

60题PyTorch简易入门指南,做技术的弄潮儿!
source link: http://mp.weixin.qq.com/s?__biz=MzIyNjM2MzQyNg%3D%3D&%3Bmid=2247488351&%3Bidx=1&%3Bsn=44421aeaaa7ce1aa49208b211a03140a
Go to the source link to view the article. You can view the picture content, updated content and better typesetting reading experience. If the link is broken, please click the button below to view the snapshot at that time.
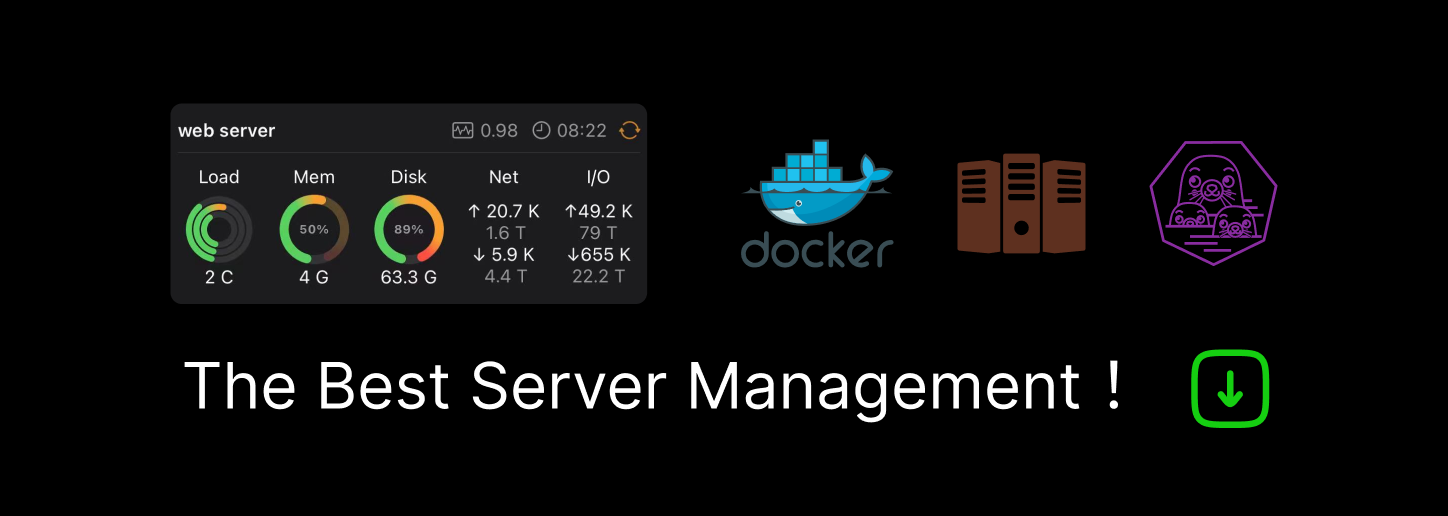
Datawhale
Datawhale,和鲸社区编辑
PyTorch是一个基于Python的库,提供了一个具有灵活易用的深度学习框架,是近年来最受欢迎的深度学习框架之一。
未经授权,禁止转载。
1 初识PyTorch
1.1 张量
1.导入pytorch包
import torch
2.创建一个空的5x3张量
x = torch.empty(5, 3) print(x)
3.创建一个随机初始化的5x3张量
x = torch.rand(5, 3) print(x)
4.创建一个5x3的0张量,类型为long
x = torch.zeros(5, 3, dtype=torch.long) print(x)
5.直接从数组创建张量
x = torch.tensor([5.5, 3]) print(x)
6.创建一个5x3的单位张量,类型为double
x = torch.ones(5, 3, dtype=torch.double) print(x)
7.从已有的张量创建相同维度的新张量,并且重新定义类型为float
x = torch.randn_like(x, dtype=torch.float) print(x)
8.打印一个张量的维度
print(x.size())
9.将两个张量相加
y = torch.rand(5, 3) print(x + y) # 方法二 # print(torch.add(x, y)) # 方法三 # result = torch.empty(5, 3) # torch.add(x, y, out=result) # print(result) # 方法四 # y.add_(x) # print(y)
10.取张量的第一列
print(x[:, 1])
11.将一个4x4的张量resize成一个一维张量
x = torch.randn(4, 4) y = x.view(16) print(x.size(),y.size())
12.将一个4x4的张量,resize成一个2x8的张量
y = x.view(2, 8) print(x.size(),y.size()) # 方法二 z = x.view(-1, 8) # 确定一个维度,-1的维度会被自动计算 print(x.size(),z.size())
13.从张量中取出数字
x = torch.randn(1) print(x) print(x.item())
1.2 Numpy的操作
14.将张量装换成numpy数组
a = torch.ones(5) print(a) b = a.numpy() print(b)
15.将张量+1,并观察上题中numpy数组的变化
a.add_(1) print(a) print(b)
16.从numpy数组创建张量
import numpy as np a = np.ones(5) b = torch.from_numpy(a) print(a) print(b)
17.将numpy数组+1并观察上题中张量的变化
np.add(a, 1, out=a) print(a) print(b)
2 自动微分
2.1 张量的自动微分
18.新建一个张量,并设置
requires_grad=True
x = torch.ones(2, 2, requires_grad=True) print(x)
19.对张量进行任意操作(y = x + 2)
y = x + 2 print(y) print(y.grad_fn) # y就多了一个AddBackward
20.再对y进行任意操作
z = y * y * 3 out = z.mean() print(z) # z多了MulBackward print(out) # out多了MeanBackward
2.2 梯度
21.对out进行反向传播
out.backward()
22.打印梯度d(out)/dx
print(x.grad) #out=0.25*Σ3(x+2)^2
23.创建一个结果为矢量的计算过程(y=x*2^n)
x = torch.randn(3, requires_grad=True) y = x * 2 while y.data.norm() < 1000: y = y * 2 print(y)
24.计算
v = [0.
1, 1.0, 0.0001]
处的梯度
