

Github 3.4k星,200余行代码,让你实时从视频中隐身
source link: http://mp.weixin.qq.com/s?__biz=MzI5MDUyMDIxNA%3D%3D&%3Bmid=2247493834&%3Bidx=2&%3Bsn=4bb2d96580a35f0b47b20e9373c99333
Go to the source link to view the article. You can view the picture content, updated content and better typesetting reading experience. If the link is broken, please click the button below to view the snapshot at that time.
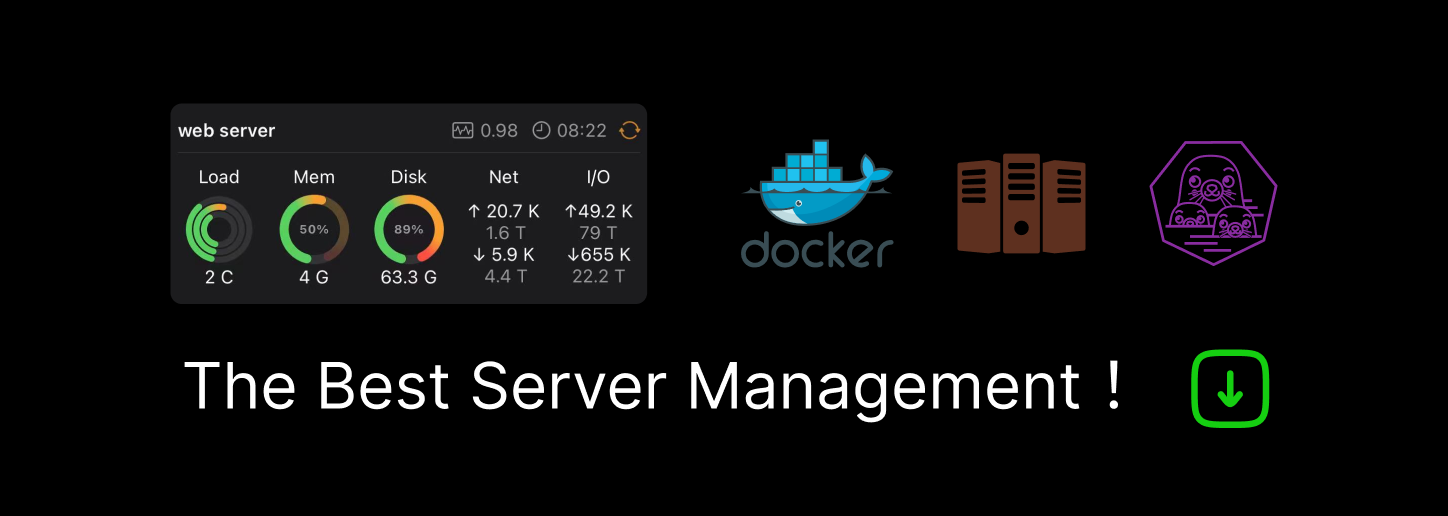
加入极市 专业CV交流群,与1 0000+来自腾讯,华为,百度,北大,清华,中科院 等名企名校视觉开发者互动交流!
同时提供每月大咖直播分享、真实项目需求对接、干货资讯汇总,行业技术交流。 关注 极市平台 公众号 , 回复 加群, 立刻申请入群~
来源:机器之心@微信公众号
只 需网页端,秒速消失 不留痕。
Jason Mayes 是一名在谷歌工作的资深网页工程师,他长期致力于运用新兴技术提供物联网解决方案。近日,充满奇思妙想的 Mayes 又使用 TensorFlow.js 制作了一个仅用 200 余行代码的项目,名为 Real-Time-Person-Removal。它能够实时将复杂背景中的人像消除,而且仅基于网页端。
现在,Mayes 在 GitHub 上开源了他的代码,并在 Codepen.io 上提供了演示 Demo。从视频中看到,你现在只需要一台能上网的电脑和一个网络摄像头就能体验它。
-
项目地址:https://github.com/jasonmayes/Real-Time-Person-Removal
-
Demo 地址:https://codepen.io/jasonmayes/pen/GRJqgma
目前,该项目异常火热,在 Github 上已经获得了 3.4k 的 Star 量。
实时隐身不留痕项目作者:Jason Mayes
我们先来看一下运行的效果。下图中,上半部分是原始视频,下半部分是使用 TensorFlow.js 对人像进行消除后的视频。可以看到,除了偶尔会在边缘处留有残影之外,整体效果还是很不错的。
为了展现这个程序在复杂背景下消除人像并重建背景的能力,Mayes 特意在床上放了一台正在播放视频的笔记本电脑。当他的身体遮挡住笔记本电脑时,可以看到消除算法暂停在电脑被遮挡前的播放画面,并能在人移开时迅速地重建出当前画面。
此外,Mayes 还在 Codepen.io 上提供了能够直接运行的示例。只需要点击 Enable Webcam,离开摄像头一段距离确保算法能够较全面的收集到背景图像,之后当你再出现在摄像头前时就能从下方的预览窗口看到「隐形」后的画面了。
网友表示有了这个程序,像之前 BBC 直播中孩子闯进门来那样的大型翻车现场就有救了。
项目运行机制
Mayes 开发的这个人像消除程序背后的运行机制十分简单,他使用了 TensorFlow.js 中提供的一个预训练的 MobileNet,用于人像分割。
const bodyPixProperties = {
architecture: 'MobileNetV1' ,
outputStride: 16 ,
multiplier: 0.75 ,
quantBytes: 4
};
TensorFlow.js 提供的部分计算机视觉预训练模型。
MobileNet 是谷歌在 2017 年针对移动端和嵌入式设备提出的网络,针对图像分割。其核心思想是使用深度可分离卷积构建快速轻量化的网络架构。Mayes 选择使用它的原因也是出于其轻量化的原因,假如使用 YOLO 或者 Fast-RCNN 这类物体检测算法的话,在移动端就很难做到实时性。
通过 MobileNet 的输出获得检测到人物像素的边界框。
// Go through pixels and figure out bounding box of body pixels.
