

BERT Text Classification Using Pytorch
source link: https://towardsdatascience.com/bert-text-classification-using-pytorch-723dfb8b6b5b?gi=71b739c6454
Go to the source link to view the article. You can view the picture content, updated content and better typesetting reading experience. If the link is broken, please click the button below to view the snapshot at that time.
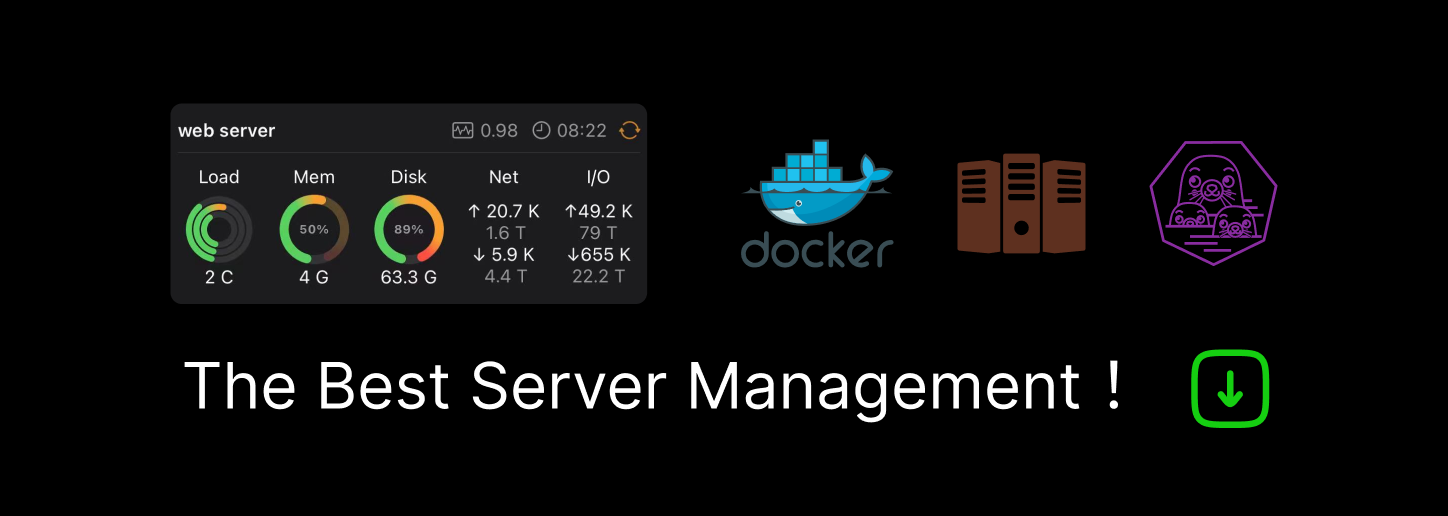
Intro
Text classificationis one of the most common tasks in NLP. It is applied in a wide variety of applications, including sentiment analysis, spam filtering, news categorization, etc. Here, we show you how you can detect fake news (classifying an article as REAL or FAKE) using the state-of-the-art models, a tutorial that can be extended to really any text classification task.
The Transformer is the basic building block of most current state-of-the-art architectures of NLP. Its primary advantage is its multi-head attention mechanisms which allow for an increase in performance and significantly more parallelization than previous competing models such as recurrent neural networks. In this tutorial, we will use pre-trained BERT , one of the most popular transformer models, and fine-tune it on fake news detection.
The main source code of this article is available in this Google Colab Notebook .
The preprocessing code is also available in this Google Colab Notebook .
Getting Started
Huggingface is the most well-known library for implementing state-of-the-art transformers in Python. It offers clear documentation and tutorials on implementing dozens of different transformers for a wide variety of different tasks. We will be using Pytorch so make sure Pytorch is installed. After ensuring relevant libraries are installed, you can install the transformers library by:
pip install transformers
For the dataset, we will be using the REAL and FAKE News Dataset from Kaggle.
Step 1: Importing Libraries
The most important library to note here is that we imported BERTokenizer and BERTSequenceClassification to construct the tokenizer and model later on.
Step 2: Preprocess and Prepare Dataset
In the original dataset, we added an additional TitleText column which is the concatenation of title and text. We want to test whether an article is fake using both the title and the text.
For the tokenizer, we use the “bert-base-uncased” version of BertTokenizer. Using TorchText , we first create the Text Field and the Label Field. The Text Field will be used for containing the news articles and the Label is the true target. We limit each article to the first 128 tokens for BERT input. Then, we create a TabularDataset from our dataset csv files using the two Fields to produce the train, validation, and test sets. Then we create Iterators to prepare them in batches.
Note: In order to use BERT tokenizer with TorchText, we have to set use_vocab=False
and tokenize=tokenizer.encode
. This will let TorchText know that we will not be building our own vocabulary using our dataset from scratch, but instead, use the pre-trained BERT tokenizer and its corresponding word-to-index mapping.
Step 3: Build Model
We are using the “bert-base-uncased” version of BERT, which is the smaller model trained on lower-cased English text (with 12-layer, 768-hidden, 12-heads, 110M parameters). Check out Huggingface’s documentation for other versions of BERT or other transformer models.
Step 4: Training
We write save and load functions for model checkpoints and training metrics, respectively. Note that the save function for model checkpoint does not save the optimizer. We do not save the optimizer because the optimizer normally takes very large storage space and we assume no training from a previous checkpoint is needed. The training metric stores the training loss, validation loss, and global steps so that visualizations regarding the training process can be made later.
We use Adam optimizer and a suitable learning rate to tune BERT for 5 epochs.
We use BinaryCrossEntropy as the loss function since fake news detection is a two-class problem. Make sure the output is passed through Sigmoid before calculating the loss between the target and itself.
During training, we evaluate our model parameters against the validation set. We save the model each time the validation loss decreases so that we end up with the model with the lowest validation loss, which can be considered as the best model. Here are the outputs during training:
After training, we can plot a diagram using the code below:
Step 5: Evaluation
For evaluation, we predict the articles using our trained model and evaluate it against the true label. We print out classification report which includes test accuracy, precision, recall, F1-score. We also print out the confusion matrix to see how much data our model predicts correctly and incorrectly for each class.
After evaluating our model, we find that our model achieves an impressive accuracy of 96.99%!
Conclusion
We find that fine-tuning BERT performs extremely well on our dataset and is really simple to implement thanks to the open-source Huggingface Transformers library. This can be extended to any text classification dataset without any hassle.
References
[1] A. Vaswani, N. Shazeer, N. Parmar, etc., Attention Is All You Need (2017), 31st Conference on Neural Information Processing Systems
[2] J. Devlin, M. Chang, K. Lee and K. Toutanova, BERT: Pre-training of Deep Bidirectional Transformers for Language Understanding (2019), 2019 Annual Conference of the North American Chapter of the Association for Computational Linguistics
Recommend
About Joyk
Aggregate valuable and interesting links.
Joyk means Joy of geeK