v = torch.tensor([0.1, 1.0, 0.0001], dtype=torch.float) y.backward(v) print(x.grad)
25.关闭梯度的功能
print(x.requires_grad) print((x ** 2).requires_grad) with torch.no_grad(): print((x ** 2).requires_grad) # 方法二 # print(x.requires_grad) # y = x.detach() # print(y.requires_grad) # print(x.eq(y).all())
3 神经网络
这部分会实现LeNet5,结构如下所示

import torch import torch.nn as nn import torch.nn.functional as F class Net(nn.Module): def __init__(self): super(Net, self).__init__() # 26.定义①的卷积层,输入为32x32的图像,卷积核大小5x5卷积核种类6 self.conv1 = nn.Conv2d(3, 6, 5) # 27.定义③的卷积层,输入为前一层6个特征,卷积核大小5x5,卷积核种类16 self.conv2 = nn.Conv2d(6, 16, 5) # 28.定义⑤的全链接层,输入为16*5*5,输出为120 self.fc1 = nn.Linear(16 * 5 * 5, 120) # 6*6 from image dimension # 29.定义⑥的全连接层,输入为120,输出为84 self.fc2 = nn.Linear(120, 84) # 30.定义⑥的全连接层,输入为84,输出为10 self.fc3 = nn.Linear(84, 10) def forward(self, x): # 31.完成input-S2,先卷积+relu,再2x2下采样 x = F.max_pool2d(F.relu(self.conv1(x)), (2, 2)) # 32.完成S2-S4,先卷积+relu,再2x2下采样 x = F.max_pool2d(F.relu(self.conv2(x)), 2) #卷积核方形时,可以只写一个维度 # 33.将特征向量扁平成列向量 x = x.view(-1, 16 * 5 * 5) # 34.使用fc1+relu x = F.relu(self.fc1(x)) # 35.使用fc2+relu x = F.relu(self.fc2(x)) # 36.使用fc3 x = self.fc3(x) return x net = Net() print(net)
37.打印网络的参数
params = list(net.parameters()) # print(params) print(len(params))
38.打印某一层参数的形状
print(params[0].size())
39.随机输入一个向量,查看前向传播输出
input = torch.randn(1, 1, 32, 32) out = net(input) print(out)
40.将梯度初始化
net.zero_grad()
41.随机一个梯度进行反向传播
out.backward(torch.randn(1, 10))
3.2 损失函数
42.用自带的MSELoss()定义损失函数
criterion = nn.MSELoss()
43.随机一个真值,并用随机的输入计算损失
target = torch.randn(10) # 随机真值 target = target.view(1, -1) # 变成列向量 output = net(input) # 用随机输入计算输出 loss = criterion(output, target) # 计算损失 print(loss)
44.将梯度初始化,计算上一步中loss的反向传播
net.zero_grad() print('conv1.bias.grad before backward') print(net.conv1.bias.grad)
45.计算43中loss的反向传播
loss.backward() print('conv1.bias.grad after backward') print(net.conv1.bias.grad)
3.3 更新权重
46.定义SGD优化器算法,学习率设置为0.01
import torch.optim as optim optimizer = optim.SGD(net.parameters(), lr=0.01)
47.使用优化器更新权重
optimizer.zero_grad() output = net(input) loss = criterion(output, target) loss.backward() # 更新权重 optimizer.step()
4 训练一个分类器
4.1 读取CIFAR10数据,做标准化
48.构造一个transform,将三通道(0,1)区间的数据转换成(-1,1)的数据
import torchvision import torchvision.transforms as transforms transform = transforms.Compose( [transforms.ToTensor(), transforms.Normalize((0.5, 0.5, 0.5), (0.5, 0.5, 0.5))])
