求对接、求职内推、算法竞赛、 干货资讯汇总、行业技术交流 , 一起来让思想之光照的更远吧~
△长按添加极市小助手
△长按关注极市平台,获取 最新CV干货
觉得有用麻烦给个在看啦~
Recommend
About Joyk
Aggregate valuable and interesting links.
Joyk means Joy of geeK