

GitHub - xvjiarui/GCNet: GCNet: Non-local Networks Meet Squeeze-Excitation Netwo...
source link: https://github.com/xvjiarui/GCNet
Go to the source link to view the article. You can view the picture content, updated content and better typesetting reading experience. If the link is broken, please click the button below to view the snapshot at that time.
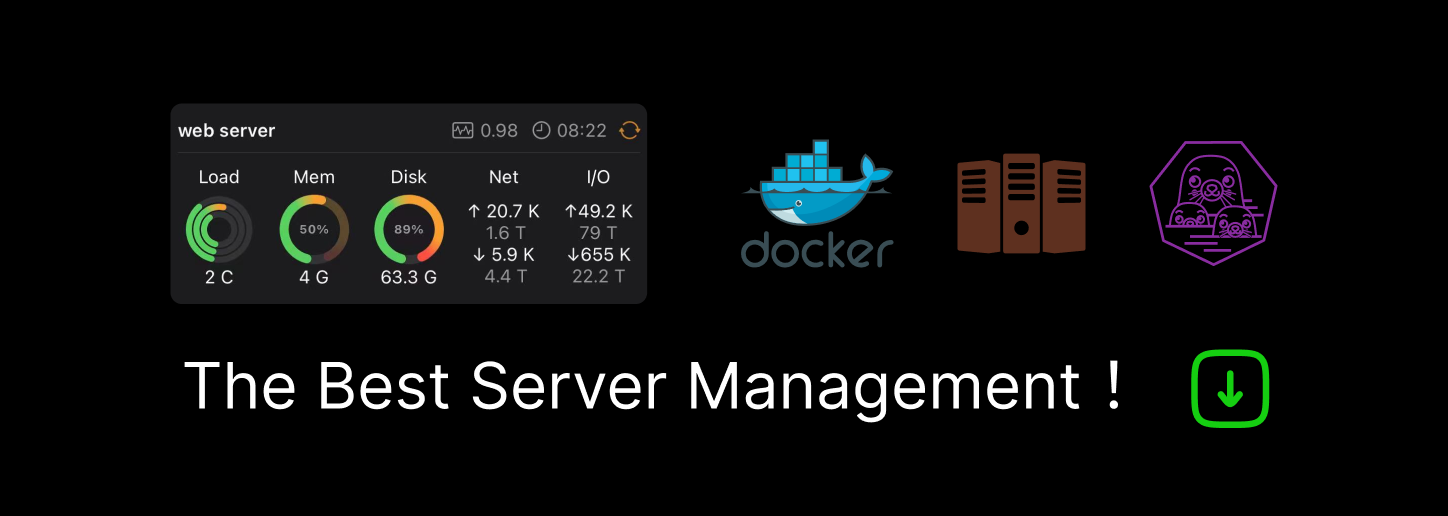
README.md
GCNet for Object Detection
By Yue Cao, Jiarui Xu, Stephen Lin, Fangyun Wei, Han Hu.
This repo is a official implementation of "GCNet: Non-local Networks Meet Squeeze-Excitation Networks and Beyond" on COCO object detection based on open-mmlab's mmdetection. Many thanks to mmdetection for their simple and clean framework.
Introduction
GCNet is initially described in arxiv. Via absorbing advantages of Non-Local Networks (NLNet) and Squeeze-Excitation Networks (SENet), GCNet provides a simple, fast and effective approach for global context modeling, which generally outperforms both NLNet and SENet on major benchmarks for various recognition tasks.
