

GitHub - baowenbo/DAIN: Depth-Aware Video Frame Interpolation (CVPR 2019)
source link: https://github.com/baowenbo/DAIN
Go to the source link to view the article. You can view the picture content, updated content and better typesetting reading experience. If the link is broken, please click the button below to view the snapshot at that time.
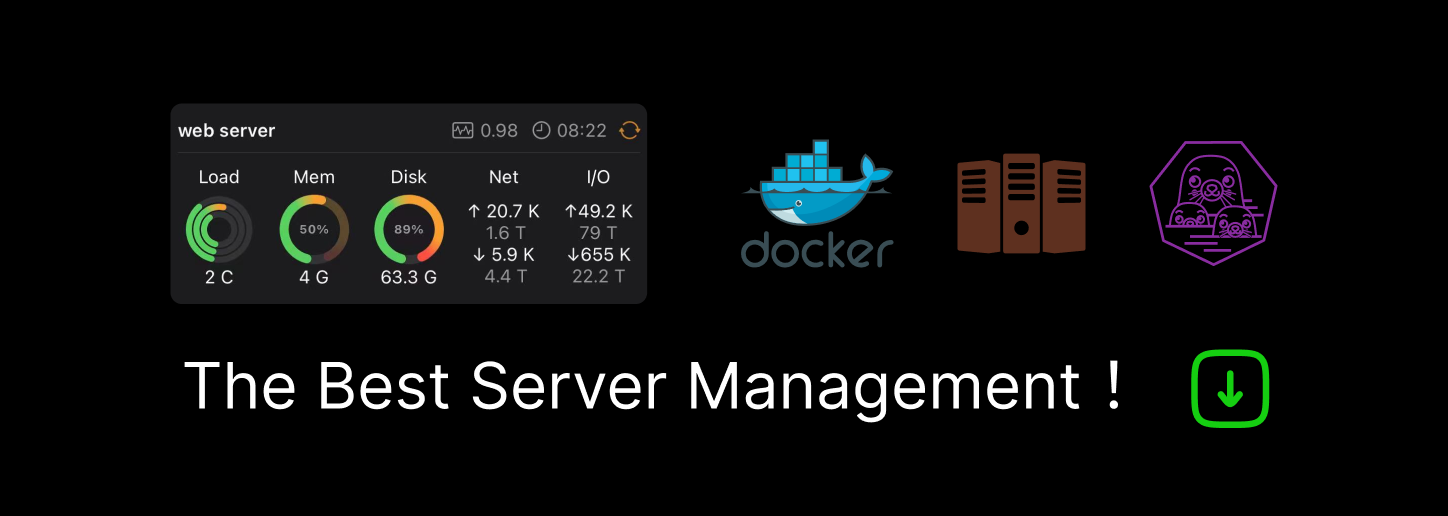
README.md
DAIN (Depth-Aware Video Frame Interpolation)
Wenbo Bao, Wei-Sheng Lai, Chao Ma, Xiaoyun Zhang, Zhiyong Gao, and Ming-Hsuan Yang
IEEE Conference on Computer Vision and Pattern Recognition, Long Beach, CVPR 2019
THIS WORK IS BUILT UPON OUR PREVIOUS WORK CALLED MEMC-NET, IN WHICH WE PROPOSE THE ADAPTIVE WARPING LAYER. PLEASE ALSO CONSIDER CITING MEMC-NET.
Table of Contents
- Introduction
- Citation
- Requirements and Dependencies
- Installation
- Testing Pre-trained Models
- Downloading Results
- Slow-motion Generation
- Training New Models
Introduction
We propose the Depth-Aware video frame INterpolation (DAIN) model to explicitly detect the occlusion by exploring the depth cue. We develop a depth-aware flow projection layer to synthesize intermediate flows that preferably sample closer objects than farther ones. Our method achieves state-of-the-art performance on the Middlebury dataset. We provide videos here.
