

How Can We Improve the Quality of Our Data?
source link: https://www.tuicool.com/articles/hit/U7NZ3ez
Go to the source link to view the article. You can view the picture content, updated content and better typesetting reading experience. If the link is broken, please click the button below to view the snapshot at that time.
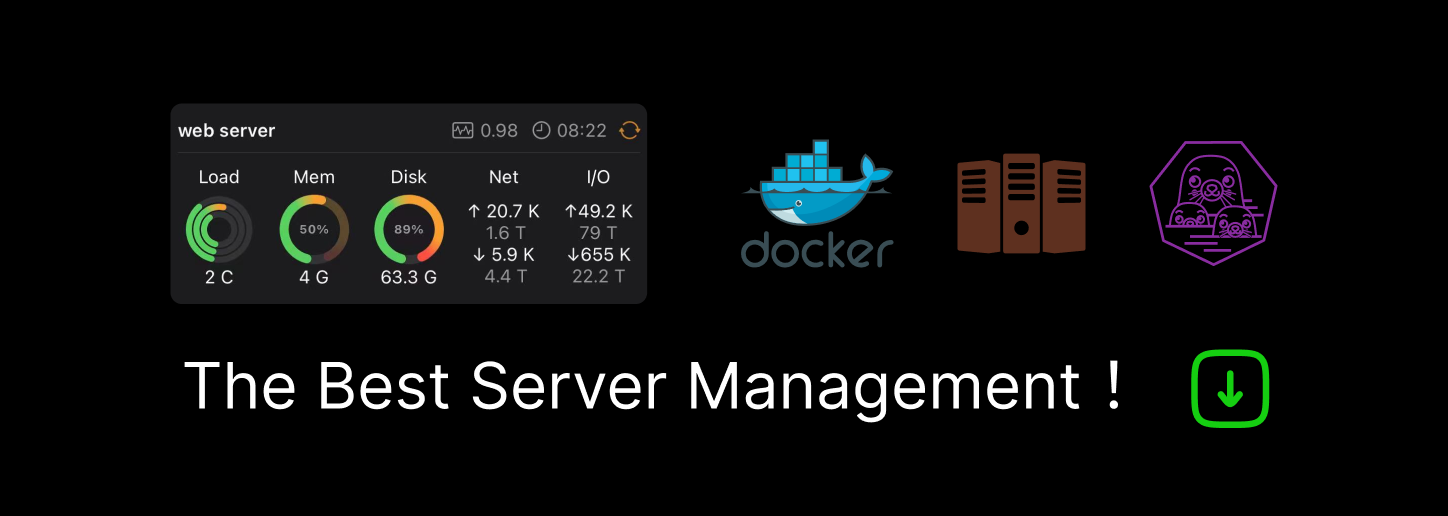
For the first time, The Data Science Salon will land in Austin, TX, an acclaimed technology hub, to discuss how we can apply AI and Machine Learning to the fields of Finance, Healthcare and Technology. We can’t wait to share our renowned lineup of speakers with you, so much so that we’ve reached out to them to relay some of their preliminary thoughts on this event’s major themes.
As we learn more and more about how to effectively manipulate our data for research, we are compelled to increasingly examine the quality of our data. Mark Boudria, VP Artificial Intelligence at HyperGiant, believes that “ this is one of the biggest issues facing people attempting to use data for machine intelligence.”
“For the last ten to 15 years, the big search engines and cloud providers have been preaching incessantly about big data. However, for all the effort put into big data, I have yet to see a true, meaningful dataset solve a problem for an enterprise,” Boudria says. “In fact, what we see most of the time, seems to tell me more and more that the data we require just doesn’t exist. What we actually have is human-readable information versus having machine-consumable data and this is a serious problem. Without raw access to a meaningful dataset, all the math in the world gets you nowhere. Namita Lokare, Sr. Biomedical Algorithm Engineer at LVL Technologies Inc., agrees with Boudria that the current quality of our collective data leaves much to be desired. “ Datasets often have problems such as missing values, high-cardinality inputs, outliers, correlated features, rare events and sparse data,” Lokare explains. “ Ignoring such data quality issues can lead to poor models with low prediction datasets.”