Citing GCNet
@article{cao2019GCNet,
title={GCNet: Non-local Networks Meet Squeeze-Excitation Networks and Beyond},
author={Cao, Yue and Xu, Jiarui and Lin, Stephen and Wei, Fangyun and Hu, Han},
journal={arXiv preprint arXiv:1904.11492},
year={2019}
}
Main Results
Backbone Model Context Lr schd box AP mask AP Download R-50-FPN Mask - 1x 37.2 33.8 model
GC(c3-c5, r16) 1x 39.4 35.7 model
GC(c3-c5, r4) 1x 39.9 36.2 model R-101-FPN Mask - 1x 39.8 36.0 model
GC(c3-c5, r16) 1x 41.1 37.4 model
GC(c3-c5, r4) 1x 41.7 37.6 model X-101-FPN Mask - 1x 41.2 37.3 model
GC(c3-c5, r16) 1x 42.4 38.0 model
GC(c3-c5, r4) 1x 42.9 38.5 model X-101-FPN Cascade Mask - 1x 44.7 38.3 model
GC(c3-c5, r16) 1x 45.9 39.3 model
GC(c3-c5, r4) 1x 46.5 39.7 model X-101-FPN DCN Cascade Mask - 1x 47.1 40.4 model
GC(c3-c5, r16) 1x 47.9 40.9 model
GC(c3-c5, r4) 1x 47.9 40.8 model
Notes:
GC
denotes Global Context (GC) block is inserted after 1x1 conv of backbone.DCN
denotes replace 3x3 conv with 3x3 Deformable Convolution inc3-c5
stages of backbone.r4
andr16
denote ratio 4 and ratio 16 in GC block respectively.
Requirements
- Linux(tested on Ubuntu 16.04)
- Python 3.6+
- PyTorch 1.0.0
- Cython
- apex (Sync BN)
Install
a. Install PyTorch 1.0 and torchvision following the official instructions.
b. Install latest apex with CUDA and C++ extensions following this instructions. The Sync BN implemented by apex is required.
c. Clone the GCNet repository.
git clone https://github.com/xvjiarui/GCNet.git
d. Compile cuda extensions.
cd GCNet pip install cython # or "conda install cython" if you prefer conda ./compile.sh # or "PYTHON=python3 ./compile.sh" if you use system python3 without virtual environments
e. Install GCNet version mmdetection (other dependencies will be installed automatically).
python(3) setup.py install # add --user if you want to install it locally # or "pip install ."
Note: You need to run the last step each time you pull updates from github.
Or you can run python(3) setup.py develop
or pip install -e .
to install mmdetection if you want to make modifications to it frequently.
Please refer to mmdetection install instruction for more details.
Environment
Hardware
- 8 NVIDIA Tesla V100 GPUs
- Intel Xeon 4114 CPU @ 2.20GHz
Software environment
- Python 3.6.7
- PyTorch 1.0.0
- CUDA 9.0
- CUDNN 7.0
- NCCL 2.3.5
Usage
Train
As in original mmdetection, distributed training is recommended for either single machine or multiple machines.
./tools/dist_train.sh <CONFIG_FILE> <GPU_NUM> [optional arguments]
Supported arguments are:
- --validate: perform evaluation every k (default=1) epochs during the training.
- --work_dir <WORK_DIR>: if specified, the path in config file will be replaced.
Evaluation
To evaluate trained models, output file is required.
python tools/test.py <CONFIG_FILE> <MODEL_PATH> [optional arguments]
Supported arguments are:
- --gpus: number of GPU used for evaluation
- --out: output file name, usually ends wiht
.pkl
- --eval: type of evaluation need, for mask-rcnn,
bbox segm
would evaluate both bounding box and mask AP.
Recommend
-
219
How to Remap Active Edge/Squeeze on the Google Pixel 2 Since the Google Pixel 2 and G...
-
179
These are the Best Pixel Phones to buy in 2022 Google Pixel phones are often ranked as the “god tier” of the
-
19
GCNet: Non-local Network Meets Squeeze-Excitation Network and Beyond Review Before getting into the GCNet, we first need to understand two networks: Non-local Networks and Squeeze-Exci...
-
18
Squeeze-and-Excitation Networks Channel self-attention to improve CNN performance Fireworks over Sydney Harbor
-
33
↑ 点击 蓝字 关注极市平台 作者|Happy...
-
9
Sorenson Squeeze with mpeg-4 support Monday, December 2, 2002 I just noticed this article over at maccentr...
-
10
Sorensen Squeeze Update 2.02 Monday, August 26, 2002 Sorenson has released a new version of Sorensen Squeeze for Flash MX . The new version fixes some problems and adds some new features including: Stitchin...
-
15
[Reading] Squeeze-and-Excitation Networks Author: nex3z 2020-08-31 Contents [
-
7
NeurIPS 2019 | 基于 Co-Attention 和 Co-Excitation 的少样本目标检测 11个月前...
-
10
与 SENet 互补提升,华为诺亚提出自注意力新机制:Weight Excitation|ECCV2020 4个月前...
About Joyk
Aggregate valuable and interesting links.
Joyk means Joy of geeK