

【NIPS2019】Infidelity and Sensitivity:模型可解释性方法的定量评估
source link: http://mp.weixin.qq.com/s?__biz=MjM5ODkzMzMwMQ%3D%3D&%3Bmid=2650414206&%3Bidx=3&%3Bsn=d723d853f7f0b6d682b14bc88126971a
Go to the source link to view the article. You can view the picture content, updated content and better typesetting reading experience. If the link is broken, please click the button below to view the snapshot at that time.
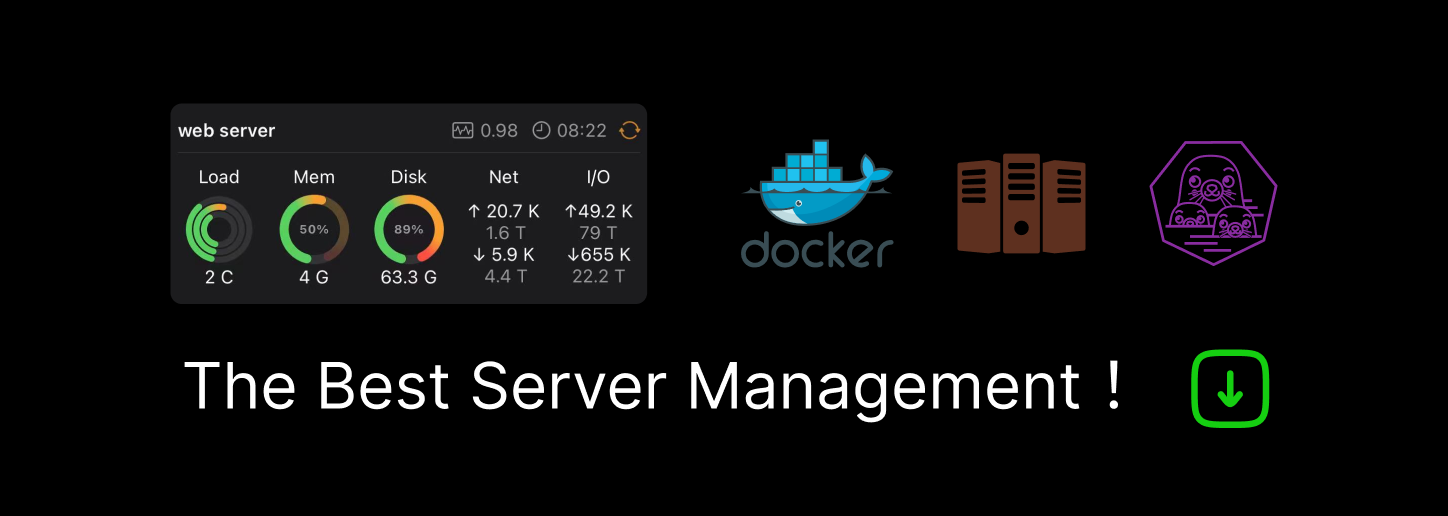
作者: HardenHuang
学校:清华大学
研究方向:自然语言处理
知乎专栏: 模型可解释性论文专栏
NIPS2019的一篇模型可解释性文章,文章主要是提出了模型可解释性方法的两个定量评估指标Infidelity和Sensitivity,同时给出了Infidelity与Sensitivity约束下的最佳可解释性方法。
https://arxiv.org/abs/1901.09392
On the (In)fidelity and Sensitivity for Explanations arxiv.org
如何模型可解释性方法的评估?
模型可解释性方法的评估可以分为主观度量和客观度量两种,可解释性本身是偏人本身的一个概念,因此目前占主流的评估方法为主观度量,但是完全依靠人的主观度量评估模型可解释性方法是不可行的。文章便提出了依靠客观度量去评估模型可解释性方法的两个重要度量 Infidelity 与 Sensitivity 。
Infidelity--失真度
-
完整性公理
Infidelity的定义来源于Ancona [1] 中的completeness axiom(完整性公理),定义如下:
设模型对应函数,输出feature importance的可解释性方法函数 , 定义为对输入 的扰动,则完整性公理的公式如下:
因此完整性公理视最佳可解释性方法函数 为扰动 下模型输出 对输入 的导数。
2 . Infidelity的定量表示
设扰动 为一随机变量,对应的概率分布为 ,Infidelity的定量公式表述为:
假定满足完整性公理的可解释性方法函数 , Infidelity衡量便是可解释性方法函数 与
之间距离期望,该距离期望越小,失真度infidelity越小 。
3. Explanations with least Infidelity
Infidelity度量下,最佳的可解释性方法函数 定义如下,

Integrated Gradient [2] 的公式为 ,
Smoot Gradient [3] 的泛化公式为 , 其中为高斯核函数,同时还可以是其他形式的核函数。
因此可以看出,具有least infidelity的可解释性函数 是Integrated Gradient的平滑版本,对应的核函数形式为
扰动有多种定义形式,如下四种扰动:Difference to baseline, Subset of difference to baseline, Difference to noisy baseline, Difference to multiple baselines
3. Many Recent Explanations Optimize Infidelity
有许多模型可解释性方法可以认为是对给定的扰动约束下,优化infidelity度量。
(1)固定基线扰动: ,此时扰动固定,对应的可解释性方法有Integrated Gradient, Deep Lift [4] , LRP [5]
(2)固定坐标扰动: , 是一个坐标向量偏置

此时的最佳可解释性函数可以认为是梯度可解释性函数 [6]

