

Dating Algorithms using Machine Learning and AI
source link: https://mc.ai/dating-algorithms-using-machine-learning-and-ai/
Go to the source link to view the article. You can view the picture content, updated content and better typesetting reading experience. If the link is broken, please click the button below to view the snapshot at that time.
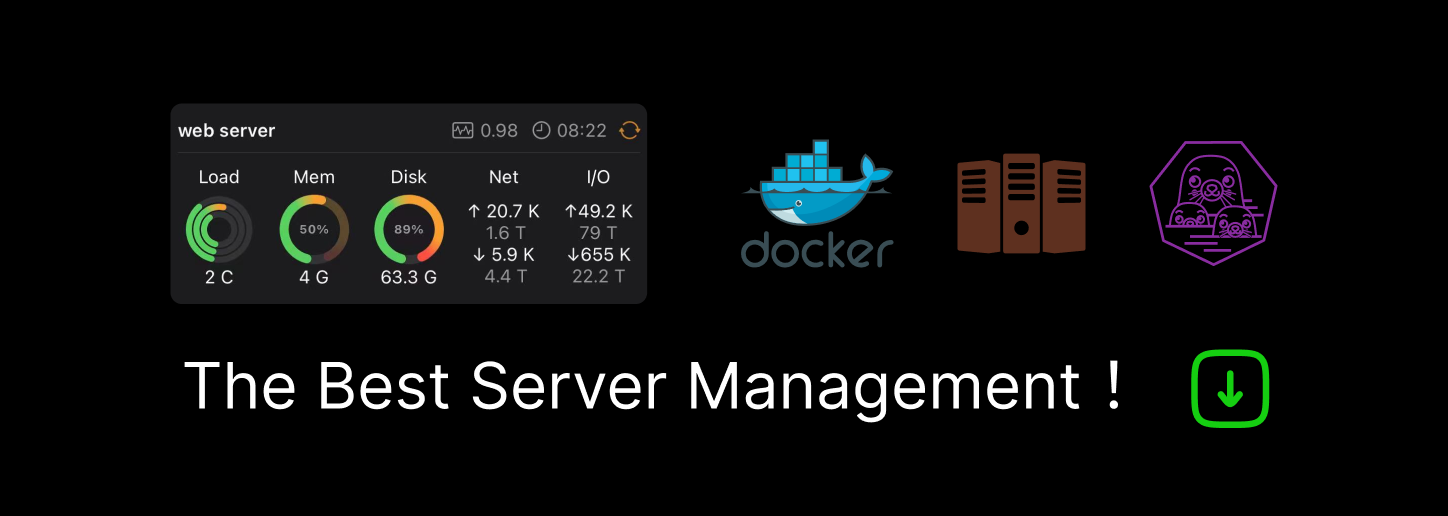
Preparing the Profile Data
To begin, we must first import all the necessary libraries we will need in order for this clustering algorithm to run properly. We will also load in the Pandas DataFrame, which we created when we forged the fake dating profiles.
With our dataset good to go, we can begin the next step for our clustering algorithm.
Scaling the Data
The next step, which will assist our clustering algorithm’s performance, is scaling the dating categories ( Movies, TV, religion, etc ). This will potentially decrease the time it takes to fit and transform our clustering algorithm to the dataset.
# Instantiating the Scaler scaler = MinMaxScaler()# Scaling the categories then replacing the old values df = df[['Bios']].join( pd.DataFrame( scaler.fit_transform( df.drop('Bios',axis=1)), columns=df.columns[1:], index=df.index))
Vectorizing the Bios
Next, we will have to vectorize the bios we have from the fake profiles. We will be creating a new DataFrame containing the vectorized bios and dropping the original ‘ Bio ’ column. With vectorization we will implementing two different approaches to see if they have significant effect on the clustering algorithm. Those two vectorization approaches are: Count Vectorization and TFIDF Vectorization . We will be experimenting with both approaches to find the optimum vectorization method.
Here we have the option of either using CountVectorizer()
or TfidfVectorizer()
for vectorizing the dating profile bios. When the Bios
have been vectorized and placed into their own DataFrame, we will concatenate them with the scaled dating categories to create a new DataFrame with all the features we need.
Based on this final DF, we have more than 100 features. Because of this, we will have to reduce the dimensionality of our dataset by using Principal Component Analysis (PCA).
PCA on the DataFrame
In order for us to reduce this large feature set, we will have to implement Principal Component Analysis (PCA) . This technique will reduce the dimensionality of our dataset but still retain much of the variability or valuable statistical information.
What we are doing here is fitting and transforming our last DF, then plotting the variance and the number of features. This plot will visually tell us how many features account for the variance.
After running our code, the number of features that account for 95% of the variance is 74 . With that number in mind, we can apply it to our PCA function to reduce the number of Principal Components or Features in our last DF to 74 from 117 . These features will now be used instead of the original DF to fit to our clustering algorithm.
Recommend
About Joyk
Aggregate valuable and interesting links.
Joyk means Joy of geeK