读取数据集
trainset = cifar(root = './input/cifar10', segmentation='train', transforms=transform) testset = cifar(root = './input/cifar10', segmentation='test', transforms=transform) trainloader = torch.utils.data.DataLoader(trainset, batch_size=batch_size,shuffle=True, num_workers=2) testloader = torch.utils.data.DataLoader(testset, batch_size=batch_size,shuffle=False, num_workers=2) classes = ('plane', 'car', 'bird', 'cat', 'deer', 'dog', 'frog', 'horse', 'ship', 'truck')
4.2 建立网络
这部分沿用前面的网络
net2 = Net()
4.3 定义损失函数和优化器
49.定义交叉熵损失函数
criterion2 = nn.CrossEntropyLoss()
50.定义SGD优化器算法,学习率设置为0.001,
momentum=0.9
optimizer2 = optim.SGD(net2.parameters(), lr=0.001, momentum=0.9)
4.4训练网络
for epoch in range(2): running_loss = 0.0 for i, data in enumerate(trainloader, 0): # 获取X,y对 inputs, labels = data # 51.初始化梯度 optimizer2.zero_grad() # 52.前馈 outputs = net2(inputs) # 53.计算损失 loss = criterion2(outputs, labels) # 54.计算梯度 loss.backward() # 55.更新权值 optimizer2.step() # 每2000个数据打印平均代价函数值 running_loss += loss.item() if i % 2000 == 1999: # print every 2000 mini-batches print('[%d, %5d] loss: %.3f' % (epoch + 1, i + 1, running_loss / 2000)) running_loss = 0.0 print('Finished Training')
4.5 使用模型预测
取一些数据
dataiter = iter(testloader) images, labels = dataiter.next() # print images imshow(torchvision.utils.make_grid(images)) print('GroundTruth: ', ' '.join('%5s' % classes[labels[j]] for j in range(4)))
56.使用模型预测
outputs = net2(images) _, predicted = torch.max(outputs, 1) print('Predicted: ', ' '.join('%5s' % classes[predicted[j]] for j in range(4)))
57.在测试集上进行打分
correct = 0 total = 0 with torch.no_grad(): for data in testloader: images, labels = data outputs = net2(images) _, predicted = torch.max(outputs.data, 1) total += labels.size(0) correct += (predicted == labels).sum().item() print('Accuracy of the network on the 10000 test images: %d %%' % ( 100 * correct / total))
4.6 存取模型
58.保存训练好的模型
PATH = './cifar_net.pth' torch.save(net.state_dict(), PATH)
59.读取保存的模型
pretrained_net = torch.load(PATH)
60.加载模型
net3 = Net()
net3.load_state_dict(pretrained_net)
PDF下载: https://pan.baidu.com/s/1UBMJk99edfMvM8CckCiO9w
AI学习路线和优质资源,在后台回复"AI"获取
Recommend
About Joyk
Aggregate valuable and interesting links.
Joyk means Joy of geeK