

Good practices in AI projects
source link: https://towardsdatascience.com/good-practices-in-ai-projects-2f36a6a51e36?gi=a039dcdfd029
Go to the source link to view the article. You can view the picture content, updated content and better typesetting reading experience. If the link is broken, please click the button below to view the snapshot at that time.
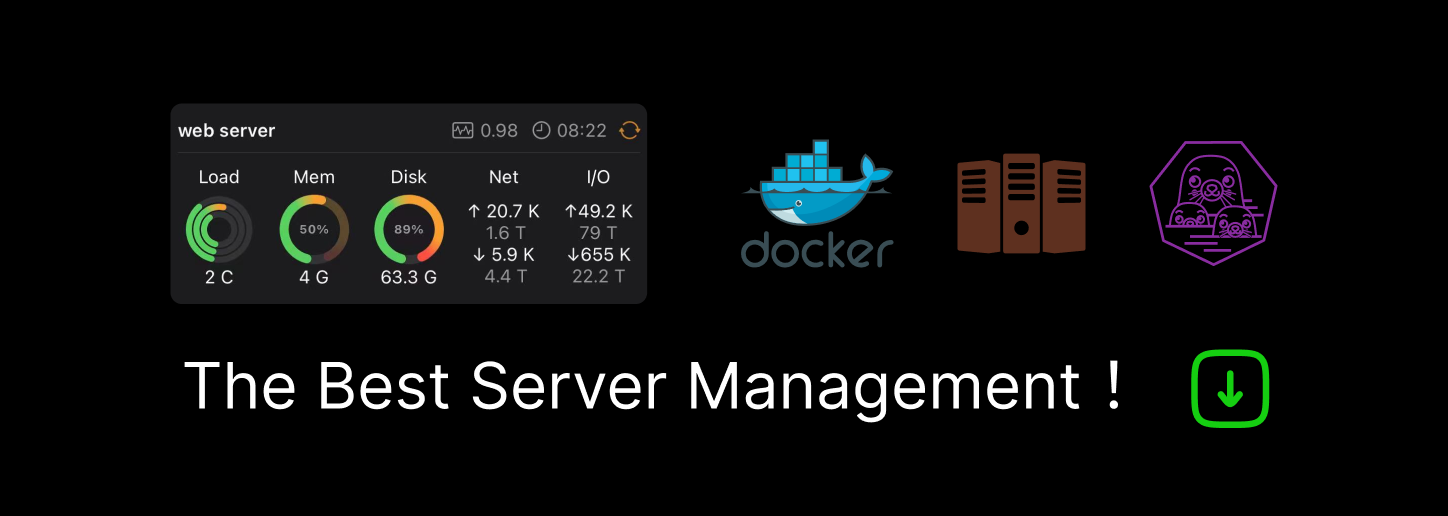
Best practices in AI
What determines the success of machine learning projects
Apr 9 ·5min read
In myprevious article, I made an effort to identify risks that are specific to AI projects and describe possible ways of addressing them. The intent was to write an essay that avoids scientific language and is understandable by professionals without a technical background.
This time, I’d like to take a look from a more technical perspective and, similarly to software engineering , try to determine best practices that help in running AI projects.
Understand your data and business
As it’s been already mentioned , the data introduces one of the critical risks related both to the quality and business understanding. Therefore, a required step before approaching any machine learning problem is to understand the data and the domain.
The exploratory data analysis is a step that can’t be avoided. Luckily, there are plenty of tools and programming languages that offer support in this activity. Business understanding is a little bit more complicated, as it requires interviewing Subject Matter Experts and spending time on familiarizing with the business domain. However, it’s a necessity for project success.
The above should result in well-defined metrics that help in setting clear goals and tracking the project not only from a machine learning perspective but also including business factors.
Stand on the shoulders of giants .
It’s highly likely that someone has already faced a problem similar to yours and found the right solution. Therefore, it doesn’t make sense to reinvent the wheel but instead to start with someone else experiences.
Literature review ,blogs, and evaluating available open-source codes can help you to determine the initial direction and shortlist possible solutions that might be used to build the product.
Don’t believe everything stated in the papers.