for ( let x = 0 ; x < canvas.width; x++) {
for ( let y = 0 ; y < canvas.height; y++) {
let n = y * canvas.width + x;
// Human pixel found. Update bounds.
if (segmentation.data[n] !== 0 ) {
if (x < minX) {
minX = x;
}
if (y < minY) {
minY = y;
}
if (x > maxX) {
maxX = x;
}
if (y > maxY) {
maxY = y;
}
foundBody = true ;
}
}
}
为避免人物没有被检测完全的现象,这里使用变量额 scale 对检测区域进行适当放缩。这个 1.3 的参数是测试出来的,感兴趣的读者可以调整试试看。
// Calculate dimensions of bounding box.
var width = maxX - minX;
var height = maxY - minY;
// Define scale factor to use to allow for false negatives around this region.
var scale = 1.3 ;
// Define scaled dimensions.
var newWidth = width * scale;
var newHeight = height * scale;
// Caculate the offset to place new bounding box so scaled from center of current bounding box.
var offsetX = (newWidth - width) / 2 ;
var offsetY = (newHeight - height) / 2 ;
var newXMin = minX - offsetX;
var
newYMin = minY - offsetY;
之后对人物 bounding box 之外的区域进行更新,并且当检测到人物移动时,更新背景区域。
// Now loop through update backgound understanding with new data
// if not inside a bounding box.
for (let x = 0 ; x < canvas.width; x++) {
for (let y = 0 ; y < canvas.height; y++) {
// If outside bounding box and we found a body, update background.
if (foundBody && (x < newXMin || x > newXMin + newWidth) || ( y < newYMin || y > newYMin + newHeight)) {
// Convert xy co-ords to array offset.
let n = y * canvas.width + x;
data [n * 4 ] = dataL[n * 4 ];
data [n * 4 + 1 ] = dataL[n * 4 + 1 ];
data [n * 4 + 2 ] = dataL[n * 4 + 2 ];
data [n * 4 + 3 ] = 255 ;
} else if (!foundBody) {
// No body found at all, update all pixels.
let n = y * canvas.width + x;
data [n * 4 ] = dataL[n * 4 ];
data [n * 4 + 1 ] = dataL[n * 4 + 1 ];
data [n * 4 + 2 ] = dataL[n * 4 + 2 ];
data [n * 4 + 3 ] = 255 ;
}
}
}
ctx.putImageData(imageData, 0 , 0 );
if (DEBUG) {
ctx.strokeStyle = "#00FF00"
ctx.beginPath();
ctx.rect(newXMin, newYMin, newWidth, newHeight);
ctx.stroke();
}
}
至此为算法的核心部分,用了这个程序,你也可以像灭霸一样弹一个响指(单击一下鼠标)让人凭空消失。
热门的「视频隐身术」
其实,这并第一个消除视频中人像的项目。
2019 年的「video-object-removal」项目:只要画个边界框,模型就能自动追踪边界框内的物体,并在视频中隐藏它。
项目地址:https://github.com/zllrunning/video-object-removal
但从项目效果来看,也会有一些瑕疵,例如去掉了行人后,背景内的车道线对不齐等。
与 Mayes 的这个项目类似,video-object-removal 主要借鉴了 SiamMask 与 Deep Video Inpainting,它们都来自 CVPR 2019 的研究。通过 SiamMask 追踪视频中的目标,并将 Mask 传递给 Deep Video Inpainting,然后模型就能重建图像,完成最终的修复了。
对此类技术感兴趣的读者可自行运行下这两个项目,做下对比。
-END -
推荐阅读:
极市平台视觉算法季度赛,提供真实应用场景数据和免费算力,特殊时期,一起在家打比赛吧!
添加极市小助手微信 (ID : cv-mart) ,备注: 研究方向-姓名-学校/公司-城市 (如:目标检测-小极-北大-深圳),即可申请加入 目标检测、目标跟踪、人脸、工业检测、医学影像、三维&SLAM、图像分割等极市技术交流群 ,更有 每月大咖直播分享、真实项目需求对接、求职内推、算法竞赛、 干货资讯汇总、行业技术交流 , 一起来让思想之光照的更远吧~
△长按添加极市小助手
△长按关注极市平台,获取 最新CV干货
觉得有用麻烦给个在看啦~
Recommend
About Joyk
Aggregate valuable and interesting links.
Joyk means Joy of geeK