

Silhouette or Elbow? That is the Question.
source link: https://towardsdatascience.com/silhouette-or-elbow-that-is-the-question-a1dda4fb974?gi=28b257cbcd5d
Go to the source link to view the article. You can view the picture content, updated content and better typesetting reading experience. If the link is broken, please click the button below to view the snapshot at that time.
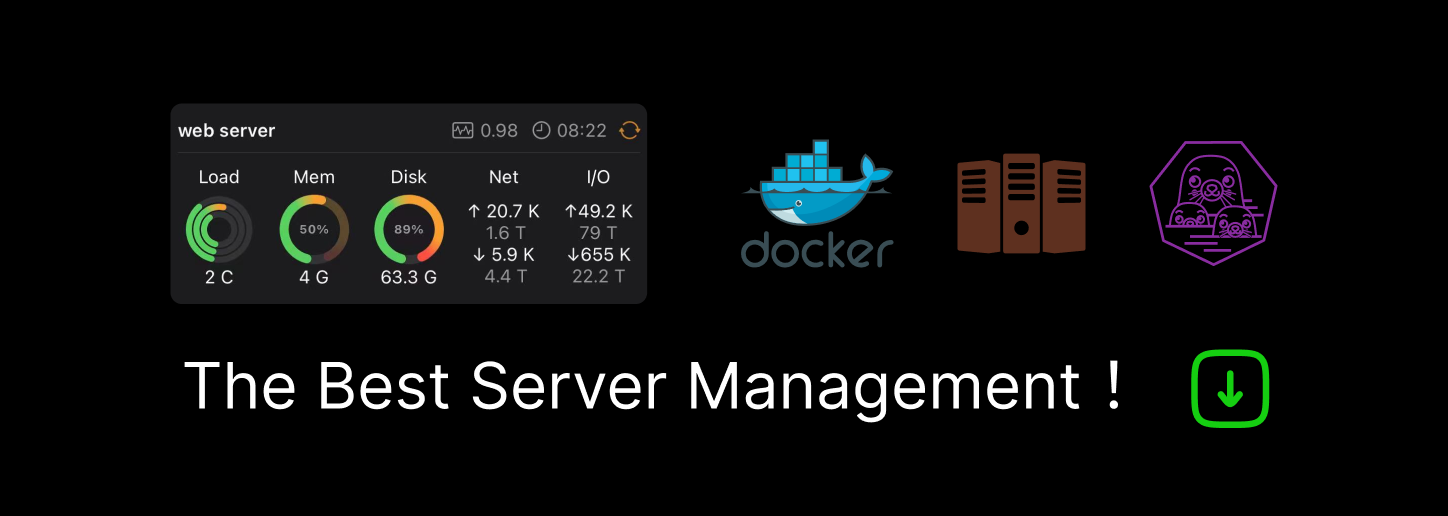
When you want to cluster a dataset with no labels, one of the most common questions that you encounter is “what is the right number of clusters?”. This question often raises when you work with, for example, the k-means algorithm that required you to fix the number of clusters. I encountered this question many times, and I am sure you did as well.
The problem can become more controversial when you work with high-dimensional data. The real-world data are often high-dimensional and you need to reduce its dimension to visualize and analyze it. The clustering results can be different in original space compared to the dimension-reduced space. You used the same algorithm but you see a discrepancy. That means the clustering results are sensitive to the space dimension mostly due to the performance of distance metrics in those spaces also referred to as the curse of dimensionality.
The clustering results can be different in original space compared to the dimension-reduced space. In other words, the clustering results are sensitive to the space dimension. Do not panic!
In this article, I do not want to explain the math behind the silhouette and elbow methods. You probably can easily find it elsewhere. However, I would like to share my experience working with these methods along with some insights that may help you.
— There is no right number of clusters but there is an optimal one.
Let me be very straight. There is no right number of clusters but there is an optimal one. I assume you select, for example, the k-means algorithm for the problem. You must run the algorithm for several consecutive k, i.e., the number of clusters. Then, you must compute the clustering performance for each k. Now, you are able to determine the k such that it works well for your problem.
First, you must select a performance metric that can evaluate the clustering quality as needed. Then, you must run the clustering algorithm with several configurations and evaluate the performance for each run. Now, you have everything to determine the number of clusters such that suits your problem.
The next question you must answer is “What is a good metric to evaluate the clustering performance?”. The answer is “Of course, It depends”.
— You can cluster using inertia and evaluate using the silhouette.
The scoring metric or objective function in a clustering algorithm can be different from the performance metric that you want to use for evaluation. For example, the k-means algorithm is designed to cluster data by minimizing the sum of within-cluster variances, also known as inertia. However, you may want to use the silhouette coefficients to evaluate the clustering performance.
You can not easily change the objective function in the k-means algorithm due to optimization challenges. However, you definitely can use a different performance metric to evaluate the results of the k-means algorithm. Now, you may ask why we do not use a similar metric in both stages? The answer is that I also wish we could do that easily but the k-means algorithm is an NP-hard problem. Under standard configuration, the algorithm converges to a good local-optimum in a reasonable time. However, if you change the objective function there is no guarantee to converge to any good result. Note that there are some works that modified the objective function in the k-means algorithm but similar concerns still exist.
In general, when the objective function in an optimization problem such as a clustering algorithm becomes more complex the search space becomes more rugged. In this case, there is a high chance that the search algorithm does not converge as needed.
— So what?
The silhouette and elbow methods are two simple, yet important, methods to find the optimum number of clusters. The silhouette method uses the silhouette coefficient, and the elbow method used inertia, the original scoring function in the k-means algorithm.
The elbow method only uses intra-cluster distances while the silhouette method uses a combination of inter- and intra-cluster distances. So, you can expect that they end up with different results.
According to the literature, the elbow method is often used with inertia. However, the elbow method, in general, only uses a heuristic to determine the elbow of a curve as a special point. In cluster analysis, the special point indicates the number of clusters. So, you may want to use the elbow method with different scoring functions rather than inertia, although it is not that common.
The Last Word
I suggest using the silhouette since it uses inter- and intra-cluster distances in its scoring function while the elbow method only uses intra-cluster distances. However, this does not mean the silhouette method is better. The silhouette and elbow methods are two out of many methods to determine the number of clusters in a dataset. If you want to learn more, you can also read about the information criterion methods such as the Akaike information criterion and Bayesian information criterion. As I said above neither of them is better nor worse. They all capture different characteristics of your data.
Recommend
About Joyk
Aggregate valuable and interesting links.
Joyk means Joy of geeK