On the other hand, many papers have only one goal: to be accepted to conferences ( cargo cult science ). To make it happen, researchers try to sell their research just as marketers sell toothpaste. I barely remember seeing a paper addressing not only the advantages and superiority of a given method but also its limitations and drawbacks. Therefore, it’s a good practice to approach each article with a dose of skepticism and common sense. You don’t believe in every advertisement on TV, don’t you?
Start with a straightforward approach.
Running a simple approach may give you more insights regarding the problem than a more complicated one, as simple methods and their results are easier to interpret. Moreover, implementing, training, and evaluating a simple model is way less time consuming than a sophisticated one.
Define your baseline
How do you know that your state-of-the-art billion parameters model does better than a naive solution? As sophisticated methods not always outperform more straightforward approaches, it is a good practice to have a simple baseline that helps in tracking the gain offered by complex strategies. Sometimes the benefit is minimal, and a simple method might be preferable for a given task for reasons like inference speed or deployment costs.
Plan and track your experiments
Each of the experiments performed in an AI project provides new insights regarding the problem and its solution. Therefore, careful analysis and interpretation of the outcome allow determining the next steps.
Moreover, numerous different variables may influence the performance of AI algorithms. The statement is particularly valid for deep learning models as one can experiment with model architectures, cost functions, and hyper-parameters. Hence, tracking the trials becomes challenging, primarily if many people work together.
The solution is simply a lab notebook. Depending on the team size and your needs, it might be a straightforward approach as a shared spreadsheet or a more sophisticated one as MLflow or Weights and Biases . Undoubtedly tracking and sharing experiment results among team members is a must!
Don’t spend too much time on finetuning.
As not all the papers come with code, it is a common challenge to implement some methods entirely from scratch based only on the publication content. However, it is a very tough task to develop an algorithm that delivers the “publication” quality.
The results presented in the papers are often an effect of pushing the described methods to their limits. The extra gain of accuracy percentage fractions might be an effect of many time-consuming experiments. Moreover, papers are not step-by-step implementation guides but instead focus on describing the essential concepts of the presented method. Therefore, the authors don’t mention many nuances that might be important from the implementation perspective.
An AI project (just like any other type of project) is usually time-constrained and requires a wise approach to time management. Hence, if the project has a different goal than replicating some publication precisely, “close enough” results might be sufficient to stop the implementation. This remark is crucial if several approaches require implementation and evaluation.
Make your experiments reproducible.
It doesn’t bring much value to the project if you managed to achieve 99% accuracy, but you are not able to reproduce this result. Therefore, you should guarantee that your experiments might be repeated.
First of all, use version control , not only to your code but also to your data . There are several tools for code versioning, but the data versioning is also gaining more and more attention, which results in solutions suitable for data science projects like DVC . When you guarantee you use a given code version with a specific data version, you are almost there.
Machine learning frameworks are non-deterministic and rely on pseudo-random numbers generators. Therefore one may obtain different results on different runs. To make things fully reproducible, store the seed you used to initialize your weights. You may find an instruction on how to do that in the docs of your favorite framework. For example, the PyTorch version is here.
Maintain code quality
It might be surprising that I refer to code quality, as this practice has been evident for many years in software engineering ventures, and it may seem like everything has already been said. Unfortunately, AI projects still struggle with this issue very often.
There is a quite common term “research code,” which is an excuse for poor quality code that is barely readable. The authors usually say, the focus was to build and evaluate a new method rather than to care for code quality. And that is a good excuse, as long as no one else is made to reuse such implementation, there is no need for changes or deployment to production. Unfortunately, all of those points are an inherent part of each commercial project. Therefore, as soon as you make your code available to the others, refactor it and make it human pleasant.
Moreover, sometimes not only the code quality is poor, but also the project structure makes it hard to understand. Also, in this case, you may benefit from already tools, like Cookiecutter , which help in maintaining a clear code organization.
Summary
The above practices are the minimal set of tools, which I try to be equipped with, in each machine learning project. From my experience, they make a powerful combination with clear communication among all team members.
The final good practice is not too loose common sense, as blindly applying any rules might bring more harm than good.
Recommend
About Joyk
Aggregate valuable and interesting links.
Joyk means Joy of geeK