:
(3)非固定坐标扰动: ,是一个坐标向量偏置
此时最佳的可解释性函数可以认为是occlusion-1 explanation [7]
(4)0-1 mask扰动:设模型输入 ,此时扰动定义为

此时最佳解释函数 与输入 的逐点乘积等于Shapley value [8]
4. Some Novel Explanations with New Perturbations
论文作者提出了一些新的扰动形式,基于该些扰动的可解释性方法在Infidelity和Sensitivity两个维度上都取得最佳效果
(1)noisy baseline: 是对固定基线扰动 的一种改进,此时 变为一个随机变量 ,例如高斯噪声。
(2)Square remove:针对图像的扰动,对图像中的一部分或者数个部分的像素块进行mask。
5. Local and Global Explanations
Local Explanation关注的是模型输出对输入feature变化的敏感度
Global Explanation关注的是输入feature变化导致的模型输出的变化。
Sensitivity-敏感度
论文将Sensitivity定义为可解释性方法函数对输入 的导数,具体的表达如下:
首先定义 ,其中

可得基于梯度的敏感性分数 的公式表达为:
由此得到基于Lips系数的敏感性分数表达
如果可解释性函数的局部满足Lipshitz continuous,我们可以得到最终的敏感性分数 表示

max-sensitivity分数 的主要好处是可以通过蒙特卡洛采样进行计算。
Reducing Sensitivity and Infidelity by Smoothing Explanations
文章指出通过smooth explanation即平滑可解释性函数,可以同时降低sensitivity与infidelity分数。
1.证明降低sensitivity分数
2. 证明降低infidelity分数

Experiment Results
在MNIST, CIFAR-10和ImageNet上的评测对比多种可解释性方法,给出infidelity与sensitivity结果的对比

Grad, IG, GBP, SHAP分别是vanilla gradient , integrated gradient, Guided Back-Propagation [9] and KernelSHAP [10] 等可解释性方法的表示。Grad-SG表示让Grad explanation进行平滑,IG-SG表示让IG explanation进行平滑,GBP-SG表示使GBP explanation进行平滑,Noisy baseline和Square是作者提出的两种新的扰动形式。
从上表可以看出:通过Smooth操作,GBP IG 与Grad等可解释性方法的性能得到了提升,
Noisy baseline和Square两种文章提出的扰动方法,对应的可解释性方法的性能达到最佳。
下面是可视化的一些结果展示

参考
-
^Ancona, M., Ceolini, E., Öztireli, C., and Gross, M. A unified view of gradient-based attribution methods for deep neural networks. International Conference on Learning Representations,2018.
-
^Sundararajan, M., Taly, A., and Yan, Q. Axiomatic attribution for deep networks. In International Conference on Machine Learning, 2017.
-
^Daniel Smilkov, Nikhil Thorat, Been Kim, Fernanda B. Viegas, and Martin Wattenberg. 2017. SmoothGrad: ´ removing noise by adding noise. In ICML Workshop on Visualization for Deep Learning.
-
^Shrikumar, A., Greenside, P., and Kundaje, A. Learning important features through propagating activation differences. International Conference on Machine Learning, 2017.
-
^Bach, S., Binder, A., Montavon, G., Klauschen, F., Müller, K.-R., and Samek, W. On pixel-wise explanations for non-linear classifier decisions by layer-wise relevance propagation. PloS one, 10(7):e0130140, 2015.
-
^Shrikumar, A., Greenside, P., Shcherbina, A., and Kundaje, A. Not just a black box: Learning important features through propagating activation differences. arXiv preprint arXiv:1605.01713, 2016.
-
^Zeiler, M. D. and Fergus, R. Visualizing and understanding convolutional networks. In European conference on computer vision, pp. 818–833. Springer, 2014.
-
^Lundberg, S. M. and Lee, S.-I. A unified approach to interpreting model predictions. In Advances in Neural Information Processing Systems, pp. 4765–4774, 2017.
-
^vanilla gradient [37], integrated gradient [43], Guided Back-Propagation [41], and KernelSHAP [25]
-
^Lundberg, S. M. and Lee, S.-I. A unified approach to interpreting model predictions. In Advances in Neural Information Processing Systems, pp. 4765–4774, 2017.
本文由作者授权AINLP原创发布于公众号平台,欢迎投稿,AI、NLP均可。 原文链接,点击"阅读原文"直达:
https://zhuanlan.zhihu.com/p/112033098
作者相关的两篇文章:
可解释性论文阅读笔记1-Tree Regularization
可解释性论文阅读笔记2-Leveraging Language Models
推荐阅读
征稿启示| 200元稿费+5000DBC(价值20个小时GPU算力)
文本自动摘要任务的“不完全”心得总结番外篇——submodular函数优化
斯坦福大学NLP组Python深度学习自然语言处理工具Stanza试用
太赞了!Springer面向公众开放电子书籍,附65本数学、编程、机器学习、深度学习、数据挖掘、数据科学等书籍链接及打包下载
数学之美中盛赞的 Michael Collins 教授,他的NLP课程要不要收藏?
关于AINLP
AINLP 是一个有趣有AI的自然语言处理社区,专注于 AI、NLP、机器学习、深度学习、推荐算法等相关技术的分享,主题包括文本摘要、智能问答、聊天机器人、机器翻译、自动生成、知识图谱、预训练模型、推荐系统、计算广告、招聘信息、求职经验分享等,欢迎关注!加技术交流群请添加AINLPer(id:ainlper),备注工作/研究方向+加群目的。
阅读至此了,点个在看吧 :point_down:
Recommend
About Joyk
Aggregate valuable and interesting links.
Joyk means Joy of geeK