

2019 is coming to an end. What’s next for AI?
source link: https://mc.ai/2019-is-coming-to-an-end-whats-next-for-ai/
Go to the source link to view the article. You can view the picture content, updated content and better typesetting reading experience. If the link is broken, please click the button below to view the snapshot at that time.
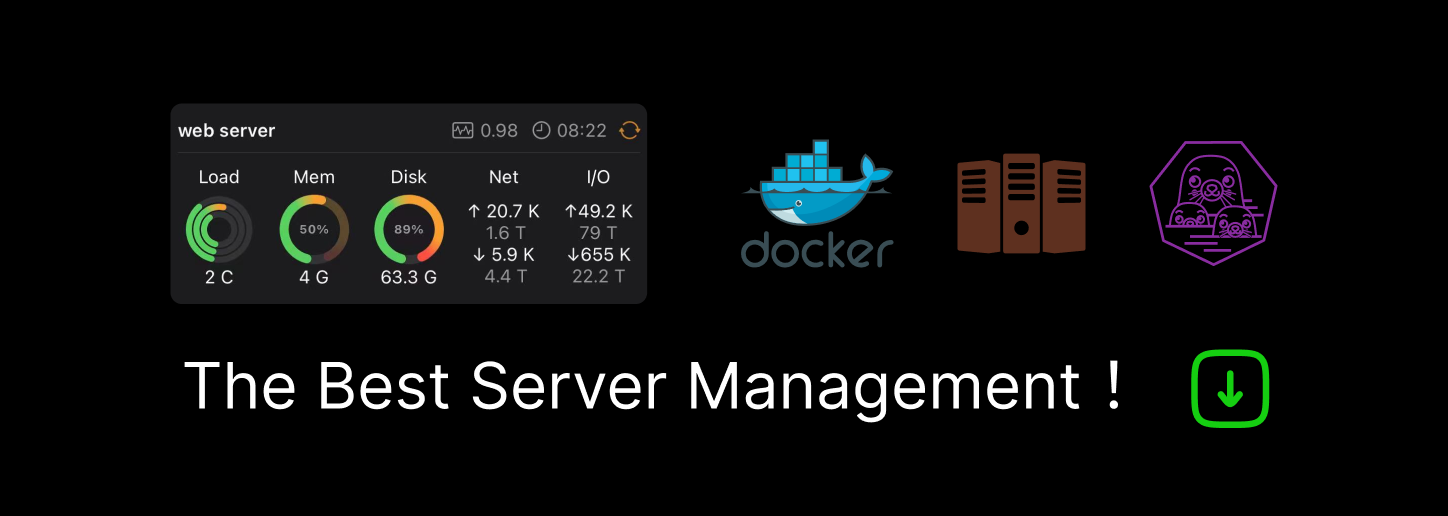
2019 is coming to an end. What’s next for AI?
2019 is ending and two of the most well known reports on AI state of the art — AI Now 2019 report , and AI Index 2019 — are out. What are they saying about AI state of the art? Where is AI moving next? I will try to summarize it in this short post.
Let’s start by AI Index 2019, developed by the Human-Centered Artificial Institute at Stanford University . As a novelty this year, the AI Index includes for the first time two powerful Data visualization tools:
- The Global AI Vibrancy Tool , which allows you to compare countries activities around AI under three metrics including R&D, Economy, Inclusion.
- The ArXiv Monitor , a full paper search engine to track metrics from papers on AI published on ArXiv.
By using those tools, anyone can deep dive into detailed information about the state of AI by country or discipline, but I will try to list here some of the report highlights that I found more interesting while crossing them to some personal thoughts, or thoughts I have shared with some experts in the field :
- China now publishes as many papers on AI per year as Europe , having passed the US in 2006.
- Attendance to AI conferences continues to increase significantly . As an example, 2019 NeuIPS was over 13,000 attendees, 41% over 2018 (and 800% relative to 2012).
- Post 2012, AI compute power is doubling every 3.4 months . That being said, the report also mentions “progress on some broad sets of natural-language processing classification tasks, as captured in the SuperGLUE and SQuAD2.0 benchmarks, has been remarkably rapid; performance is still lower on some NLP tasks requiring reasoning , such as the AI2 Reasoning Challenge, or human-level concept learning task, such as the Omniglot Challenge.”
While discussing these figures with some experts, the feeling they get is that:
- Paper originilaty has definitely decreased .
- An extense amount of papers now focus on just presenting minor improvements over previous work by means of tweaking models, or even by applying brute force (both including dataset scale or computing power).
It seems that the experts I know personally are not the ones pointing in that direction, as you can see in this article in which Yoshua Bengio warns that “ progress is slowing, big challenges remain, and simply throwing more computers at a problem isn’t sustainable ”. Other articles, like this one from MIT Technology Review go further by suggesting the era of deep learning may come to an end .
Also, as I wrote on my article “ Is Deep Learning too big too fail? ” it seems that Deep Learning models are becoming massive while accuracy at scale is not benefiting that much from it .
Another interesting field to look for progress is education. While enrollment in AI training (both in traditional universities and online) is growing , I still find two worrying areas . One of them is mentioned in the AI Index 2019 report highlights, while the other does not:
- Diversity in AI is still an issue . In particular, diversity in gender, with women comprising less that 20% of the new faculty hires in 2018 or PhD in AI recipients.
- While the report focus a lot on AI talent, another relevant topic is missing, which is how are governments and companies training their non technical talent to prepare for AI. Actually, the executive summary of the AI Now 2019 Report clearly states that “ The spread of algorithmic management technology in the workplace is increasing the power asymmetry between workers and employers. AI threatens not only to disproportionately displace lower-wage earners, but also to reduce wages, job security, and other protections for those who need it most .”
Finally, the AI Index Report Highlights points out a trend that has been quite noticeable during the last months. AI Ethics is becoming very relevant with Interpretability and Explainability as most frequently mentioned ethical challanges . This leads me to the second report, the AI Now 2019 Report , which focuses a lot on ethics. Let me try to summarize which I think are some of the most relevant takeaways of this second report. First of all, some executive takeaways:
- AI Ethics pressure comes primarily from communities, journalists and researchers, not companies themselves.
- While there are efforts underway to regulate AI, government adoption for surveillance outpaces them .
- AI investment has profound implications in climate change (note the comptuting power increase rate mentioned before) as well as in geopolitics and inequities reinforcement.
Secondly, regarding the recommendations, the authors make it clear that techniques like affect recognition or facial recognition should be banned or not used while not regulated in sensitive environments that could impact people’s lives and access to opportunities. Regarding bias, the authors point out that research should move beyond technical fixes to address boarder politics consequences.
While I agree, I honestly think that there is another important thing to consider, and that is that bias control should move from research to business implementation , linking this to another recommendation of the paper, being it making Data Scientists accountable for potential risks and harms associated with their models and data .
Of course the reports deal with a lot more topics, and I would be more than happy to discuss any relevant aspect you find interesting. Which are for you the highlights on these two reports?
Recommend
About Joyk
Aggregate valuable and interesting links.
Joyk means Joy of geeK