Despite these undeniable challenges data scientists face, researchers are already taking tangible steps to improve. “ Sampling appropriately or weighting classes during the training phase can help reduce bias in models. Variable selection helps aid the removal of redundant variables and allows for more interpretable models,” Lokare says. “Recently a lot of work has been done in the field of ‘Automated Feature Engineering’ to generate features in an unsupervised fashion from the raw data. This is great because non-experts can utilize this method without having any domain or machine learning knowledge.”
What is the Next Big Trend in Data Science?
With 2019 fresh off the griddle, data scientists and researchers alike are wondering what new innovations are likely to take over the field this year. Our Austin speakers and workshop leaders come from a diverse range of backgrounds and many gave us their own predictions of what to expect.
Andy Terrel, President of NumFocus:
“With the prominence of the GPU in deep learning and the new development of the data frame in C++ and CUDA, I can see libraries moving their high level logic to C or C++ from the usual R or Python. The newer Julia language also has a major opportunity to impact the development of new tools. It is one of the best abstractions for high level programming generating low level code and is already generating petaflop codes on GPUs and TPUs. With the data science tools catching up to accelerated hardware, perhaps 2019 is the year the rest of the data science stack moves to accelerators.”
Patrick McGarry, Head of Community at data.world:
“ Given the continued meteoric rise of data science as a central part of business and organizational decision making, 2019 is already shaping up to be a year of heavy education. We’re seeing an enormous need for data assets, analysis, and practitioners and that is starting to color our interactions with prospects, customers, and the broader data ecosystem. Obviously, our hope is to continue to enable the open data community through our platform, our efforts around datapractices.org , and whitepapers or other educational materials, but it is also heavily impacting how we think about physical events.
The days of “fire-and-forget” sponsorships, or even passive consumption of presented materials are quickly fading. Consumers of technology have ever evolving sophistication in how they think about their own approach to technology stacks and daily processes. As such, we are challenging ourselves to try to build as much of an interactive approach as possible when it comes to industry events, webinars, and other public-facing events.
While major events like WebSummit, CES, and others will continue for years to come, I think you’ll quickly start to see the appetite for small targeted events continue to rise. You only have to look as far as Columbia’s Data Innovation Network, Formulated.By’s Data Science Salon, or Collibra’s Data Citizens events for intimate data-focused events. Even major events like Tableau Conference, Strata, and ISWC continue to increase their round tables, workshops, panels, and interactive programming to give consumers the ability to go hands on to get more personalized engagement.”
Lex Roman, Senior Product Designer at The Black Tux:
“The next big trend in data strategy will be privacy: We’re not having enough conversations about how much information we really need. I’m particularly interested in how we will evolve our thinking on location tracking. Currently, we are often over-collecting information and under-considering what value it really has. When data is used or managed poorly, it can endanger people’s safety. For example, exposing the location or habits of someone who has escaped a domestic violence situation. I’d like to see technologists driving this conversation more publicly so it’s not left to legislators to decide.
As for data science and machine learning specifically, the next trend will be self-learning experiments. Many teams have outgrown simple AB testing as the dominant tactic for learning. Teams that can design adaptive systems will learn and improve faster. Multivariate tests that predict and create variations. Responding to user behavior dynamically rather then predefining every step and expected result. Reframing your entire platform as a learning tool rather than static software to be evolved. The key is focusing on the outcomes and designing your system around those.”
Sridharan Kamalakannan, Principle Data Scientist at Humana:
“Interpretability of predictive models is increasingly becoming a key component for these models to be widely accepted and used in a healthcare setting. At a patient level, these highly complex machine learning models often act as a black-box for the clinicians and care managers. Many techniques like partial dependence plots, individual, conditional expectations and surrogate models like LIME have emerged in the recent past to address the interpretability aspect of these models. Moving forward this year, one can expect a lot of research work to surface, involving automating and improving these model-agnostic techniques that provide explanations for these complex models.”