Citation
If you find the code and datasets useful in your research, please cite:
@inproceedings{DAIN,
author = {Bao, Wenbo and Lai, Wei-Sheng and Ma, Chao and Zhang, Xiaoyun and Gao, Zhiyong and Yang, Ming-Hsuan},
title = {Depth-Aware Video Frame Interpolation},
booktitle = {IEEE Conference on Computer Vision and Pattern Recognition},
year = {2019}
}
Requirements and Dependencies
- Ubuntu (We test with Ubuntu = 16.04.5 LTS)
- Python (We test with Python = 3.6.8 in Anaconda3 = 4.1.1)
- Cuda & Cudnn (We test with Cuda = 9.0 and Cudnn = 7.0)
- PyTorch (The customized depth-aware flow projection and other layers require ATen API in PyTorch = 1.0.0)
- GCC (Compiling PyTorch 1.0.0 extension files (.c/.cu) requires gcc = 4.9.1 and nvcc = 9.0 compilers)
- NVIDIA GPU (We use Titan X (Pascal) with compute = 6.1, but we support compute_50/52/60/61 devices, should you have devices with higher compute capability, please revise this)
Installation
Download repository:
$ git clone https://github.com/baowenbo/DAIN.git
Before building Pytorch extensions, be sure you have pytorch >= 1.0.0
:
$ python -c "import torch; print(torch.__version__)"
Generate our PyTorch extensions:
$ cd DAIN
$ cd my_package
$ ./build.sh
Generate the Correlation package required by PWCNet:
$ cd ../PWCNet/correlation_package_pytorch1_0
$ ./build.sh
Testing Pre-trained Models
Make model weights dir and Middlebury dataset dir:
$ cd DAIN
$ mkdir model_weights
$ mkdir MiddleBurySet
Download pretrained models,
$ cd model_weights
$ wget http://vllab1.ucmerced.edu/~wenbobao/DAIN/best.pth
and Middlebury dataset:
$ cd ../MiddleBurySet
$ wget http://vision.middlebury.edu/flow/data/comp/zip/other-color-allframes.zip
$ unzip other-color-allframes.zip
$ wget http://vision.middlebury.edu/flow/data/comp/zip/other-gt-interp.zip
$ unzip other-gt-interp.zip
$ cd ..
We are good to go by:
$ CUDA_VISIBLE_DEVICES=0 python demo_MiddleBury.py
The interpolated results are under MiddleBurySet/other-result-author/[random number]/
, where the random number
is used to distinguish different runnings.
Downloading Results
Our DAIN model achieves the state-of-the-art performance on the UCF101, Vimeo90K, and Middlebury (eval and other). Dowload our interpolated results with:
$ wget http://vllab1.ucmerced.edu/~wenbobao/DAIN/UCF101_DAIN.zip
$ wget http://vllab1.ucmerced.edu/~wenbobao/DAIN/Vimeo90K_interp_DAIN.zip
$ wget http://vllab1.ucmerced.edu/~wenbobao/DAIN/Middlebury_eval_DAIN.zip
$ wget http://vllab1.ucmerced.edu/~wenbobao/DAIN/Middlebury_other_DAIN.zip
Slow-motion Generation
Our model is fully capable of generating slow-motion effect with minor modification on the network architecture.
Run the following code by specifying time_step = 0.25
to generate x4 slow-motion effect:
$ CUDA_VISIBLE_DEVICES=0 python demo_MiddleBury_slowmotion.py --netName DAIN_slowmotion --time_step 0.25
or set time_step
to 0.125
or 0.1
as follows
$ CUDA_VISIBLE_DEVICES=0 python demo_MiddleBury_slowmotion.py --netName DAIN_slowmotion --time_step 0.125
$ CUDA_VISIBLE_DEVICES=0 python demo_MiddleBury_slowmotion.py --netName DAIN_slowmotion --time_step 0.1
to generate x8 and x10 slow-motion respectively. Or if you would like to have x100 slow-motion for a little fun.
$ CUDA_VISIBLE_DEVICES=0 python demo_MiddleBury_slowmotion.py --netName DAIN_slowmotion --time_step 0.01
You may also want to create gif animations by:
$ cd MiddleBurySet/other-result-author/[random number]/Beanbags
$ convert -delay 1 *.png -loop 0 Beanbags.gif //1*10ms delay
Have fun and enjoy yourself!
Training New Models
Download the Vimeo90K triplet dataset for video frame interpolation task, also see here by Xue et al., IJCV19.
$ cd DAIN
$ mkdir /path/to/your/dataset & cd /path/to/your/dataset
$ wget http://data.csail.mit.edu/tofu/dataset/vimeo_triplet.zip
$ unzip vimeo_triplet.zip
$ rm vimeo_triplet.zip
Download the pretrained MegaDepth and PWCNet models
$ cd MegaDepth/checkpoints/test_local
$ wget http://vllab1.ucmerced.edu/~wenbobao/DAIN/best_generalization_net_G.pth
$ cd ../../../PWCNet
$ wget http://vllab1.ucmerced.edu/~wenbobao/DAIN/pwc_net.pth.tar
$ cd ..
Run the training script:
$ CUDA_VISIBLE_DEVICES=0 python train.py --datasetPath /path/to/your/dataset --batch_size 1 --save_which 1 --lr 0.0001 --rectify_lr 0.0001 --flow_lr_coe 0.01 --occ_lr_coe 0.0 --filter_lr_coe 1.0 --ctx_lr_coe 1.0 --alpha 0.0 1.0 --patience 4 --factor 0.2
The optimized models will be saved to the model_weights/[random number]
directory, where [random number] is generated for different runs.
Replace the pre-trained model_weights/best.pth
model with the newly trained model_weights/[random number]/best.pth
model.
Then test the new model by executing:
$ CUDA_VISIBLE_DEVICES=0 python demo_MiddleBury.py
Contact
Wenbo Bao; Wei-Sheng (Jason) Lai
License
See MIT License
Recommend
About Joyk
Aggregate valuable and interesting links.
Joyk means Joy of geeK