Gerald Fahner, Principle Data Scientist at FICO:
“Machine learning models are currently popular in finance and healthcare because of their effectiveness at learning complex nonlinear associations from large datasets, resulting in strong predictive performance. Yet for critical applications such as credit risk management or medical decision support, predictions are seldom considering satisfying solutions on their own. Such applications also demand transparent and intuitive explanations of how the models arrive at their predictions. Not only may this be legally required, but sound explanations can indeed pave the way for advanced reasoning and for planning optimal interventions.
Reasoning about interventions is very different from learning associations and goes materially beyond data mining and statistical inference. Related developments occur in the newer research areas of explainable AI and causal analysis. Both from our applied work and innovations in credit risk and healthcare, as well as from tracking wider academic progress, I conclude that analytics dedicated to explanation and intervention will achieve greater prominence over the coming years, as well as benefit an increasing number of valuable applications in finance and healthcare.”
Where Can We Get More of Everything Data Science?
One of the most popular questions we and our speakers receive involves where our audience members can go to immerse themselves further into the world of data science. We knew that Laura Noren, Director of Research at Obsidian and acclaimed expert on ethics in data science, would have some noteworthy answers. We’ve listed her recommendations below:
Laura Noren’s Top Data Science Events to Attend:
NeurIPS
Vancouver, Canada | December 2019
“Formerly NIPS, but now known as NeurIPS to protect the audience from bad jokes and predictably unfortunate content using the same hashtag on twitter, NeurIPS is one of the premiere data science and AI conferences in the world. Go there to hear the latest from industry, from academia, to find a job, to learn new methods at a workshop, to find a project partner, or simply just to be with people who appreciate the true complexity of the field. NeurIPS stands for Neural Information Processing Systems, so you’ll hear a lot about neural networks. This conference has attracted some hype, but is still a methodologically deep, true practitioner event. They bumped up their focus on bias in AI and other ethical considerations a couple years ago, which was a welcome improvement.”
ICML and/or ICLR
ICML | Long Beach, CA | June 10–15, 2019
ICLR | New Orleans, LA | May 6–9, 2019
“ICML and ICLR are in the same family as NeurIPS. They’re cousin conferences focusing on slightly different aspects of machine learning methodology, though there is a fair amount of overlap between them. ICML stands for the International Conference on Machine Learning and is a more of a general purpose conference. ICLR stands for International Conference on Learning Representations and focuses a little more on image recognition in addition to “computational biology, speech recognition, text understanding, gaming, and robotics”. Just like NeurIPS, the conference features research papers and posters; workshops; recruiting; and a convivial atmosphere. These two conferences are slightly less global than NeurIPS, but they are both non-fluffy events seriously focused on data science methods.”
Open Data Science Conference
Boston, MA | April 30-May 3, 2019
“These are conferences for anyone using or developing open source software. Want to learn more about the open source data science tools that you’re already using? These conferences tend to feature workshops by core developers. They have also been paying attention to research ethics and data privacy issues, which is missing from some of the more market-oriented conferences. These conferences are great for people who want to pick up new skills. They host one annually on the west coast, one on the east coast, and one in Europe.”
Women in Data Science
Stanford University | March 4, 2019
“Women in Data Science is headquartered at Stanford University and takes place in early March. It also has companion events all over the world. It’s one of the most interesting conference formats I’ve ever encountered. One hub location puts on an incredibly high-end day of top researchers and industry leaders in data science and artificial intelligence. The other locations can either stream the content, put together their own panels and speakers with local talent, or do a mix of both. All the speakers are women, but men are more than welcome to attend as well. Because each location draws on the local area, it’s a great place to network with data scientists working near you.”
Local meetups
“ Last but not least, get out there and go to local meetups with other data scientists. I encourage everyone I mentor to attend and present at meetups to build their networks, stay fresh in their skills, and learn how to explain what they know to peers.”
Like the Preview?
Make sure to catch the whole show with our full speaker lineup at the Data Science Salon Austin on Thursday, February 21 and Friday, February 22, 2019. Tickets are almost sold out, so pick yours up today!
Recommend
About Joyk
Aggregate valuable and interesting links.
Joyk means Joy of geeK